热点论文解读AI应用领域 ——图书馆前沿文献专题推荐服务(3)
2020-03-18
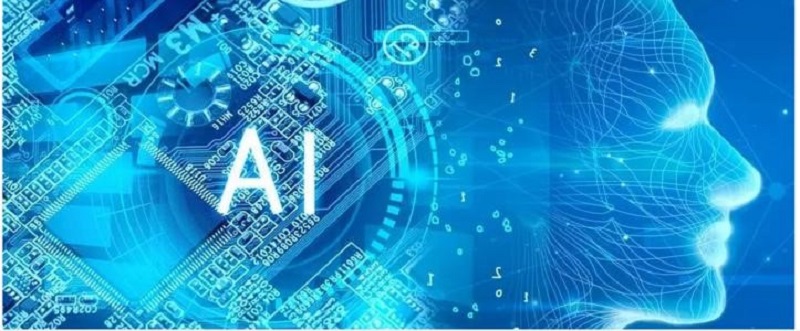
新一代人工智能技术正在全球范围蓬勃发展,推动世界进入智能信息时代,给人们的生产生活方式带来颠覆性影响。人工智能作为计算机学科的一个分支,包括神经网络、机器学习、深度学习、自然语言处理、计算机视觉、语音和音频处理等研究范畴,在计算机、通信、医学、仿生学、材料科学等学科均有飞速发展。在此,我们为您推荐以Nature、Science等为代表的国际前沿期刊上的人工智能领域相关文献,以期能够引起您的学术共鸣。
本期我们为您推送5篇文献,从不同领域关注人工智能的发展。

A general reinforcement learning algorithm that masters chess, shogi, and Go through self-play
Silver, David, etc.
SCIENCE, 2018, 362(6419)
The game of chess is the longest-studied domain in the history of artificial intelligence. The strongest programs are based on a combination of sophisticated search techniques, domain-specific adaptations, and handcrafted evaluation functions that have been refined by human experts over several decades. By contrast, the AlphaGo Zero program recently achieved superhuman performance in the game of Go by reinforcement learning from self-play. In this paper, we generalize this approach into a single AlphaZero algorithm that can achieve superhuman performance in many challenging games. Starting from random play and given no domain knowledge except the game rules, AlphaZero convincingly defeated a world champion program in the games of chess and shogi (Japanese chess), as well as Go.

All-optical spiking neurosynaptic networks with self-learning capabilities
Feldmann, J., etc.
NATURE, 2019, 569(7755)
Software implementations of brain-inspired computing underlie many important computational tasks, from image processing to speech recognition, artificial intelligence and deep learning applications. Yet, unlike real neural tissue, traditional computing architectures physically separate the core computing functions of memory and processing, making fast, efficient and low-energy computing difficult to achieve. To overcome such limitations, an attractive alternative is to design hardware that mimics neurons and synapses. Such hardware, when connected in networks or neuromorphic systems, processes information in a way more analogous to brains. Here we present an all-optical version of such a neurosynaptic system, capable of supervised and unsupervised learning. We exploit wavelength division multiplexing techniques to implement a scalable circuit architecture for photonic neural networks, successfully demonstrating pattern recognition directly in the optical domain. Such photonic neurosynaptic networks promise access to the high speed and high bandwidth inherent to optical systems, thus enabling the direct processing of optical telecommunication and visual data.

High-performance medicine: the convergence of human and artificial intelligence
Topol, Eric J.
NATURE MEDICINE, 2019, 25(1)
The use of artificial intelligence, and the deep-learning subtype in particular, has been enabled by the use of labeled big data, along with markedly enhanced computing power and cloud storage, across all sectors. In medicine, this is beginning to have an impact at three levels: for clinicians, predominantly via rapid, accurate image interpretation; for health systems, by improving workflow and the potential for reducing medical errors; and for patients, by enabling them to process their own data to promote health. The current limitations, including bias, privacy and security, and lack of transparency, along with the future directions of these applications will be discussed in this article. Over time, marked improvements in accuracy, productivity, and workflow will likely be actualized, but whether that will be used to improve the patient-doctor relationship or facilitate its erosion remains to be seen.

Machine learning for molecular and materials science
Butler, Keith T., etc.
NATURE, 2018, 559(7715)
Here we summarize recent progress in machine learning for the chemical sciences. We outline machine-learning techniques that are suitable for addressing research questions in this domain, as well as future directions for the field. We envisage a future in which the design, synthesis, characterization and application of molecules and materials is accelerated by artificial intelligence.

Deep learning and process understanding for data-driven Earth system science
Reichstein, Markus, etc.
NATURE, 2019, 566(7743)
Machine learning approaches are increasingly used to extract patterns and insights from the ever-increasing stream of geospatial data, but current approaches may not be optimal when system behaviour is dominated by spatial or temporal context. Here, rather than amending classical machine learning, we argue that these contextual cues should be used as part of deep learning (an approach that is able to extract spatio-temporal features automatically) to gain further process understanding of Earth system science problems, improving the predictive ability of seasonal forecasting and modelling of long-range spatial connections across multiple timescales, for example. The next step will be a hybrid modelling approach, coupling physical process models with the versatility of data-driven machine learning.
往期精彩推荐
《Nature》带您探究人工智能世界——图书馆前沿文献专题推荐服务(1)