热点文献带您关注机器学习与量子物理 ——图书馆前沿文献专题推荐服务(23)
2020-10-19
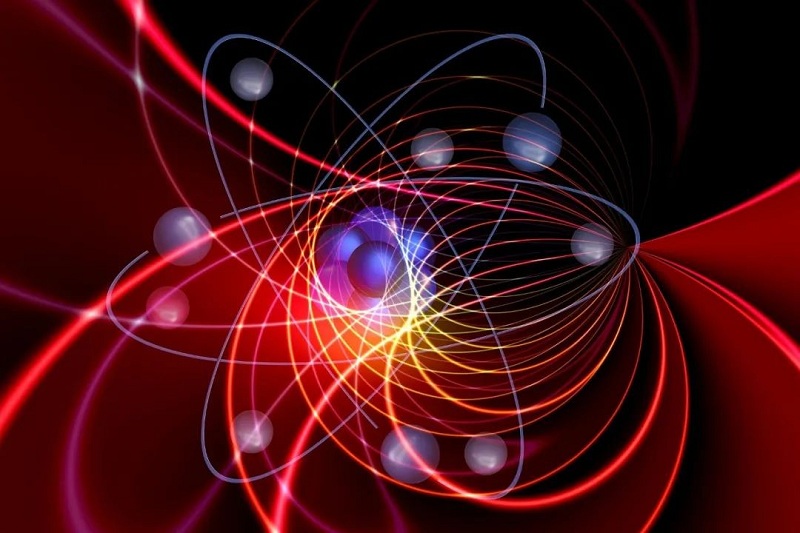
在上一期AI文献推荐中,我们为您推荐了模式识别的热点论文。在本期推荐中,我们将为您推荐人工智能与量子物理的前沿论文。
机器学习是目前最活跃的跨学科领域之一,它与量子物理虽然是两个截然不同的学科,但它们在数据科学方面的结合引起了学术界的高度关注,日后必将为各领域带来巨大的收获。
本期选取了4篇文献,介绍机器学习与量子物理跨学科的最新动态,包括具有量子增强特征空间的监督学习,使用量子经典混合机在Bars-Stripes数据集上实现数据驱动的量子电路训练算法,机器学习在粒子物理学和宇宙学、量子多体物理学、量子计算以及化学和材料物理学中的应用等文献,推送给相关领域的科研人员。
领域一 具有量子增强特征空间的监督学习
Supervised learning with quantum-enhanced feature spaces
Havlicek, Vojtech, etc.
NATURE, 2019, 567(7747): 209-212
Machine learning and quantum computing are two technologies that each have the potential to alter how computation is performed to address previously untenable problems. Kernel methods for machine learning are ubiquitous in pattern recognition, with support vector machines (SVMs) being the best known method for classification problems. However, there are limitations to the successful solution to such classification problems when the feature space becomes large, and the kernel functions become computationally expensive to estimate. A core element in the computational speed-ups enabled by quantum algorithms is the exploitation of an exponentially large quantum state space through controllable entanglement and interference. Here we propose and experimentally implement two quantum algorithms on a superconducting processor. A key component in both methods is the use of the quantum state space as feature space. The use of a quantum-enhanced feature space that is only efficiently accessible on a quantum computer provides a possible path to quantum advantage. The algorithms solve a problem of supervised learning: the construction of a classifier. One method, the quantum variational classifier, uses a variational quantum circuit( 1,2) to classify the data in away similar to the method of conventional SVMs. The other method, a quantum kernel estimator, estimates the kernel function on the quantum computer and optimizes a classical SVM. The two methods provide tools for exploring the applications of noisy intermediate-scale quantum computers(3) to machine learning.阅读原文 https://www.nature.com/articles/s41586-019-0980-2
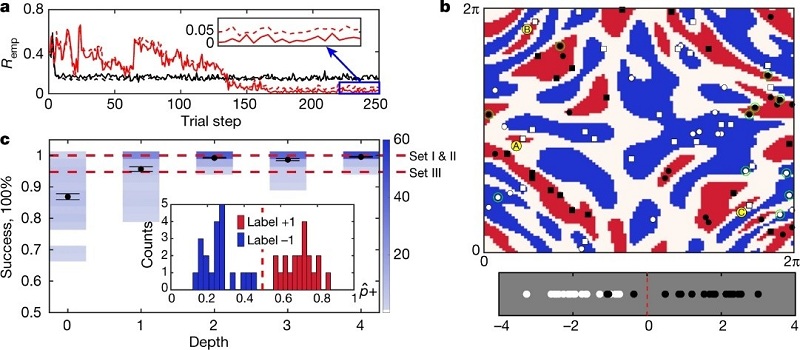
Convergence of the method and classification results
领域二 在混合量子计算机上训练量子电路
Training of quantum circuits on a hybrid quantum computer
Zhu, D., etc.
SCIENCE ADVANCES, 2019, 5(10)
Generative modeling is a flavor of machine learning with applications ranging from computer vision to chemical design. It is expected to be one of the techniques most suited to take advantage of the additional resources provided by near-term quantum computers. Here, we implement a data-driven quantum circuit training algorithm on the canonical Bars-and-Stripes dataset using a quantum-classical hybrid machine. The training proceeds by running parameterized circuits on a trapped ion quantum computer and feeding the results to a classical optimizer. We apply two separate strategies, Particle Swarm and Bayesian optimization to this task. We show that the convergence of the quantum circuit to the target distribution depends critically on both the quantum hardware and classical optimization strategy. Our study represents the first successful training of a high-dimensional universal quantum circuit and highlights the promise and challenges associated with hybrid learning schemes.阅读原文 https://advances.sciencemag.org/content/5/10/eaaw9918
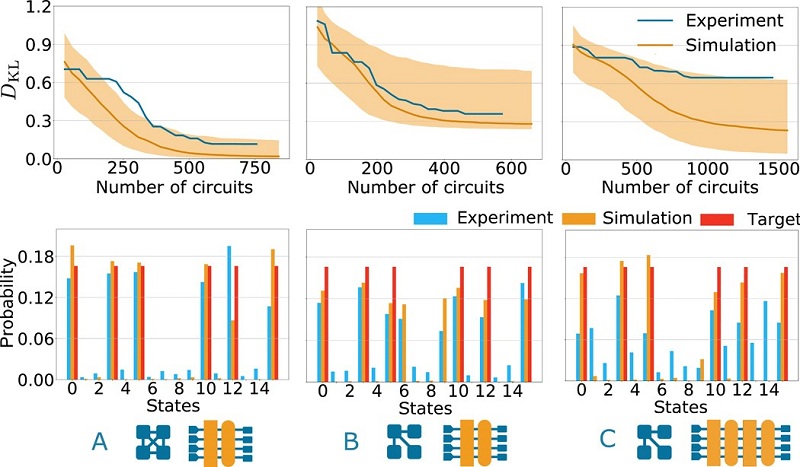
Quantum circuit training results with Particle Swarm Optimization, with simulations (orange) and trapped ion quantum computer results (blue)
领域三 机器学习在物理学、宇宙学、化学和材料物理学中的应用
Machine learning and the physical sciences
Carleo, Giuseppe, etc.
REVIEWS OF MODERN PHYSICS, 2019, 91(4)
Machine learning (ML) encompasses a broad range of algorithms and modeling tools used for a vast array of data processing tasks, which has entered most scientific disciplines in recent years. This article reviews in a selective way the recent research on the interface between machine learning and the physical sciences. This includes conceptual developments in ML motivated by physical insights, applications of machine learning techniques to several domains in physics, and cross fertilization between the two fields. After giving a basic notion of machine learning methods and principles, examples are described of how statistical physics is used to understand methods in ML. This review then describes applications of ML methods in particle physics and cosmology, quantum many-body physics, quantum computing, and chemical and material physics. Research and development into novel computing architectures aimed at accelerating ML are also highlighted. Each of the sections describe recent successes as well as domain-specific methodology and challenges.阅读原文 http://export.arxiv.org/pdf/1903.10563
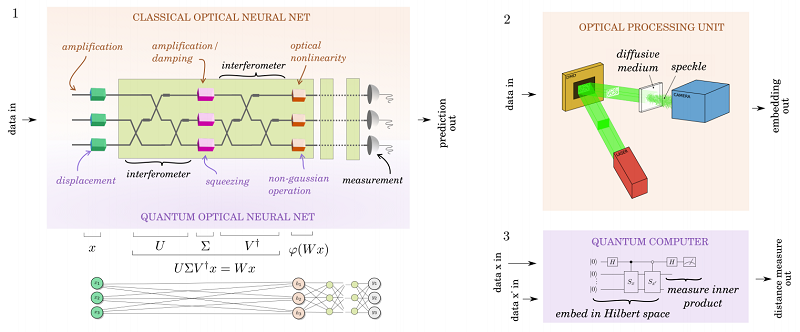
Illustrations of the methods discussed in the text
领域四 量子多体态的神经网络表示
Neural network representations of quantum many-body states
Yang, Ying, etc.
SCIENCE CHINA-PHYSICS MECHANICS & ASTRONOMY, 2020, 63(1)
Machine learning is currently the most active interdisciplinary field having numerous applications; additionally, machine-learning techniques are used to research quantum many-body problems. In this study, we first propose neural network quantum states (NNQSs) with general input observables and explore a few related properties, such as the tensor product and local unitary operation. Second, we determine the necessary and sufficient conditions for the representability of a general graph state using normalized NNQS. Finally, to quantify the approximation degree of a given pure state, we de fi ne the best approximation degree using normalized NNQSs. Furthermore, we observe that some N-qubit states can be represented by a normalized NNQS, such as separable pure states, Bell states and GHZ states.阅读原文 https://kns.cnki.net/KCMS/detail/detail.aspx?dbcode=CJFD&filename=JGXG202001003
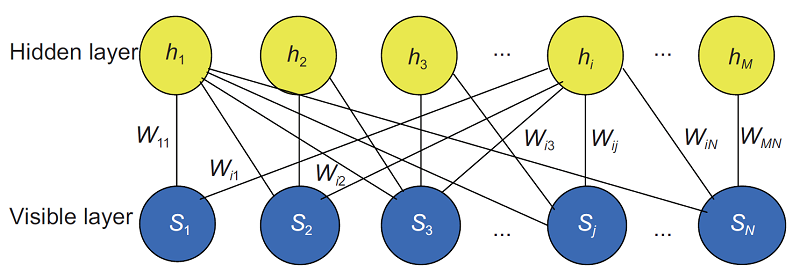
Artificial neural network encoding an NNQS
前沿论文带您解读5G应用领域 ——图书馆前沿文献专题推荐服务(2)
热点论文解读AI应用领域 ——图书馆前沿文献专题推荐服务(3)
热点论文带您探究5G和未来通信——图书馆前沿文献专题推荐服务 (4)
前沿文献带您解读自然语言处理技术 ——图书馆前沿文献专题推荐服务(5)
热点论文带您探究5G和未来通信材料技术领域 ——图书馆前沿文献专题推荐服务(6)
热点论文解读AI应用领域 ——图书馆前沿文献专题推荐服务(3)
热点论文带您探究5G和未来通信——图书馆前沿文献专题推荐服务 (4)
前沿文献带您解读自然语言处理技术 ——图书馆前沿文献专题推荐服务(5)
热点论文带您探究5G和未来通信材料技术领域 ——图书馆前沿文献专题推荐服务(6)
热点文献带您关注AI情感分类技术 ——图书馆前沿文献专题推荐服务(7)
热点论文带您探究6G的无限可能——图书馆前沿文献专题推荐服务(8)
热点文献带您关注AI文本摘要自动生成 ——图书馆前沿文献专题推荐服务(9)
热点论文:5G/6G引领社会新进步——图书馆前沿文献专题推荐服务(10)
热点文献带您关注AI机器翻译 ——图书馆前沿文献专题推荐服务(11)
热点论文与您探讨5G/6G网络技术新进展——图书馆前沿文献专题推荐服务(12)
热点文献带您关注AI计算机视觉 ——图书馆前沿文献专题推荐服务(13)
热点论文与带您领略5G/6G的硬科技与新思路 ——图书馆前沿文献专题推荐服务(14)
热点文献带您关注AI计算机视觉 ——图书馆前沿文献专题推荐服务(15)