热点文献带您关注AI神经网络与忆阻器——图书馆前沿文献专题推荐服务(27)
2020-11-13
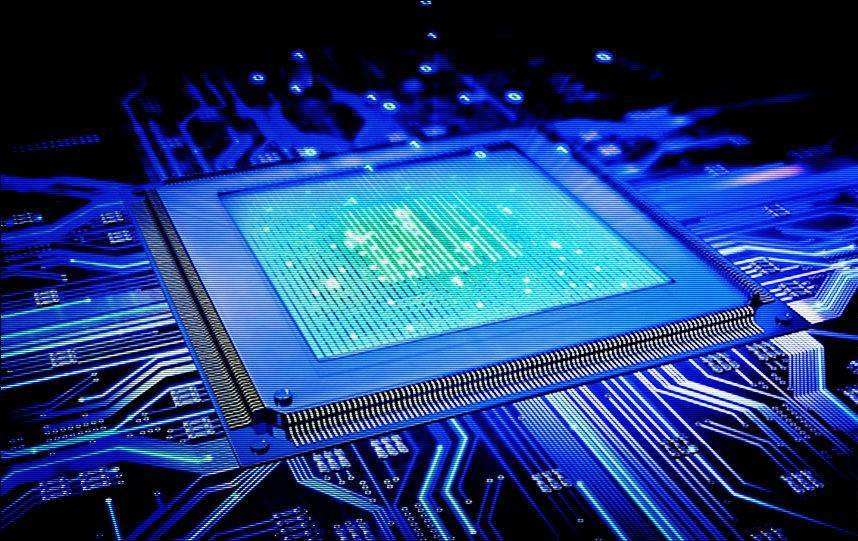
在上一期AI文献推荐中,我们为您推荐了人工智能在自动驾驶领域的热点论文。在本期推荐中,我们将为您带来神经网络与忆阻器的前沿论文。
随着人工智能的高速发展,神经网络内存计算架构吸引了很多研究者的兴趣。作为功能接近神经元突触的电子器件,忆阻器成为近几年研究神经网络硬件电路的热点。
本期选取了4篇文献,介绍人工智能神经网络与忆阻器的最新动态,包括完全由硬件实现的基于忆阻器的五层卷积神经网络,采用由八层忆阻器件构成的3D电路的卷积神经网络,用于移动机器人的基于忆阻器的混合模数计算平台,具有在线最小均方的基于忆阻器的回声状态网络等文献,推送给相关领域的科研人员。
Fully hardware-implemented memristor convolutional neural network
Yao, Peng, etc.
NATURE, 2020, 577(7792) : 641-646
Memristor-enabled neuromorphic computing systems provide a fast and energy-efficient approach to training neural networks(1-4). However, convolutional neural networks (CNNs)-one of the most important models for image recognition(5)-have not yet been fully hardware-implemented using memristor crossbars, which are cross-point arrays with a memristor device at each intersection. Moreover, achieving software-comparable results is highly challenging owing to the poor yield, large variation and other non-ideal characteristics of devices(6-9). Here we report the fabrication of high-yield, high-performance and uniform memristor crossbar arrays for the implementation of CNNs, which integrate eight 2,048-cell memristor arrays to improve parallel-computing efficiency. In addition, we propose an effective hybrid-training method to adapt to device imperfections and improve the overall system performance. We built a five-layer memristor-based CNN to perform MNIST10 image recognition, and achieved a high accuracy of more than 96 per cent. In addition to parallel convolutions using different kernels with shared inputs, replication of multiple identical kernels in memristor arrays was demonstrated for processing different inputs in parallel. The memristor-based CNN neuromorphic system has an energy efficiency more than two orders of magnitude greater than that of state-of-the-art graphics-processing units, and is shown to be scalable to larger networks, such as residual neural networks. Our results are expected to enable a viable memristor-based non-von Neumann hardware solution for deep neural networks and edge computing.阅读原文 https://www.nature.com/articles/s41586-020-1942-4
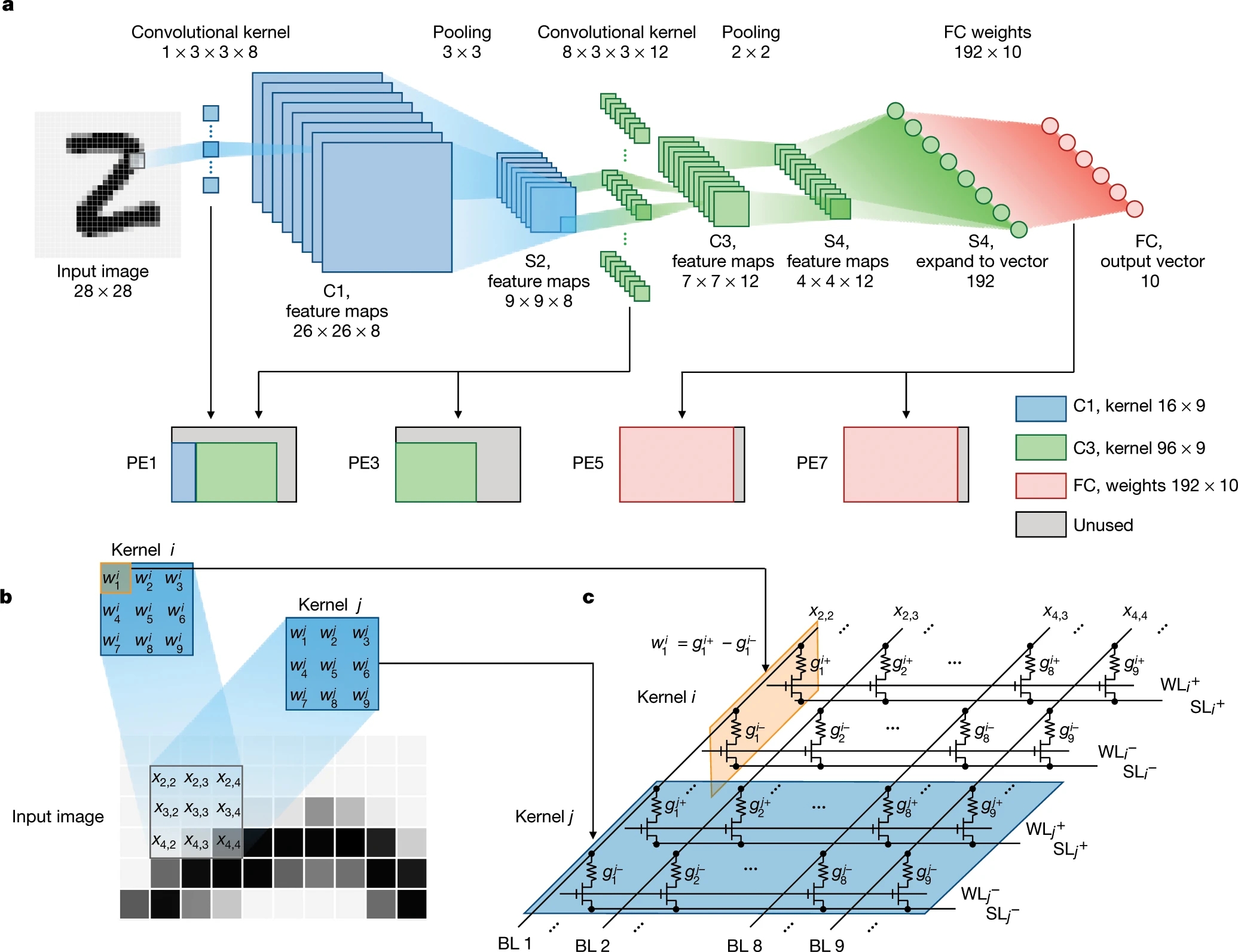
Five-layer mCNN with memristor convolver
Three-dimensional memristor circuits as complex neural networks
Lin, Peng, etc.
NATURE ELECTRONICS, 2020, 3(4): 225-232
Constructing a computing circuit in three dimensions (3D) is a necessary step to enable the massive connections and efficient communications required in complex neural networks. 3D circuits based on conventional complementary metal-oxide-semiconductor transistors are, however, difficult to build because of challenges involved in growing or stacking multilayer single-crystalline silicon channels. Here we report a 3D circuit composed of eight layers of monolithically integrated memristive devices. The vertically aligned input and output electrodes in our 3D structure make it possible to directly map and implement complex neural networks. As a proof-of-concept demonstration, we programmed parallelly operated kernels into the 3D array, implemented a convolutional neural network and achieved software-comparable accuracy in recognizing handwritten digits from the Modified National Institute of Standard and Technology database. We also demonstrated the edge detection of moving objects in videos by applying groups of Prewitt filters in the 3D array to process pixels in parallel.A three-dimensional circuit composed of eight layers of monolithically integrated memristive devices is built and used to implement complex neural networks, demonstrating accurate MNIST classification and effective edge detection in videos.
阅读原文 https://www.nature.com/articles/s41928-020-0397-9
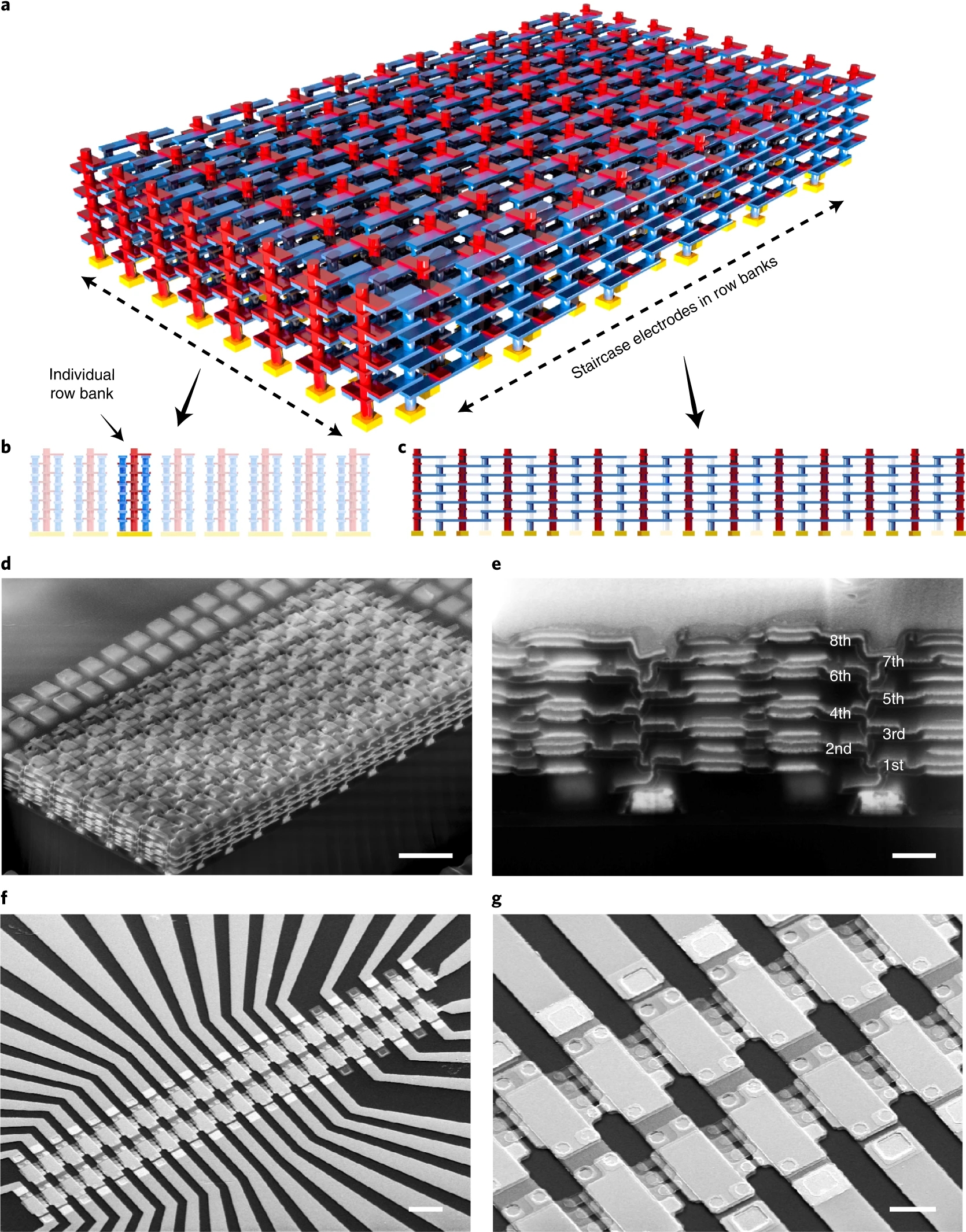
3D monolithic integrated memristor circuits
A memristor-based hybrid analog-digital computing platform for mobile robotics
Chen, Buyun, etc.
SCIENCE ROBOTICS, 2020, 5(47)
Algorithms for mobile robotic systems are generally implemented on purely digital computing platforms. Developing alternative computational platforms may lead to more energy-efficient and responsive mobile robotics. Here, we report a hybrid analog-digital computing platform enabled by memristors on a mobile inverted pendulum robot. Our mobile robotic system can tune the conductance states of memristors adaptively using a model-free optimization method to achieve optimal control performance. We implement sensor fusion and the motion control algorithms on our hybrid analog-digital computing platform and demonstrate more than one order of magnitude enhancement of speed and energy efficiency over traditional digital platforms.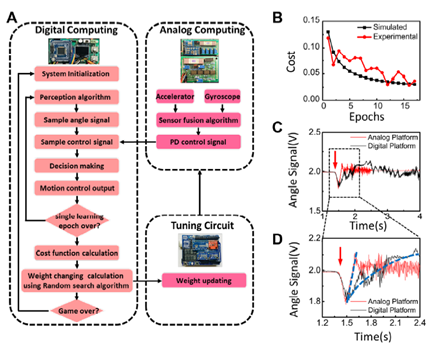
The adaptive learning process on the hybrid computing platform and the optimal control performance
Memristor-Based Echo State Network With Online Least Mean Square
Wen, Shiping, etc.
IEEE TRANSACTIONS ON SYSTEMS MAN CYBERNETICS-SYSTEMS, 2019, 49(9): 1787-1796
In this paper, we propose a novel computational architecture of memristor-based echo state network (MESN) with the online least mean square (LMS) algorithm. Newman and Watts small-world network is adopted for the topological structure of MESN network with memristive neural synapses. In the MESN network, the state matrix of the reservoir layer, which is obtained by raising the dimension of input data, is utilized as an input of the LMS algorithm to train the output weight matrix on chip. After certain iterations, the resistance value of memristor is adjusted to a constant. Thus, the final weight output matrix is obtained. To verify the effectiveness of the proposed MESN network, car evaluation and short-term power load forecasting are employed with the effect evaluation of the node number and the connectivity degree of the reservoir layer. The research provides a novel way to design neuromorphic computing systems.阅读原文 https://ieeexplore.ieee.org/document/8354924
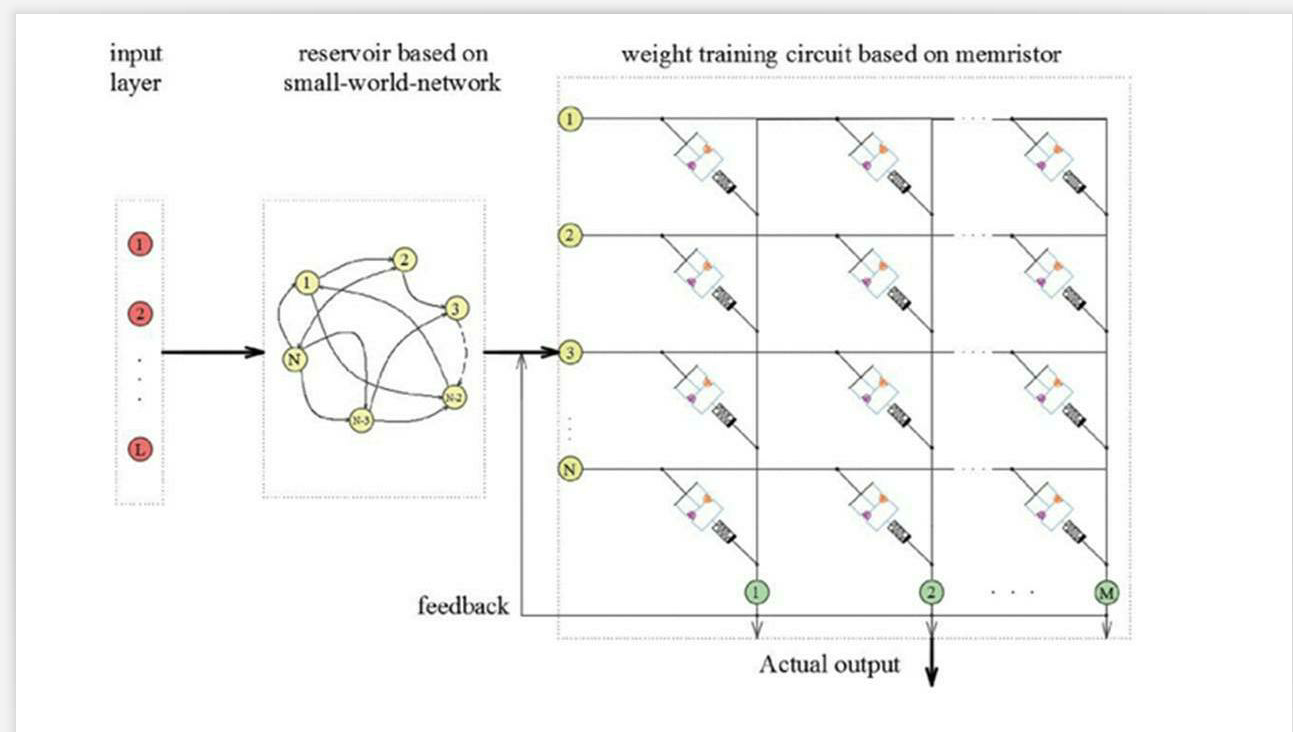
Architecture overview of MESN
前沿论文带您解读5G应用领域 ——图书馆前沿文献专题推荐服务(2)
热点论文解读AI应用领域 ——图书馆前沿文献专题推荐服务(3)
热点论文带您探究5G和未来通信——图书馆前沿文献专题推荐服务 (4)
前沿文献带您解读自然语言处理技术 ——图书馆前沿文献专题推荐服务(5)
热点论文带您探究5G和未来通信材料技术领域 ——图书馆前沿文献专题推荐服务(6)
热点论文解读AI应用领域 ——图书馆前沿文献专题推荐服务(3)
热点论文带您探究5G和未来通信——图书馆前沿文献专题推荐服务 (4)
前沿文献带您解读自然语言处理技术 ——图书馆前沿文献专题推荐服务(5)
热点论文带您探究5G和未来通信材料技术领域 ——图书馆前沿文献专题推荐服务(6)
热点文献带您关注AI情感分类技术 ——图书馆前沿文献专题推荐服务(7)
热点论文带您探究6G的无限可能——图书馆前沿文献专题推荐服务(8)
热点文献带您关注AI文本摘要自动生成 ——图书馆前沿文献专题推荐服务(9)
热点论文:5G/6G引领社会新进步——图书馆前沿文献专题推荐服务(10)
热点文献带您关注AI机器翻译 ——图书馆前沿文献专题推荐服务(11)
热点论文与您探讨5G/6G网络技术新进展——图书馆前沿文献专题推荐服务(12)
热点文献带您关注AI计算机视觉 ——图书馆前沿文献专题推荐服务(13)
热点论文与带您领略5G/6G的硬科技与新思路 ——图书馆前沿文献专题推荐服务(14)
热点文献带您关注AI计算机视觉 ——图书馆前沿文献专题推荐服务(15)
热点论文带您领略5G/6G的最新技术动向 ——图书馆前沿文献专题推荐服务(18)
热点文献带您关注图神经网络——图书馆前沿文献专题推荐服务(19)
热点论文与带您领略5G/6G材料技术的最新发展——图书馆前沿文献专题推荐服务(20)
热点文献带您关注模式识别——图书馆前沿文献专题推荐服务(21)
热点论文与带您领略6G网络技术的最新发展趋势 ——图书馆前沿文献专题推荐服务(22)
热点文献带您关注机器学习与量子物理 ——图书馆前沿文献专题推荐服务(23)
热点论文与带您领略5G/6G通信器件材料的最新进展 ——图书馆前沿文献专题推荐服务(24)
热点文献带您关注AI自动驾驶——图书馆前沿文献专题推荐服务(25)
热点论文与带您领略5G/6G网络安全和技术的最新进展——图书馆前沿文献专题推荐服务(26)