热点文献带您关注AI领域的最新进展——图书馆前沿文献专题推荐服务(63)
2022-04-19
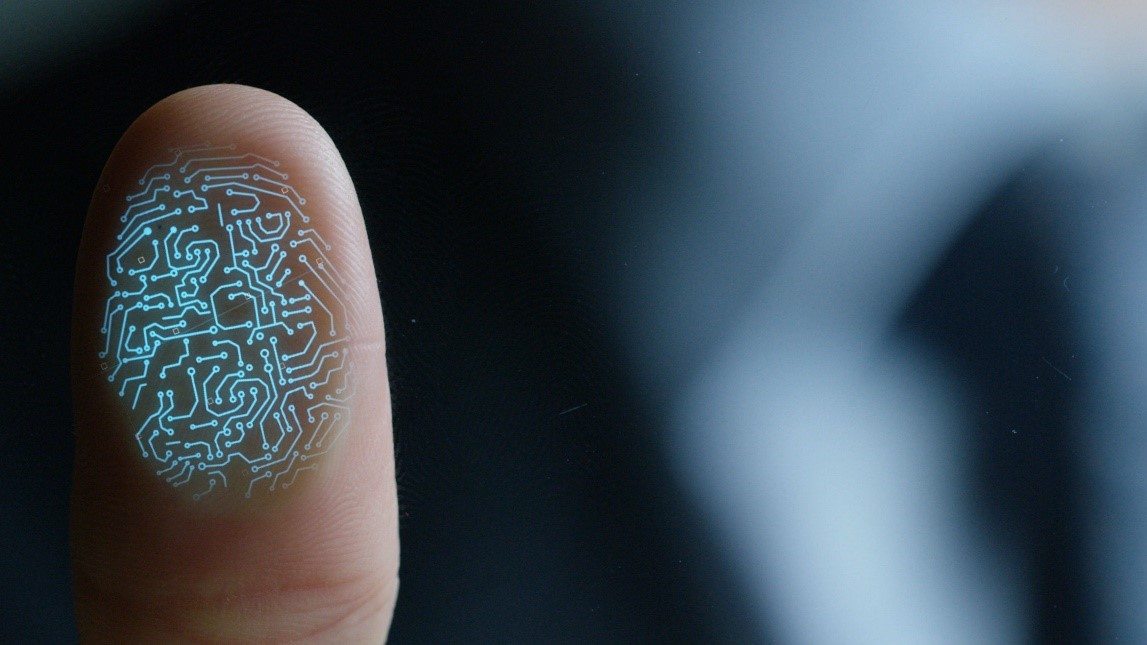
在上一期AI文献推荐中,我们为您推荐了人工智能在其他学科中应用的热点文献,包括基于深度神经网络的古籍恢复,利用人工智能机器学习指导数学直觉,气泡铸造软体机器人,以及人工智能与量子力学方法在化学中的应用。
本期我们为您选取了4篇文献,介绍人工智能在生物特征识别方向的热点文献。近日,斯坦福大学发布的《人工智能指数2022》(Artificial Intelligence Index Report 2022)报告用整页篇幅重点介绍了我校人工智能学院邓伟洪教授课题组在蒙面人脸识别中的研究成果。课题组发布了6000张蒙面人脸的人脸识别数据集,以应对大规模戴口罩带来的新识别挑战。通过相似外貌、跨年龄、跨姿态、口罩遮挡、对抗攻击等无约束条件下的人脸图片组,构建了五个规模相同的数据集(SLLFW/CALFW/CPLFW/MLFW/TALFW),从五个维度综合评价人脸识别鲁棒性和安全性,已被帝国理工、腾讯、百度等发表的数百篇论文实验使用。报告引用的测试结果表明,课题组发表的SFace深度学习方法“SFace: Sigmoid-Constrained Hypersphere Loss for Robust Face Recognition”取得了最佳的综合性能。目前OpenCV已经收录了SFace的轻量级版本,成为其默认的人脸识别算法模型。此外,还为您推荐基于未经训练深度神经网络的人脸检测,基于复数神经网络的虹膜识别,以及可处理缺失输入模式的多模式步态识别三篇生物特征识别领域的热点文献。
文献一 基于SFace深度学习的人脸识别
SFace: Sigmoid-Constrained Hypersphere Loss for Robust Face Recognition
Zhong, Yaoyao, etc.
IEEE TRANSACTIONS ON IMAGE PROCESSING, 2021, 30: 2587-2598
Deep face recognition has achieved great success due to large-scale training databases and rapidly developing loss functions. The existing algorithms devote to realizing an ideal idea: minimizing the intra-class distance and maximizing the inter-class distance. However, they may neglect that there are also low quality training images which should not be optimized in this strict way. Considering the imperfection of training databases, we propose that intra-class and inter-class objectives can be optimized in a moderate way to mitigate overfitting problem, and further propose a novel loss function, named sigmoid-constrained hypersphere loss (SFace). Specifically, SFace imposes intra-class and inter-class constraints on a hypersphere manifold, which are controlled by two sigmoid gradient re-scale functions respectively. The sigmoid curves precisely re-scale the intra-class and inter-class gradients so that training samples can be optimized to some degree. Therefore, SFace can make a better balance between decreasing the intra-class distances for clean examples and preventing overfitting to the label noise, and contributes more robust deep face recognition models. Extensive experiments of models trained on CASIA-WebFace, VGGFace2, and MS-Celeb-1M databases, and evaluated on several face recognition benchmarks, such as LFW, MegaFace and IJB-C databases, have demonstrated the superiority of SFace.
阅读原文:https://ieeexplore.ieee.org/document/9318547
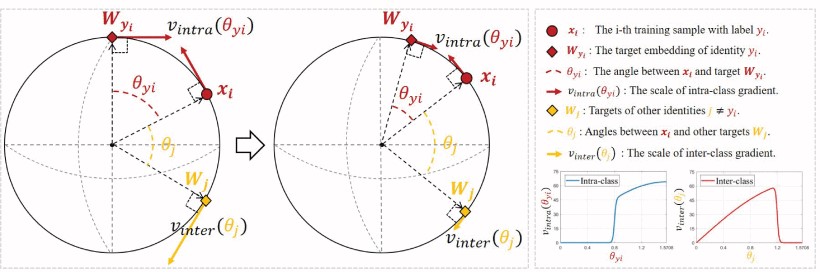
Schematic illustration of the sigmoid-constrained hypersphere loss (SFace)
文献二 基于未经训练深度神经网络的人脸检测
Face detection in untrained deep neural networks
Baek, Seungdae, etc.
NATURE COMMUNICATIONS, 2021, 12(1): 7328
Face-selective neurons are observed in the primate visual pathway and are considered as the basis of face detection in the brain. Here, using a hierarchical deep neural network model of the ventral visual stream, the authors suggest that face selectivity arises in the complete absence of training.
Face-selective neurons are observed in the primate visual pathway and are considered as the basis of face detection in the brain. However, it has been debated as to whether this neuronal selectivity can arise innately or whether it requires training from visual experience. Here, using a hierarchical deep neural network model of the ventral visual stream, we suggest a mechanism in which face-selectivity arises in the complete absence of training. We found that units selective to faces emerge robustly in randomly initialized networks and that these units reproduce many characteristics observed in monkeys. This innate selectivity also enables the untrained network to perform face-detection tasks. Intriguingly, we observed that units selective to various non-face objects can also arise innately in untrained networks. Our results imply that the random feedforward connections in early, untrained deep neural networks may be sufficient for initializing primitive visual selectivity.
阅读原文:https://www.nature.com/articles/s41467-021-27606-9
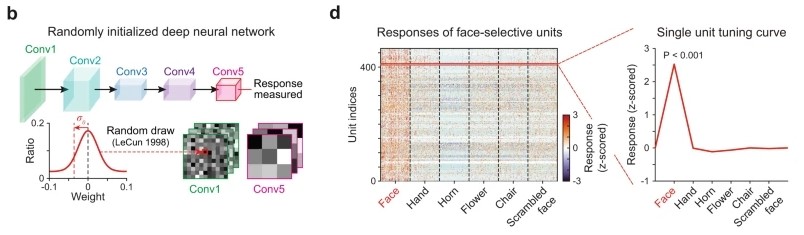
Spontaneous emergence of face-selectivity in untrained networks
文献三 基于复数神经网络的虹膜识别
Complex-valued Iris Recognition Network
Trevor J. Jones, etc.
IEEE TRANSACTIONS ON PATTERN ANALYSIS AND MACHINE INTELLIGENCE, 2022
In this work, we design a fully complex-valued neural network for the task of iris recognition. Unlike the problem of general object recognition, where real-valued neural networks can be used to extract pertinent features, iris recognition depends on the extraction of both phase and amplitude information from the input iris texture in order to better represent its stochastic content. This necessitates the extraction and processing of phase information that cannot be effectively handled by a real-valued neural network. In this regard, we design a fully complex-valued neural network that can better capture the multi-scale, multi-resolution, and multi-orientation phase and amplitude features of the iris texture. We show a strong correspondence of the proposed complex-valued iris recognition network with Gabor wavelets that are used to generate the classical IrisCode; however, the proposed method enables a new capability of automatic complex-valued feature learning that is tailored for iris recognition. We conduct experiments on three benchmark datasets - ND-CrossSensor-2013, CASIA-Iris-Thousand and UBIRIS.v2 - and show the benefit of the proposed network for the task of iris recognition. We exploit visualization schemes to convey how the complex-valued network, when in comparison to standard real-valued networks, extract fundamentally different features from the iris texture.
阅读原文:https://ieeexplore.ieee.org/document/9721052
文献四 可处理缺失输入模式的多模式步态识别
UGaitNet: Multimodal Gait Recognition With Missing Input Modalities
Marin-Jimenez, Manuel J., etc.
IEEE TRANSACTIONS ON INFORMATION FORENSICS AND SECURITY, 2021, 16: 5452-5462
Gait recognition systems typically rely solely on silhouettes for extracting gait signatures. Nevertheless, these approaches struggle with changes in body shape and dynamic backgrounds; a problem that can be alleviated by learning from multiple modalities. However, in many real-life systems some modalities can be missing, and therefore most existing multimodal frameworks fail to cope with missing modalities. To tackle this problem, in this work, we propose UGaitNet, a unifying framework for gait recognition, robust to missing modalities. UGaitNet handles and mingles various types and combinations of input modalities, i.e. pixel gray value, optical flow, depth maps, and silhouettes, while being camera agnostic. We evaluate UGaitNet on two public datasets for gait recognition: CASIA-B and TUM-GAID, and show that it obtains compact and state-of-the-art gait descriptors when leveraging multiple or missing modalities. Finally, we show that UGaitNet with optical flow and grayscale inputs achieves almost perfect (98.9%) recognition accuracy on CASIA-B (same-view "normal") and 100% on TUM-GAID ("ellapsed time"). Code will be available at https://github.com/avagait/ugaitnet
阅读原文:https://ieeexplore.ieee.org/document/9634027/
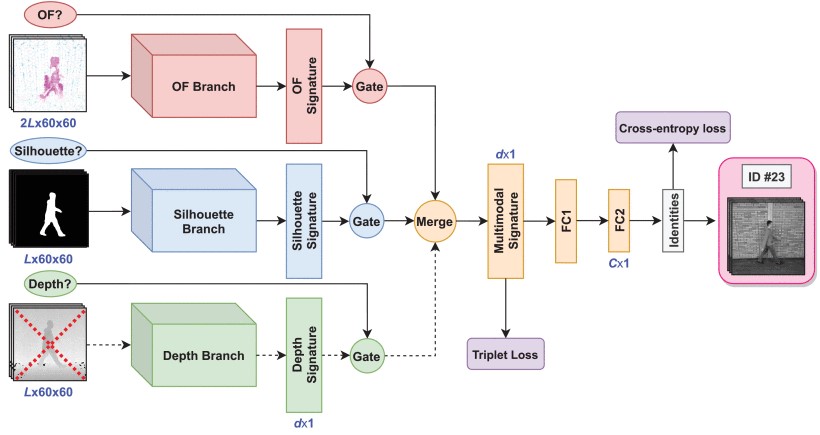
UGaitNet: multimodal gait recognition network robust to missing modalities
往期精彩推荐
前沿论文带您解读5G应用领域 ——图书馆前沿文献专题推荐服务(2)
热点论文解读AI应用领域 ——图书馆前沿文献专题推荐服务(3)
热点论文带您探究5G和未来通信——图书馆前沿文献专题推荐服务 (4)
前沿文献带您解读自然语言处理技术 ——图书馆前沿文献专题推荐服务(5)
热点论文带您探究5G和未来通信材料技术领域 ——图书馆前沿文献专题推荐服务(6)
热点论文解读AI应用领域 ——图书馆前沿文献专题推荐服务(3)
热点论文带您探究5G和未来通信——图书馆前沿文献专题推荐服务 (4)
前沿文献带您解读自然语言处理技术 ——图书馆前沿文献专题推荐服务(5)
热点论文带您探究5G和未来通信材料技术领域 ——图书馆前沿文献专题推荐服务(6)
热点文献带您关注AI情感分类技术 ——图书馆前沿文献专题推荐服务(7)
热点论文带您探究6G的无限可能——图书馆前沿文献专题推荐服务(8)
热点文献带您关注AI文本摘要自动生成 ——图书馆前沿文献专题推荐服务(9)
热点论文:5G/6G引领社会新进步——图书馆前沿文献专题推荐服务(10)
热点文献带您关注AI机器翻译 ——图书馆前沿文献专题推荐服务(11)
热点论文与您探讨5G/6G网络技术新进展——图书馆前沿文献专题推荐服务(12)
热点文献带您关注AI计算机视觉 ——图书馆前沿文献专题推荐服务(13)
热点论文与带您领略5G/6G的硬科技与新思路 ——图书馆前沿文献专题推荐服务(14)
热点文献带您关注AI计算机视觉 ——图书馆前沿文献专题推荐服务(15)
热点论文带您领略5G/6G的最新技术动向 ——图书馆前沿文献专题推荐服务(18)
热点文献带您关注图神经网络——图书馆前沿文献专题推荐服务(19)
热点论文与带您领略5G/6G材料技术的最新发展——图书馆前沿文献专题推荐服务(20)
热点文献带您关注模式识别——图书馆前沿文献专题推荐服务(21)
热点论文与带您领略6G网络技术的最新发展趋势 ——图书馆前沿文献专题推荐服务(22)
热点文献带您关注机器学习与量子物理 ——图书馆前沿文献专题推荐服务(23)
热点论文与带您领略5G/6G通信器件材料的最新进展 ——图书馆前沿文献专题推荐服务(24)
热点文献带您关注AI自动驾驶——图书馆前沿文献专题推荐服务(25)
热点论文与带您领略5G/6G网络安全和技术的最新进展——图书馆前沿文献专题推荐服务(26)
热点文献带您关注AI神经网络与忆阻器——图书馆前沿文献专题推荐服务(27)
热点论文与带您领略5G/6G电子器件和太赫兹方面的最新进展——图书馆前沿文献专题推荐服务(28)
热点文献带您关注AI与机器人——图书馆前沿文献专题推荐服务(29)
热点论文与带您领略5G/6G热点技术的最新进展——图书馆前沿文献专题推荐服务(30)
热点文献带您关注AI与触觉传感技术——图书馆前沿文献专题推荐服务(31)
热点论文与带您领略5G/6G热点技术的最新进展——图书馆前沿文献专题推荐服务(32)
热点文献带您关注AI深度学习与计算机视觉——图书馆前沿文献专题推荐服务(33)
热点论文与带您领略未来通信的热点技术及最新进展——图书馆前沿文献专题推荐服务(34)
热点文献带您关注AI强化学习——图书馆前沿文献专题推荐服务(35)
热点论文与带您领略5G/6G基础研究的最新进展——图书馆前沿文献专题推荐服务(36)
热点文献带您关注AI与边缘计算——图书馆前沿文献专题推荐服务(37)
热点论文与带您领略5G/6G领域热点研究的最新进展——图书馆前沿文献专题推荐服务(38)
热点文献带您关注AI技术的最新进展——图书馆前沿文献专题推荐服务(39)
热点论文与带您领略5G相关领域研究的最新进展——图书馆前沿文献专题推荐服务(40)
热点文献带您关注AI视觉跟踪——图书馆前沿文献专题推荐服务(41)
热点论文带您领略未来通信在海空领域研究的最新进展——图书馆前沿文献专题推荐服务(42)
热点文献带您关注AI与医学研究——图书馆前沿文献专题推荐服务(43)
热点论文带您领略未来通信在材料领域研究的最新进展——图书馆前沿文献专题推荐服务(44)
热点文献带您关注AI与医学图像——图书馆前沿文献专题推荐服务(45)
热点论文带您领略未来通信在光电材料及信息编码领域的最新进展——图书馆前沿文献专题推荐服务(46)
热点文献带您关注AI与生物学——图书馆前沿文献专题推荐服务(47)
热点论文带您领略未来通信在新材料技术领域的最新进展——图书馆前沿文献专题推荐服务(48)
热点文献带您关注AI与人脸识别——图书馆前沿文献专题推荐服务(49)
热点论文带您领略光电半导体领域的最新进展——图书馆前沿文献专题推荐服务(50)
热点文献带您关注AI在集成电路领域的最新进展——图书馆前沿文献专题推荐服务(51)
热点论文带您领略半导体领域的最新进展——图书馆前沿文献专题推荐服务(52)
热点文献带您关注AI在光神经网络领域的最新进展——图书馆前沿文献专题推荐服务(53)
热点论文带您领略未来通信在新材料技术领域的最新进展——图书馆前沿文献专题推荐服务(54)
热点文献带您关注AI深度神经网络的最新进展——图书馆前沿文献专题推荐服务(55)
热点论文带您领略新材料半导体领域的最新进展——图书馆前沿文献专题推荐服务(56)
热点文献带您关注AI视频动作识别的最新进展——图书馆前沿文献专题推荐服务(57)
热点论文带您领略未来通信热点技术的最新进展——图书馆前沿文献专题推荐服务(58)
热点文献带您关注AI深度学习的最新进展——图书馆前沿文献专题推荐服务(59)
热点论文带您领略未来通信研究热点的最新进展——图书馆前沿文献专题推荐服务(60)
热点文献带您关注AI领域的最新进展——图书馆前沿文献专题推荐服务(61)
热点论文带您探索智能化以及超表面在未来通信中的应用——图书馆前沿文献专题推荐服务(62)