热点文献带您关注存算一体技术的最新进展——图书馆前沿文献专题推荐服务(73)
2022-11-18
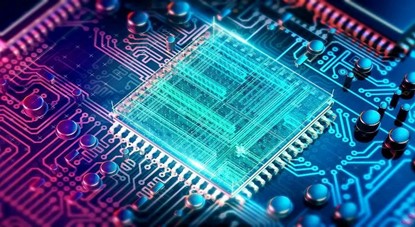
在上一期热点文献推荐中,我们为您推荐了电子皮肤的最新发展前沿,包括利用纤维Bragg光栅和深层神经网络的大面积触觉敏感皮肤,可用于无线健康监测设备的基于表面声波传感器的无芯片无线电子皮肤,基于印刷突触晶体管的可感受“疼痛”的电子皮肤,一种由水凝胶和硅胶弹性体组成的具有触觉感应能力的仿生弹性机器人皮肤。
本期我们为您选取了4篇文献,介绍存算一体技术的最新发展前沿,包括三星电子发表的全球首个基于MRAM交叉阵列的存内计算研究,基于阻变存储器RRAM的存算一体芯片,基于三维阻变存储器的存内计算宏芯片,基于集成铁电突触阵列的CMOS兼容存内计算加速器,推送给相关领域的科研人员。
文献一 用于图像分类和人脸检测的基于MRAM交叉阵列的存内计算
A crossbar array of magnetoresistive memory devices for in-memory computing
Jung, Seungchul, etc.
NATURE, 2022, 601(7892): 211–216
Implementations of artificial neural networks that borrow analogue techniques could potentially offer low-power alternatives to fully digital approaches(1-3). One notable example is in-memory computing based on crossbar arrays of non-volatile memories(4-7) that execute, in an analogue manner, multiply-accumulate operations prevalent in artificial neural networks. Various non-volatile memories-including resistive memory(8-13), phase-change memory(14,15) and flash memory(16-19)-have been used for such approaches. However, it remains challenging to develop a crossbar array of spin-transfer-torque magnetoresistive random-access memory (MRAM)(20-22), despite the technology's practical advantages such as endurance and large-scale commercialization(5). The difficulty stems from the low resistance of MRAM, which would result in large power consumption in a conventional crossbar array that uses current summation for analogue multiply-accumulate operations. Here we report a 64 x 64 crossbar array based on MRAM cells that overcomes the low-resistance issue with an architecture that uses resistance summation for analogue multiply-accumulate operations. The array is integrated with readout electronics in 28-nanometre complementary metal-oxide-semiconductor technology. Using this array, a two-layer perceptron is implemented to classify 10,000 Modified National Institute of Standards and Technology digits with an accuracy of 93.23 per cent (software baseline: 95.24 per cent). In an emulation of a deeper, eight-layer Visual Geometry Group-8 neural network with measured errors, the classification accuracy improves to 98.86 per cent (software baseline: 99.28 per cent). We also use the array to implement a single layer in a ten-layer neural network to realize face detection with an accuracy of 93.4 per cent.
阅读原文:https://www.nature.com/articles/s41586-021-04196-6
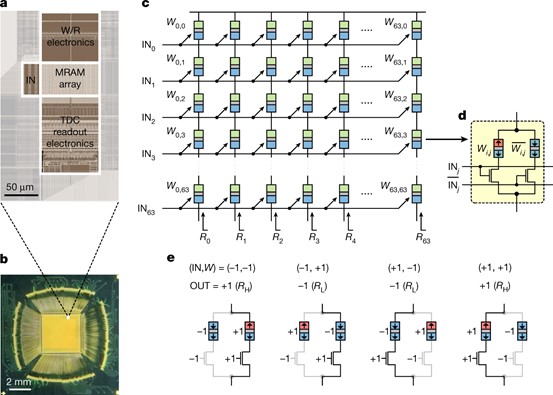
MRAM crossbar array
文献二 基于RRAM的存内计算芯片
A compute-in-memory chip based on resistive random-access memory
Wan, Weier, etc.
NATURE, 2022,608(7923): 504-512
Realizing increasingly complex artificial intelligence (AI) functionalities directly on edge devices calls for unprecedented energy efficiency of edge hardware. Compute-in-memory (CIM) based on resistive random-access memory (RRAM)(1) promises to meet such demand by storing AI model weights in dense, analogue and non-volatile RRAM devices, and by performing AI computation directly within RRAM, thus eliminating power-hungry data movement between separate compute and memory(2-5). Although recent studies have demonstrated in-memory matrix-vector multiplication on fully integrated RRAM-CIM hardware(6-17), it remains a goal for a RRAM-CIM chip to simultaneously deliver high energy efficiency, versatility to support diverse models and software-comparable accuracy. Although efficiency, versatility and accuracy are all indispensable for broad adoption of the technology, the inter-related trade-offs among them cannot be addressed by isolated improvements on any single abstraction level of the design. Here, by co-optimizing across all hierarchies of the design from algorithms and architecture to circuits and devices, we present NeuRRAM-a RRAM-based CIM chip that simultaneously delivers versatility in reconfiguring CIM cores for diverse model architectures, energy efficiency that is two-times better than previous state-of-the-art RRAM-CIM chips across various computational bit-precisions, and inference accuracy comparable to software models quantized to four-bit weights across various AI tasks, including accuracy of 99.0 percent on MNIST18 and 85.7 percent on CIFAR-10(19) image classification, 84.7-percent accuracy on Google speech command recognition(20), and a 70-percent reduction in image-reconstruction error on a Bayesian image-recovery task.
阅读原文:https://www.nature.com/articles/s41586-022-04992-8
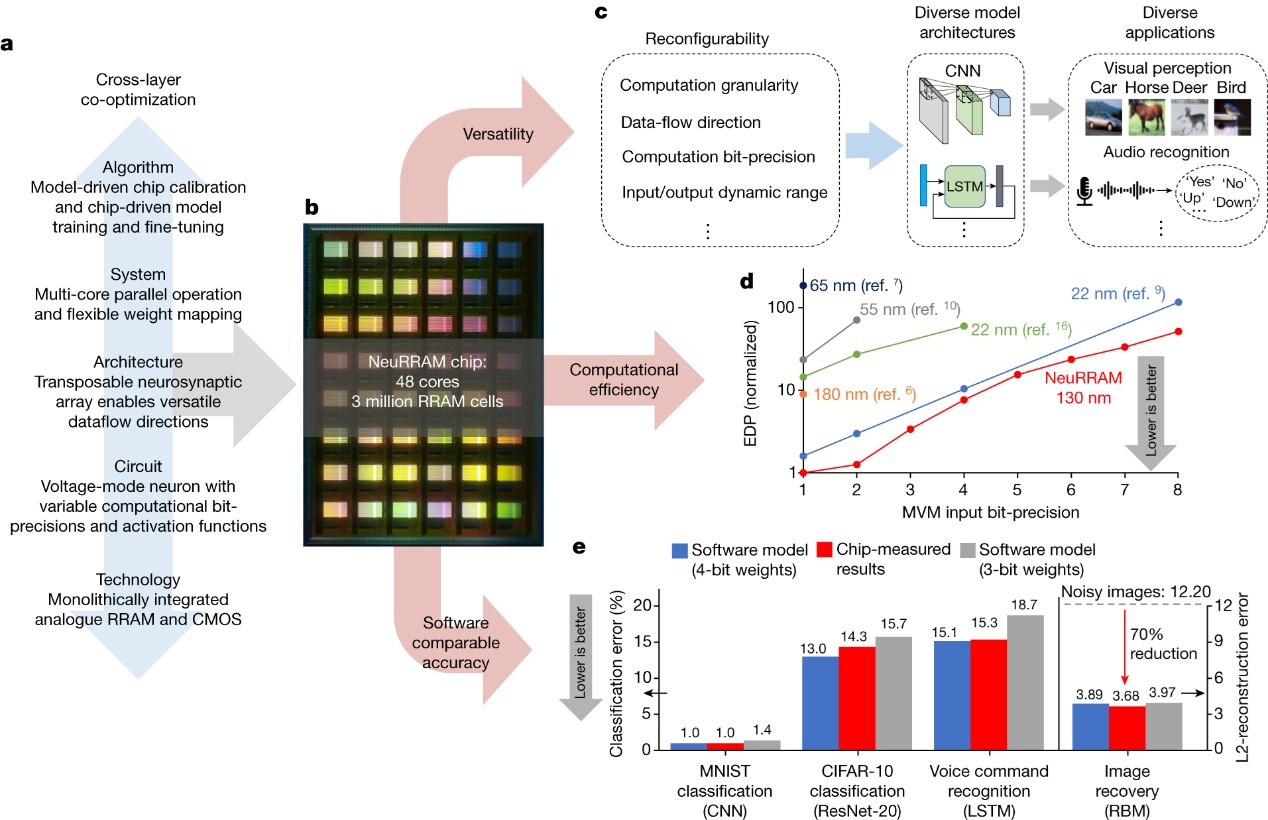
Design methodology and main contributions of the NeuRRAM chip
文献三 基于三维阻变存储器的存内计算宏芯片
A computing-in-memory macro based on three-dimensional resistive random-access memory
Huo, Qiang, etc.
NATURE ELECTRONICS, 2022, 5(7): 469-477
Non-volatile computing-in-memory macros that are based on two-dimensional arrays of memristors are of use in the development of artificial intelligence edge devices. Scaling such systems to three-dimensional arrays could provide higher parallelism, capacity and density for the necessary vector-matrix multiplication operations. However, scaling to three dimensions is challenging due to manufacturing and device variability issues. Here we report a two-kilobit non-volatile computing-in-memory macro that is based on a three-dimensional vertical resistive random-access memory fabricated using a 55 nm complementary metal-oxide-semiconductor process. Our macro can perform 3D vector-matrix multiplication operations with an energy efficiency of 8.32 tera-operations per second per watt when the input, weight and output data are 8, 9 and 22 bits, respectively, and the bit density is 58.2 bit mu m(-2). We show that the macro offers more accurate brain MRI edge detection and improved inference accuracy on the CIFAR-10 dataset than conventional methods.
阅读原文:https://www.nature.com/articles/s41928-022-00795-x
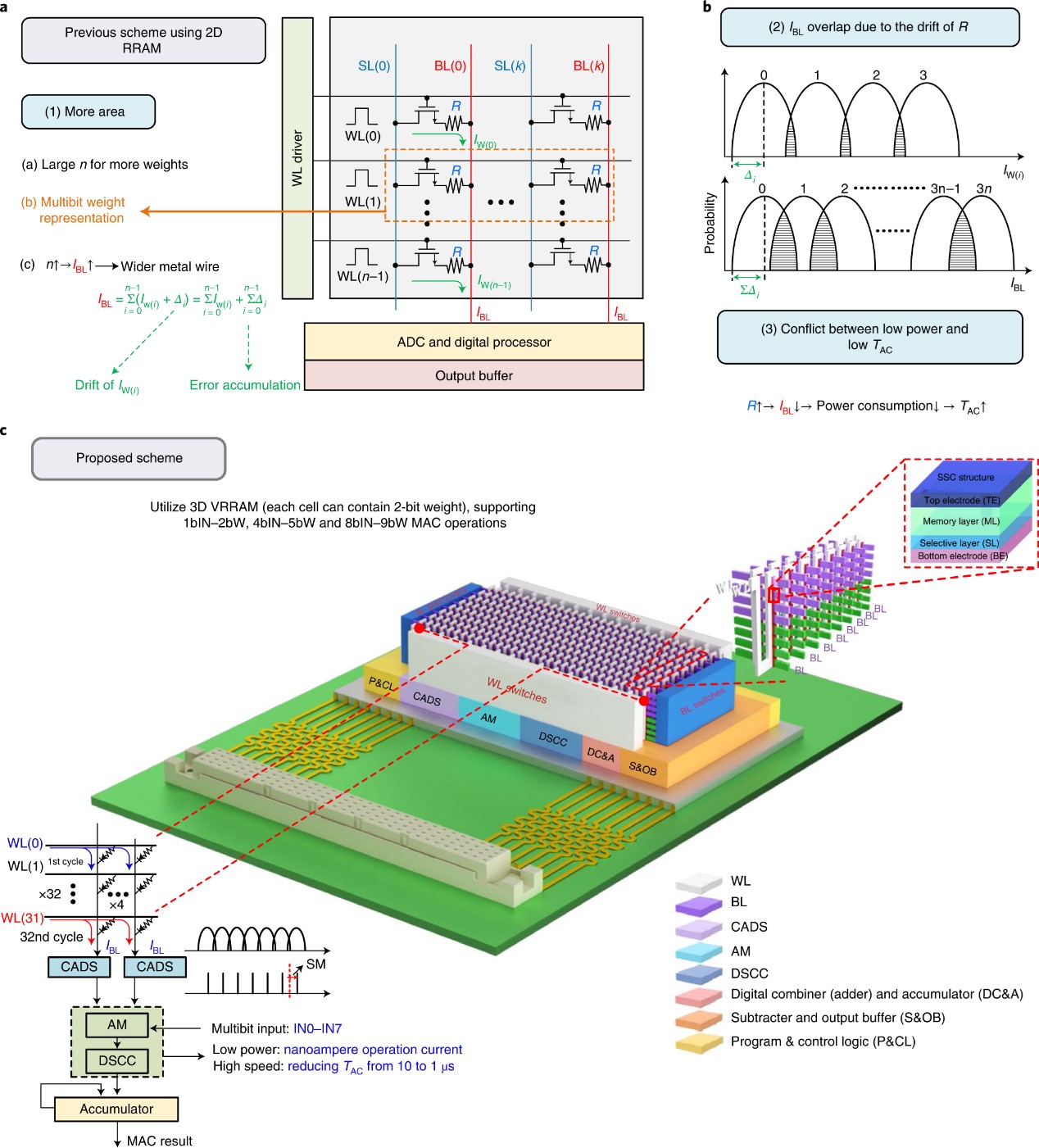
Challenges of applying 2D RRAM to large 3D CNNs and proposed 3D nvCIM scheme
文献四 基于集成铁电突触阵列的CMOS兼容存内计算加速器
CMOS-compatible compute-in-memory accelerators based on integrated ferroelectric synaptic arrays for convolution neural networks
Kim, Min-Kyu, etc.
SCIENCE ADVANCES, 2022, 8(14)
Convolutional neural networks (CNNs) have gained much attention because they can provide superior complex image recognition through convolution operations. Convolution processes require repeated multiplication and accumulation operations, which are difficult tasks for conventional computing systems. Compute-in-memory (CIM) that uses parallel data processing is an ideal device structure for convolution operations. CIM based on two-terminal synaptic devices with a crossbar structure has been developed, but unwanted leakage current paths and the high-power consumption remain as the challenges. Here, we demonstrate integrated ferroelectric thin-film transistor (FeTFT) synaptic arrays that can provide efficient parallel programming and data processing for CNNs by the selective and accurate control of polarization in the ferroelectric layer. In addition, three-terminal FeTFTs can act as both nonvolatile memory and access device, which tackle issues from two-terminal devices. An integrated FeTFT synaptic array with parallel programming capabilities can perform convolution operations to extract image features with a high-recognition accuracy.
阅读原文:https://www.science.org/doi/10.1126/sciadv.abm8537
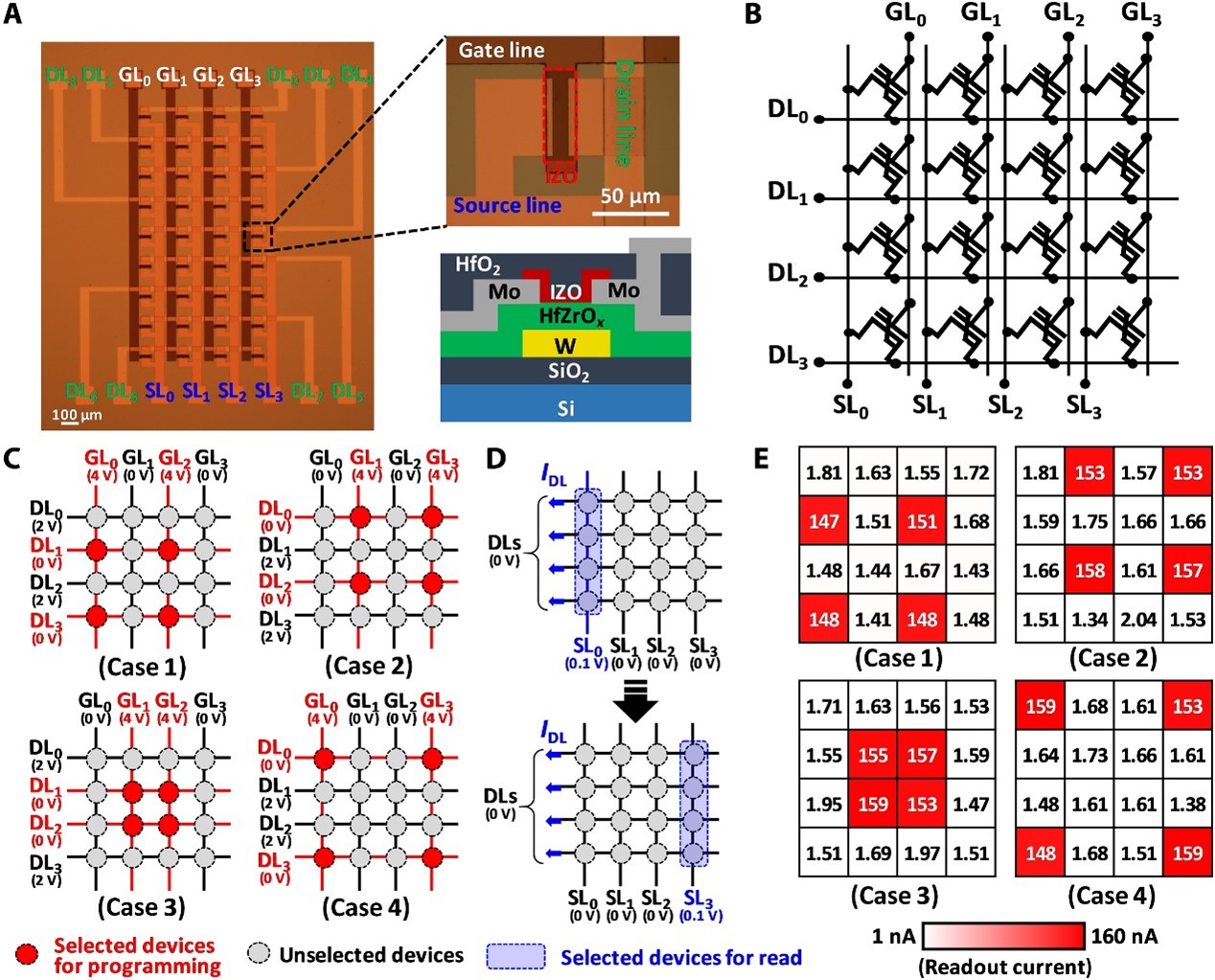
Ferroelectric synaptic transistor array and parallel programming operation
往期精彩推荐
热点论文解读AI应用领域 ——图书馆前沿文献专题推荐服务(3)
热点论文带您探究5G和未来通信——图书馆前沿文献专题推荐服务 (4)
前沿文献带您解读自然语言处理技术 ——图书馆前沿文献专题推荐服务(5)
热点论文带您探究5G和未来通信材料技术领域 ——图书馆前沿文献专题推荐服务(6)
热点文献带您关注AI情感分类技术 ——图书馆前沿文献专题推荐服务(7)
热点论文带您探究6G的无限可能——图书馆前沿文献专题推荐服务(8)
热点文献带您关注AI文本摘要自动生成 ——图书馆前沿文献专题推荐服务(9)
热点论文:5G/6G引领社会新进步——图书馆前沿文献专题推荐服务(10)
热点文献带您关注AI机器翻译 ——图书馆前沿文献专题推荐服务(11)
热点论文与您探讨5G/6G网络技术新进展——图书馆前沿文献专题推荐服务(12)
热点文献带您关注AI计算机视觉 ——图书馆前沿文献专题推荐服务(13)
热点论文与带您领略5G/6G的硬科技与新思路 ——图书馆前沿文献专题推荐服务(14)
热点文献带您关注AI计算机视觉 ——图书馆前沿文献专题推荐服务(15)
热点论文带您领略5G/6G的最新技术动向 ——图书馆前沿文献专题推荐服务(18)
热点文献带您关注图神经网络——图书馆前沿文献专题推荐服务(19)
热点论文与带您领略5G/6G材料技术的最新发展——图书馆前沿文献专题推荐服务(20)
热点文献带您关注模式识别——图书馆前沿文献专题推荐服务(21)
热点论文与带您领略6G网络技术的最新发展趋势 ——图书馆前沿文献专题推荐服务(22)
热点文献带您关注机器学习与量子物理 ——图书馆前沿文献专题推荐服务(23)
热点论文与带您领略5G/6G通信器件材料的最新进展 ——图书馆前沿文献专题推荐服务(24)
热点文献带您关注AI自动驾驶——图书馆前沿文献专题推荐服务(25)
热点论文与带您领略5G/6G网络安全和技术的最新进展——图书馆前沿文献专题推荐服务(26)
热点文献带您关注AI神经网络与忆阻器——图书馆前沿文献专题推荐服务(27)
热点论文与带您领略5G/6G电子器件和太赫兹方面的最新进展——图书馆前沿文献专题推荐服务(28)
热点文献带您关注AI与机器人——图书馆前沿文献专题推荐服务(29)
热点论文与带您领略5G/6G热点技术的最新进展——图书馆前沿文献专题推荐服务(30)
热点文献带您关注AI与触觉传感技术——图书馆前沿文献专题推荐服务(31)
热点论文与带您领略5G/6G热点技术的最新进展——图书馆前沿文献专题推荐服务(32)
热点文献带您关注AI深度学习与计算机视觉——图书馆前沿文献专题推荐服务(33)
热点论文与带您领略未来通信的热点技术及最新进展——图书馆前沿文献专题推荐服务(34)
热点文献带您关注AI强化学习——图书馆前沿文献专题推荐服务(35)
热点论文与带您领略5G/6G基础研究的最新进展——图书馆前沿文献专题推荐服务(36)
热点文献带您关注AI与边缘计算——图书馆前沿文献专题推荐服务(37)
热点论文与带您领略5G/6G领域热点研究的最新进展——图书馆前沿文献专题推荐服务(38)
热点文献带您关注AI技术的最新进展——图书馆前沿文献专题推荐服务(39)
热点论文与带您领略5G相关领域研究的最新进展——图书馆前沿文献专题推荐服务(40)
热点文献带您关注AI视觉跟踪——图书馆前沿文献专题推荐服务(41)
热点论文带您领略未来通信在海空领域研究的最新进展——图书馆前沿文献专题推荐服务(42)
热点文献带您关注AI与医学研究——图书馆前沿文献专题推荐服务(43)
热点论文带您领略未来通信在材料领域研究的最新进展——图书馆前沿文献专题推荐服务(44)
热点文献带您关注AI与医学图像——图书馆前沿文献专题推荐服务(45)
热点论文带您领略未来通信在光电材料及信息编码领域的最新进展——图书馆前沿文献专题推荐服务(46)
热点文献带您关注AI与生物学——图书馆前沿文献专题推荐服务(47)
热点论文带您领略未来通信在新材料技术领域的最新进展——图书馆前沿文献专题推荐服务(48)
热点文献带您关注AI与人脸识别——图书馆前沿文献专题推荐服务(49)
热点论文带您领略光电半导体领域的最新进展——图书馆前沿文献专题推荐服务(50)
热点文献带您关注AI在集成电路领域的最新进展——图书馆前沿文献专题推荐服务(51)
热点论文带您领略半导体领域的最新进展——图书馆前沿文献专题推荐服务(52)
热点文献带您关注AI在光神经网络领域的最新进展——图书馆前沿文献专题推荐服务(53)
热点论文带您领略未来通信在新材料技术领域的最新进展——图书馆前沿文献专题推荐服务(54)
热点文献带您关注AI深度神经网络的最新进展——图书馆前沿文献专题推荐服务(55)
热点论文带您领略新材料半导体领域的最新进展——图书馆前沿文献专题推荐服务(56)
热点文献带您关注AI视频动作识别的最新进展——图书馆前沿文献专题推荐服务(57)
热点论文带您领略未来通信热点技术的最新进展——图书馆前沿文献专题推荐服务(58)
热点文献带您关注AI深度学习的最新进展——图书馆前沿文献专题推荐服务(59)
热点论文带您领略未来通信研究热点的最新进展——图书馆前沿文献专题推荐服务(60)
热点文献带您关注AI领域的最新进展——图书馆前沿文献专题推荐服务(61)
热点论文带您探索智能化以及超表面在未来通信中的应用——图书馆前沿文献专题推荐服务(62)
热点文献带您关注AI领域的最新进展——图书馆前沿文献专题推荐服务(63)
热点论文带您领略半导体领域的最新进展——图书馆前沿文献专题推荐服务(64)
热点文献带您关注AI领域的最新进展——图书馆前沿文献专题推荐服务(65)
热点论文带您探索新型网络架构以及超表面在未来通信中的应用——图书馆前沿文献专题推荐服务(66)
热点文献带您关注AI深度强化学习的最新进展——图书馆前沿文献专题推荐服务(67)
热点论文带您探索新型网络架构以及超材料在未来通信中的应用——图书馆前沿文献专题推荐服务(68)
热点文献带您关注AI图神经网络的最新进展——图书馆前沿文献专题推荐服务(69)
热点论文带您探索未来网络中使用的新材料以及相关技术 ——图书馆前沿文献专题推荐服务(70)
热点文献带您关注AI的最新进展——图书馆前沿文献专题推荐服务(71)