热点文献带您关注AI情感分类技术 ——图书馆前沿文献专题推荐服务(7)
2020-04-17
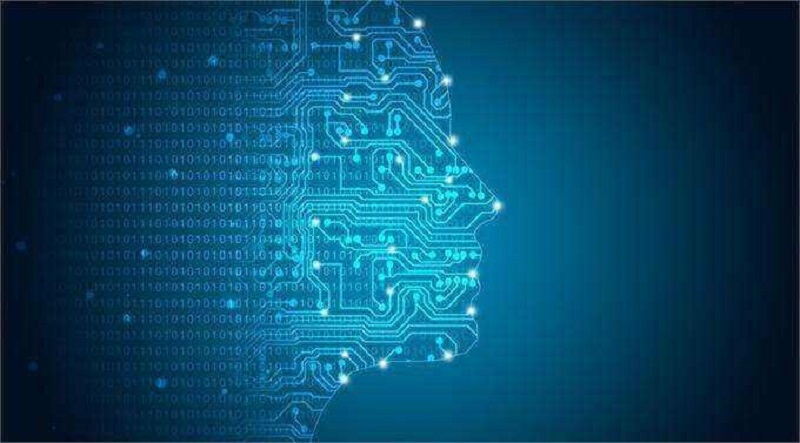
在上一期人工智能自然语言处理技术的推荐中,我们从四大常见任务展示了自然语言处理技术的应用。在本期推荐中,我们继续介绍其中情感分类的技术与应用。
情感分类是自然语言处理领域的一个重要任务。通过情感分类,我们可以判断情感偏向并挖掘深层次的情感信息。情感分类方法从传统的基于情感词典的方法,发展到基于机器学习的方法、基于深度学习的方法、基于注意力机制的方法等。本期选取了4篇文献,介绍情感分类的最新动态,包括用于情感分析的深度学习比较与综述、利用卷积神经网络(CNN)与LSTM建模,具有注意力机制的神经网络模型等,推送给相关领域的科研人员。

Deep Learning for Aspect-Based Sentiment Analysis: A Comparative Review
Do, Hai Ha, etc.
EXPERT SYSTEMS WITH APPLICATIONS, 2019, 118: 272-299
The increasing volume of user-generated content on the web has made sentiment analysis an important tool for the extraction of information about the human emotional state. A current research focus for sentiment analysis is the improvement of granularity at aspect level, representing two distinct aims: aspect extraction and sentiment classification of product reviews and sentiment classification of target-dependent tweets. Deep learning approaches have emerged as a prospect for achieving these aims with their ability to capture both syntactic and semantic features of text without requirements for high-level feature engineering, as is the case in earlier methods. In this article, we aim to provide a comparative review of deep learning for aspect-based sentiment analysis to place different approaches in context.

Speech emotion recognition using deep 1D & 2D CNN LSTM networks
Zhao, Jianfeng, etc.
BIOMEDICAL SIGNAL PROCESSING AND CONTROL, 2019, 47: 312-323
We aimed at learning deep emotion features to recognize speech emotion. Two convolutional neural network and long short-term memory (CNN LSTM) networks, one 1D CNN LSTM network and one 2D CNN LSTM network, were constructed to learn local and global emotion-related features from speech and logmel spectrogram respectively. The two networks have the similar architecture, both consisting of four local feature learning blocks (LFLBs) and one long short-term memory (LSTM) layer. LFLB, which mainly contains one convolutional layer and one max-pooling layer, is built for learning local correlations along with extracting hierarchical correlations. LSTM layer is adopted to learn long-term dependencies from the learned local features. The designed networks, combinations of the convolutional neural network (CNN) and LSTM, can take advantage of the strengths of both networks and overcome the shortcomings of them, and are evaluated on two benchmark databases. The experimental results show that the designed networks achieve excellent performance on the task of recognizing speech emotion, especially the 2D CNN LSTM network outperforms the traditional approaches, Deep Belief Network (DBN) and CNN on the selected databases. The 2D CNN LSTM network achieves recognition accuracies of 95.33% and 95.89% on Berlin EmoDB of speakerdependent and speaker-independent experiments respectively, which compare favourably to the accuracy of 91.6% and 92.9% obtained by traditional approaches; and also yields recognition accuracies of 89.16% and 52.14% on IEMOCAP database of speaker-dependent and speaker-independent experiments, which are much higher than the accuracy of 73.78% and 40.02% obtained by DBN and CNN.

Carrying out consensual Group Decision Making processes under social networks using sentiment analysis over comparative expressions
Morente-Molinera, J. A., etc.
KNOWLEDGE-BASED SYSTEMS, 2019, 165: 335-345
Social networks are the most preferred mean for the people to communicate. Therefore, it is quite usual that experts use them to carry out Group Decision Making processes. One disadvantage that recent Group Decision Making methods have is that they do not allow the experts to use free text to express themselves. On the contrary, they force them to follow a specific user-computer communication structure. This is against social network nature where experts are free to express themselves using their preferred text structure. This paper presents a novel model for experts to carry out Group Decision Making processes using free text and alternatives pairwise comparisons. The main advantage of this method is that it is designed to work using social networks. Sentiment analysis procedures are used to analyze free texts and extract the preferences that the experts provide about the alternatives. Also, our method introduces two ways of applying consensus measures over the Group Decision Making process. They can be used to determine if the experts agree among them or if there are different postures. This way, it is possible to promote the debate in those cases where consensus is low.

Feature-Based Fusion Adversarial Recurrent Neural Networks for Text Sentiment Classification
Ma, Yaohong, etc.
IEEE ACCESS, 2019, 7: 132542-132551
Text sentiment classification is a fundamental task of natural language processing. In the past few years, many outstanding methods have attained favorable results in text sentiment classification. However, most of these methods do not make full use of the contextual information of the word embedding layer and attach less importance to the loss of information in the process of forwarding propagation. Hence, an ample room exists for further progress in enhancing the robustness of the model and the feature extraction of text. To tackle these problems, we propose a Feature-Based Fusion Adversarial Recurrent Neural Networks (FARNN-Att) integrated model with an attention mechanism. Firstly, we extract the long-term dependence of text using the BiLSTM network and put forward a novel contextual feature representation way. Subsequently, we combine the prediction results of two features vectors in the full connection layer, which can be captured through the feature connection and attention mechanism. Finally, a regularization method of adversarial training is used to improve the robustness and generalization ability of the model. Our proposed model was compared with other baseline methods such as TextCNN, BiLSTM, BiLSTM-Att, and RCNN on three different public datasets. The experimental results show that our model has state-of-the-art performance in text sentiment classification tasks in terms of accuracy, recall, and F1 score.
往期精彩推荐
《Nature》带您探究人工智能世界——图书馆前沿文献专题推荐服务(1)
前沿论文带您解读5G应用领域 ——图书馆前沿文献专题推荐服务(2)
热点论文解读AI应用领域 ——图书馆前沿文献专题推荐服务(3)
热点论文带您探究5G和未来通信——图书馆前沿文献专题推荐服务 (4)
前沿文献带您解读自然语言处理技术 ——图书馆前沿文献专题推荐服务(5)
热点论文带您探究5G和未来通信材料技术领域 ——图书馆前沿文献专题推荐服务(6)
热点论文解读AI应用领域 ——图书馆前沿文献专题推荐服务(3)
热点论文带您探究5G和未来通信——图书馆前沿文献专题推荐服务 (4)
前沿文献带您解读自然语言处理技术 ——图书馆前沿文献专题推荐服务(5)
热点论文带您探究5G和未来通信材料技术领域 ——图书馆前沿文献专题推荐服务(6)