热点文献带您关注AI深度学习与计算机视觉——图书馆前沿文献专题推荐服务(33)
2020-12-31
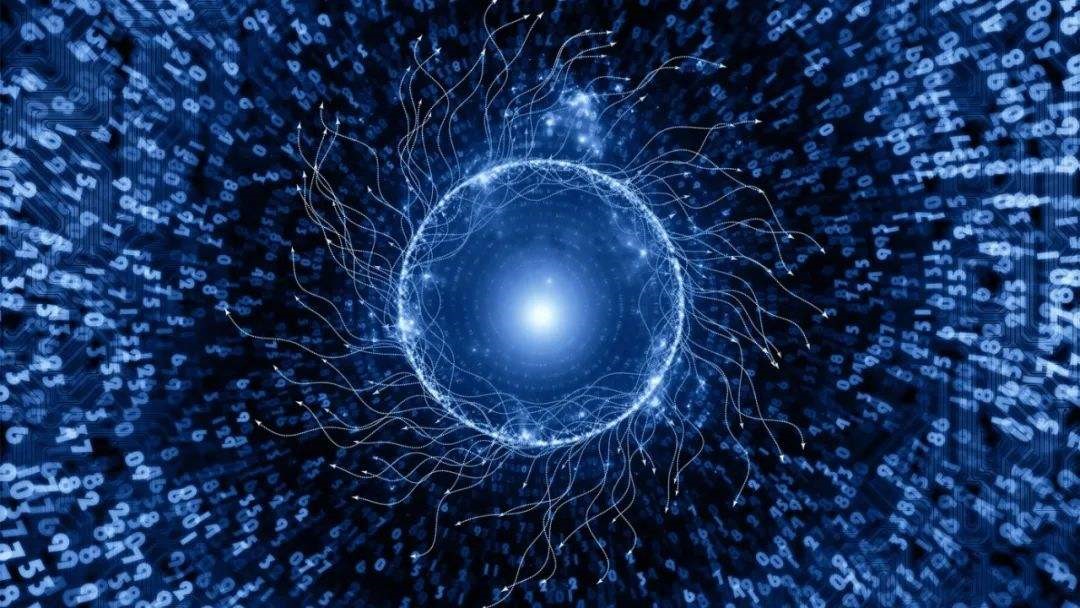
在上一期AI文献推荐中,我们为您推荐了人工智能领域触觉应用的热点论文,包括使用可伸缩的触觉手套学习人类抓握的特征,基于导电微结构气隙栅极和二维半导体晶体管的高灵敏度压力传感器,基于手部弯曲所产生摩擦电信号的触觉反馈智能手套,基于触觉视觉的自主机器人心内导管导航等文献。
本期我们为您选取了4篇文献,介绍深度学习与计算机视觉的最新动态,包括基于机器学习逆设计的三维矢量全息技术,基于上下文深层网络对单幅图像进行雨水检测与去除,一种利用交替方向乘子法的图像压缩传感深度网络体系结构,利用一种新的交叉熵损失(焦损)的损失函数实现高精度单级目标检测器RetinaNet等文献,推送给相关领域的科研人员。
领域一 基于机器学习逆设计的三维矢量全息技术
Three-dimensional vectorial holography based on machine learning inverse design
Ren, Haoran, etc.
SCIENCE ADVANCES, 2020, 6(16)
The three-dimensional (3D) vectorial nature of electromagnetic waves of light has not only played a fundamental role in science but also driven disruptive applications in optical display, microscopy, and manipulation. However, conventional optical holography can address only the amplitude and phase information of an optical beam, leaving the 3D vectorial feature of light completely inaccessible. We demonstrate 3D vectorial holography where an arbitrary 3D vectorial field distribution on a wavefront can be precisely reconstructed using the machine learning inverse design based on multilayer perceptron artificial neural networks. This 3D vectorial holography allows the lensless reconstruction of a 3D vectorial holographic image with an ultrawide viewing angle of 94 degrees and a high diffraction efficiency of 78%, necessary for floating displays. The results provide an artificial intelligence-enabled holographic paradigm for harnessing the vectorial nature of light, enabling new machine learning strategies for holographic 3D vectorial fields multiplexing in display and encryption.阅读原文 https://advances.sciencemag.org/content/6/16/eaaz4261.full
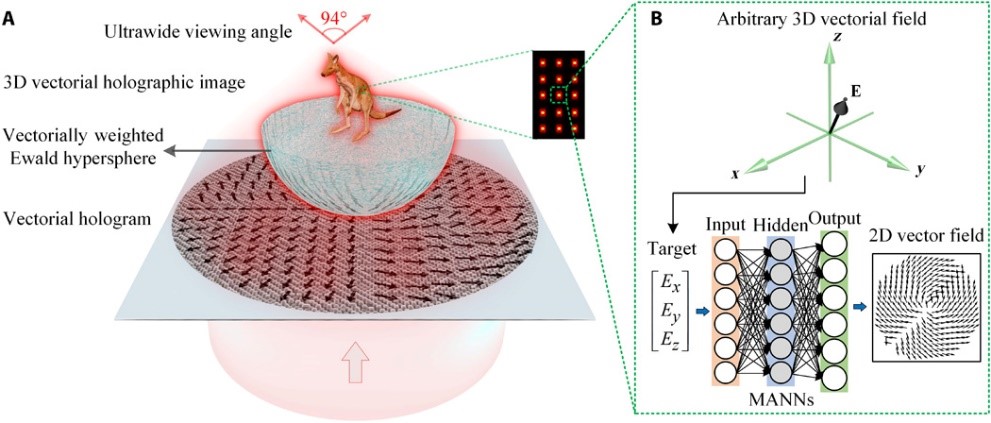
Principle of 3D vectorial holography based on the machine learning inverse design using the MANN
领域二 基于深层网络对单幅图像进行雨水检测与去除
Joint Rain Detection and Removal from a Single Image with Contextualized Deep Networks
Yang, Wenhan, etc.
IEEE TRANSACTIONS ON PATTERN ANALYSIS AND MACHINE INTELLIGENCE, 2020, 42(6): 1377-1393
Rain streaks, particularly in heavy rain, not only degrade visibility but also make many computer vision algorithms fail to function properly. In this paper, we address this visibility problem by focusing on single-image rain removal, even in the presence of dense rain streaks and rain-streak accumulation, which is visually similar to mist or fog. To achieve this, we introduce a new rain model and a deep learning architecture. Our rain model incorporates a binary rain map indicating rain-streak regions, and accommodates various shapes, directions, and sizes of overlapping rain streaks, as well as rain accumulation, to model heavy rain. Based on this model, we construct a multi-task deep network, which jointly learns three targets: the binary rain-streak map, rain streak layers, and clean background, which is our ultimate output. To generate features that can be invariant to rain steaks, we introduce a contextual dilated network, which is able to exploit regional contextual information. To handle various shapes and directions of overlapping rain streaks, our strategy is to utilize a recurrent process that progressively removes rain streaks. Our binary map provides a constraint and thus additional information to train our network. Extensive evaluation on real images, particularly in heavy rain, shows the effectiveness of our model and architecture.阅读原文 https://ieeexplore.ieee.org/document/8627954
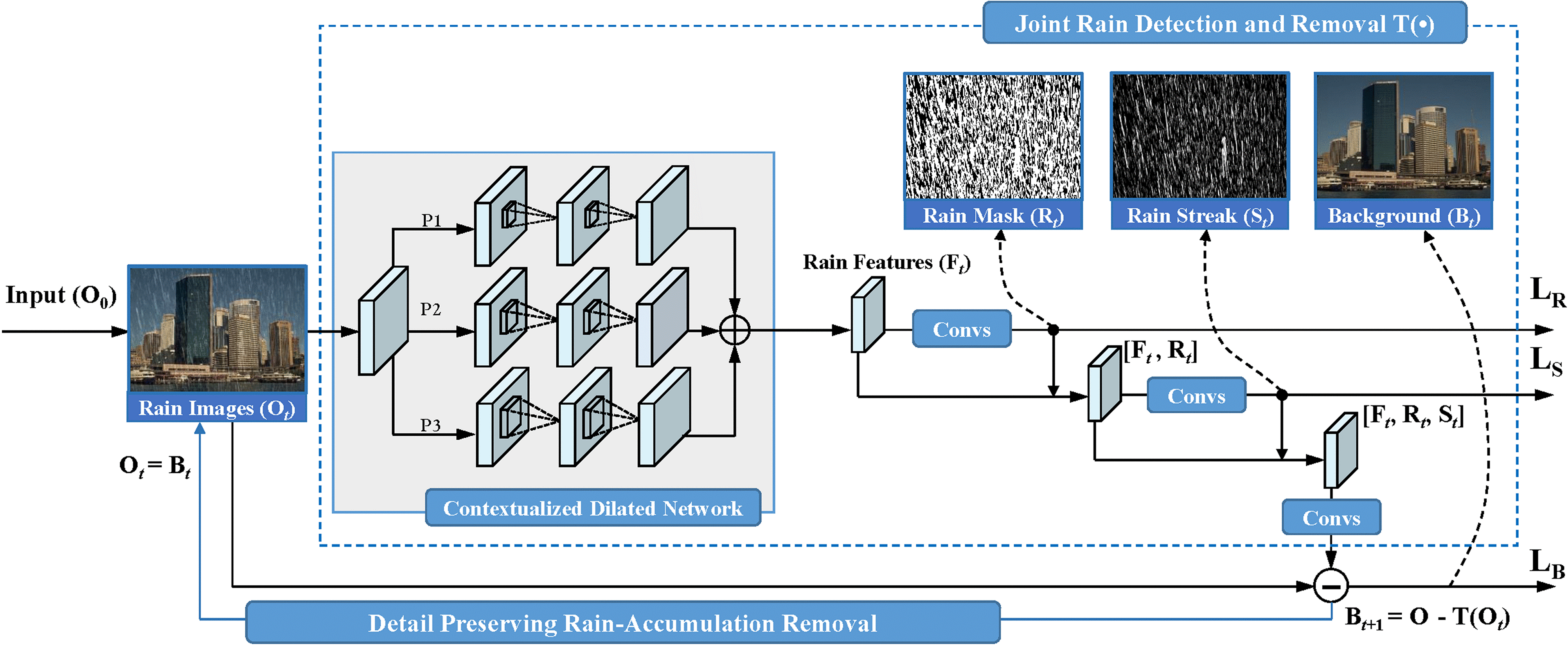
The architecture of the recurrent joint rain detection and removal method
领域三 一种利用交替方向乘子法的图像压缩传感深度学习方法
ADMM-CSNet: A Deep Learning Approach for Image Compressive Sensing
Yang, Yan, etc.
IEEE TRANSACTIONS ON PATTERN ANALYSIS AND MACHINE INTELLIGENCE, 2020, 42(3): 521-538
Compressive sensing (CS) is an effective technique for reconstructing image from a small amount of sampled data. It has been widely applied in medical imaging, remote sensing, image compression, etc. In this paper, we propose two versions of a novel deep learning architecture, dubbed as ADMM-CSNet, by combining the traditional model-based CS method and data-driven deep learning method for image reconstruction from sparsely sampled measurements. We first consider a generalized CS model for image reconstruction with undetermined regularizations in undetermined transform domains, and then two efficient solvers using Alternating Direction Method of Multipliers (ADMM) algorithm for optimizing the model are proposed. We further unroll and generalize the ADMM algorithm to be two deep architectures, in which all parameters of the CS model and the ADMM algorithm are discriminatively learned by end-to-end training. For both applications of fast CS complex-valued MR imaging and CS imaging of real-valued natural images, the proposed ADMM-CSNet achieved favorable reconstruction accuracy in fast computational speed compared with the traditional and the other deep learning methods.阅读原文 https://ieeexplore.ieee.org/document/8550778
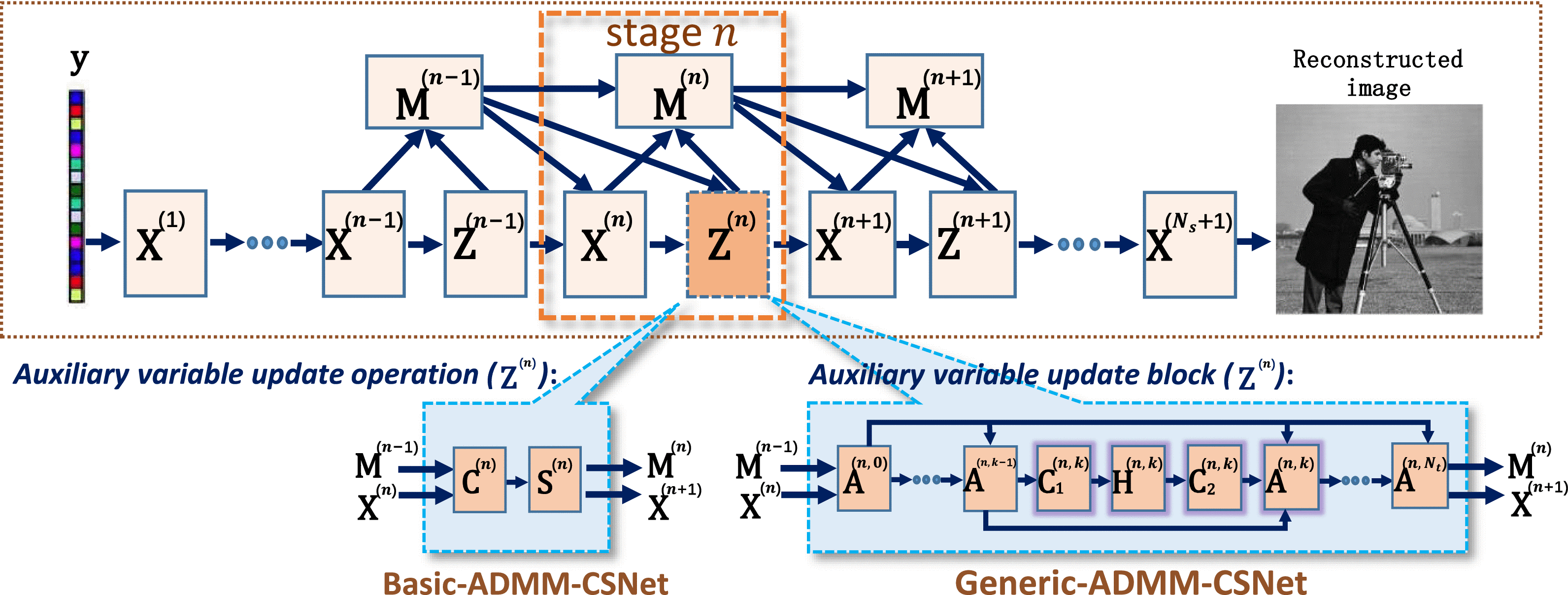
The data flow graph of ADMM-CSNets
领域四 利用一种新的损耗(焦损)实现高精度单级目标检测器
Dense Object Detection
Lin, Tsung-Yi, etc.
IEEE TRANSACTIONS ON PATTERN ANALYSIS AND MACHINE INTELLIGENCE, 2020, 42(2): 318-327
The highest accuracy object detectors to date are based on a two-stage approach popularized by R-CNN, where a classifier is applied to a sparse set of candidate object locations. In contrast, one-stage detectors that are applied over a regular, dense sampling of possible object locations have the potential to be faster and simpler, but have trailed the accuracy of two-stage detectors thus far. In this paper, we investigate why this is the case. We discover that the extreme foreground-background class imbalance encountered during training of dense detectors is the central cause. We propose to address this class imbalance by reshaping the standard cross entropy loss such that it down-weights the loss assigned to well-classified examples. Our novel Focal Loss focuses training on a sparse set of hard examples and prevents the vast number of easy negatives from overwhelming the detector during training. To evaluate the effectiveness of our loss, we design and train a simple dense detector we call RetinaNet. Our results show that when trained with the focal loss, RetinaNet is able to match the speed of previous one-stage detectors while surpassing the accuracy of all existing state-of-the-art two-stage detectors.阅读原文 https://ieeexplore.ieee.org/document/8417976
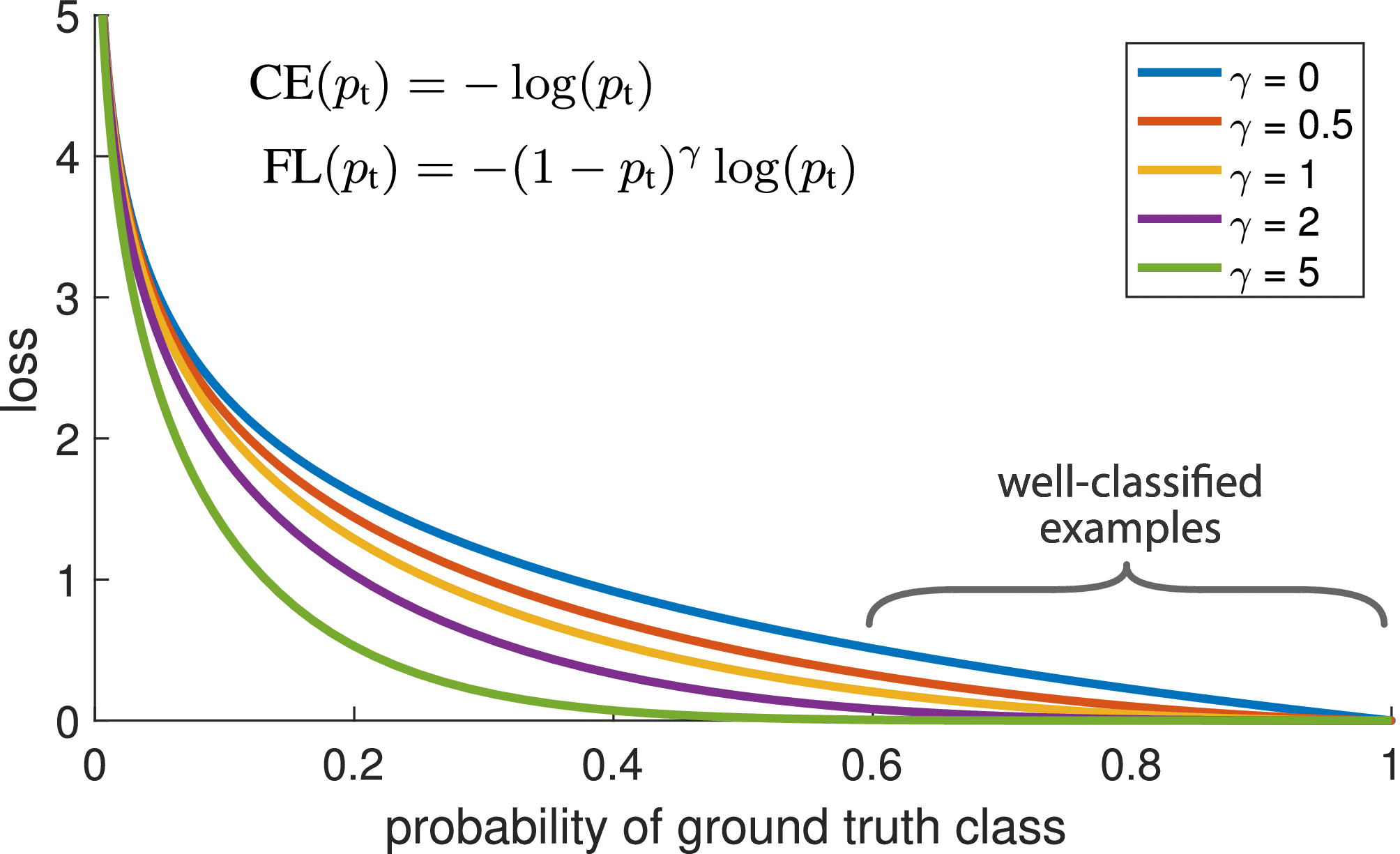
A novel loss Focal Loss that adds a factor to the standard cross entropy criterion which enables training highly accurate dense object detectors
往期精彩推荐
前沿论文带您解读5G应用领域 ——图书馆前沿文献专题推荐服务(2)
热点论文解读AI应用领域 ——图书馆前沿文献专题推荐服务(3)
热点论文带您探究5G和未来通信——图书馆前沿文献专题推荐服务 (4)
前沿文献带您解读自然语言处理技术 ——图书馆前沿文献专题推荐服务(5)
热点论文带您探究5G和未来通信材料技术领域 ——图书馆前沿文献专题推荐服务(6)
热点论文解读AI应用领域 ——图书馆前沿文献专题推荐服务(3)
热点论文带您探究5G和未来通信——图书馆前沿文献专题推荐服务 (4)
前沿文献带您解读自然语言处理技术 ——图书馆前沿文献专题推荐服务(5)
热点论文带您探究5G和未来通信材料技术领域 ——图书馆前沿文献专题推荐服务(6)
热点文献带您关注AI情感分类技术 ——图书馆前沿文献专题推荐服务(7)
热点论文带您探究6G的无限可能——图书馆前沿文献专题推荐服务(8)
热点文献带您关注AI文本摘要自动生成 ——图书馆前沿文献专题推荐服务(9)
热点论文:5G/6G引领社会新进步——图书馆前沿文献专题推荐服务(10)
热点文献带您关注AI机器翻译 ——图书馆前沿文献专题推荐服务(11)
热点论文与您探讨5G/6G网络技术新进展——图书馆前沿文献专题推荐服务(12)
热点文献带您关注AI计算机视觉 ——图书馆前沿文献专题推荐服务(13)
热点论文与带您领略5G/6G的硬科技与新思路 ——图书馆前沿文献专题推荐服务(14)
热点文献带您关注AI计算机视觉 ——图书馆前沿文献专题推荐服务(15)
热点论文带您领略5G/6G的最新技术动向 ——图书馆前沿文献专题推荐服务(18)
热点文献带您关注图神经网络——图书馆前沿文献专题推荐服务(19)
热点论文与带您领略5G/6G材料技术的最新发展——图书馆前沿文献专题推荐服务(20)
热点文献带您关注模式识别——图书馆前沿文献专题推荐服务(21)
热点论文与带您领略6G网络技术的最新发展趋势 ——图书馆前沿文献专题推荐服务(22)
热点文献带您关注机器学习与量子物理 ——图书馆前沿文献专题推荐服务(23)
热点论文与带您领略5G/6G通信器件材料的最新进展 ——图书馆前沿文献专题推荐服务(24)
热点文献带您关注AI自动驾驶——图书馆前沿文献专题推荐服务(25)
热点论文与带您领略5G/6G网络安全和技术的最新进展——图书馆前沿文献专题推荐服务(26)
热点文献带您关注AI神经网络与忆阻器——图书馆前沿文献专题推荐服务(27)
热点论文与带您领略5G/6G电子器件和太赫兹方面的最新进展——图书馆前沿文献专题推荐服务(28)
热点文献带您关注AI与机器人——图书馆前沿文献专题推荐服务(29)
热点论文与带您领略5G/6G热点技术的最新进展——图书馆前沿文献专题推荐服务(30)
热点文献带您关注AI与触觉传感技术——图书馆前沿文献专题推荐服务(31)
热点论文与带您领略5G/6G热点技术的最新进展——图书馆前沿文献专题推荐服务(32)