热点文献带您关注AI自动驾驶——图书馆前沿文献专题推荐服务(25)
2020-10-30
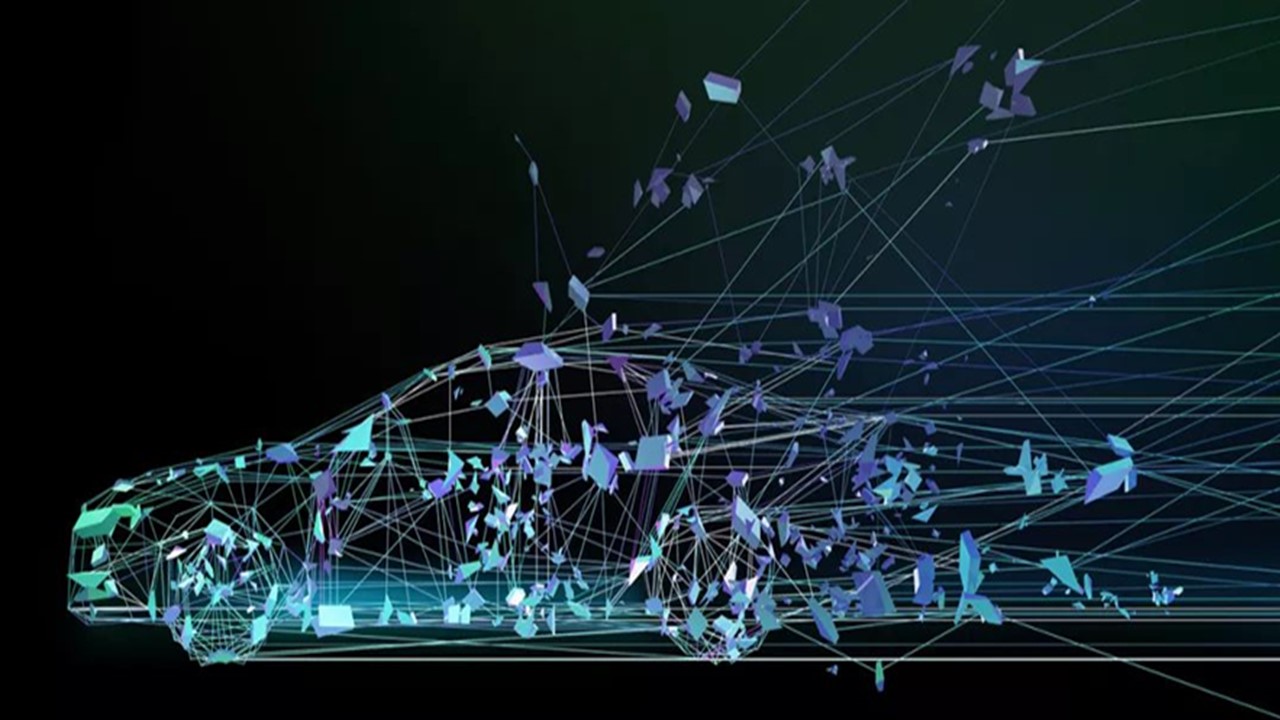
在上一期AI文献推荐中,我们为您推荐了人工智能与量子物理的热点论文。在本期推荐中,我们将为您带来人工智能在自动驾驶领域的前沿论文。
作为解决未来交通出行的重要方式,自动驾驶已成为全球范围内的新的技术研究热点,而人工智能技术在环境智能感知、传感、控制等关键技术的完善与突破则大大推动了自动驾驶技术的发展。
本期选取了4篇文献,介绍人工智能在自动驾驶领域的最新动态,包括使用数据驱动算法的增强型自动驾驶模拟,结合神经计算原理和深度学习的紧凑型神经控制器,采用课程领域自适应方法的城市场景语义分割,一种易于训练且可以基于高维数据建模的生成对抗性密度估计器等文献,推送给相关领域的科研人员。
领域一 数据驱动算法的增强型自动驾驶仿真
AADS: Augmented autonomous driving simulation using data-driven algorithms
Li, W., etc.
SCIENCE ROBOTICS, 2019, 4(28)
Simulation systems have become essential to the development and validation of autonomous driving (AD) technologies. The prevailing state-of-the-art approach for simulation uses game engines or high-fidelity computer graphics (CG) models to create driving scenarios. However, creating CG models and vehicle movements (the assets for simulation) remain manual tasks that can be costly and time consuming. In addition, CG images still lack the richness and authenticity of real-world images, and using CG images for training leads to degraded performance. Here, we present our augmented autonomous driving simulation (AADS). Our formulation augmented real-world pictures with a simulated traffic flow to create photorealistic simulation images and renderings. More specifically, we used LiDAR and cameras to scan street scenes. From the acquired trajectory data, we generated plausible traffic flows for cars and pedestrians and composed them into the background. The composite images could be resynthesized with different viewpoints and sensor models (camera or LiDAR). The resulting images are photorealistic, fully annotated, and ready for training and testing of AD systems from perception to planning. We explain our system design and validate our algorithms with a number of AD tasks from detection to segmentation and predictions. Compared with traditional approaches, our method offers scalability and realism. Scalability is particularly important for AD simulations, and we believe that real-world complexity and diversity cannot be realistically captured in a virtual environment. Our augmented approach combines the flexibility of a virtual environment (e.g., vehicle movements) with the richness of the real world to allow effective simulation.阅读原文 https://robotics.sciencemag.org/content/4/28/eaaw0863
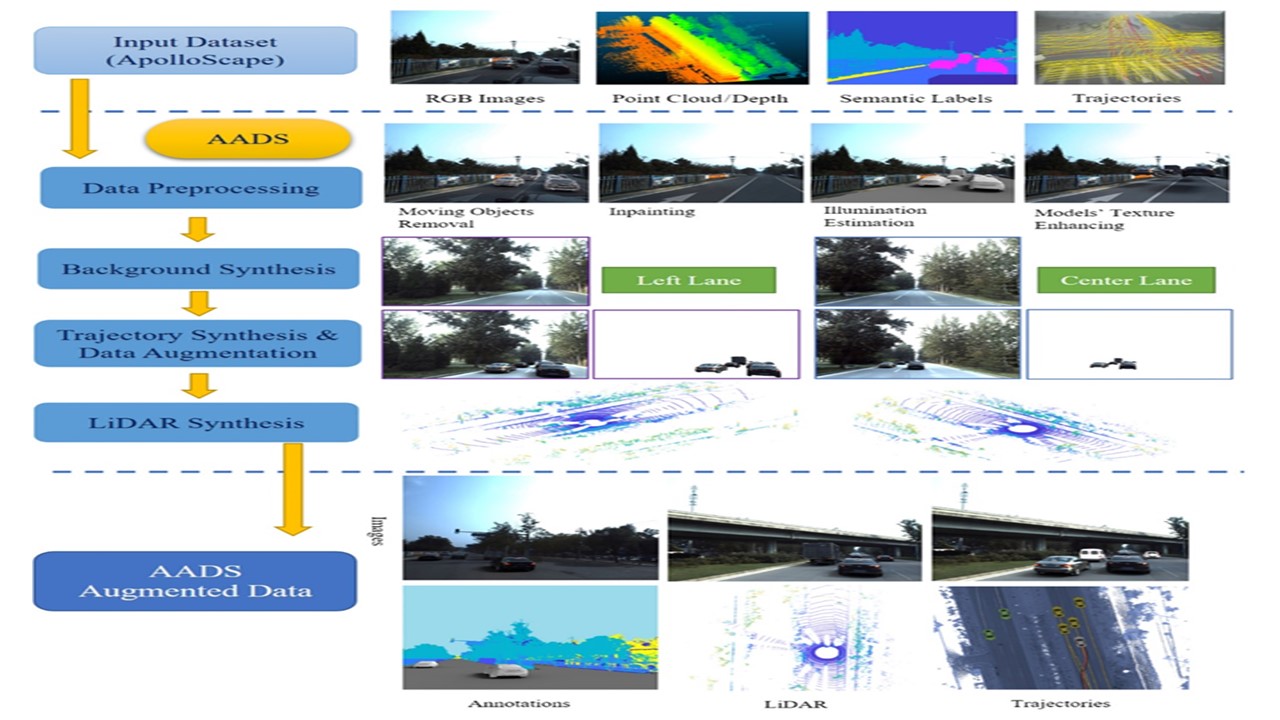
领域二 用于全栈自动驾驶控制系统的紧凑型神经控制器
Neural circuit policies enabling auditable autonomy
Zhu, D., etc.
Nature Machine Intelligence, 2020, 2: 642–652
A central goal of artificial intelligence in high-stakes decision-making applications is to design a single algorithm that simultaneously expresses generalizability by learning coherent representations of their world and interpretable explanations of its dynamics. Here, we combine brain-inspired neural computation principles and scalable deep learning architectures to design compact neural controllers for task-specific compartments of a full-stack autonomous vehicle control system. We discover that a single algorithm with 19 control neurons, connecting 32 encapsulated input features to outputs by 253 synapses, learns to map high-dimensional inputs into steering commands. This system shows superior generalizability, interpretability and robustness compared with orders-of-magnitude larger black-box learning systems. The obtained neural agents enable high-fidelity autonomy for task-specific parts of a complex autonomous system.
领域三 城市场景语义分割的课程领域自适应方法
A Curriculum Domain Adaptation Approach to the Semantic Segmentation of Urban Scenes
Zhang, Yang, etc.
IEEE TRANSACTIONS ON PATTERN ANALYSIS AND MACHINE INTELLIGENCE, 2020, 42(8): 1823-1841
During the last half decade, convolutional neural networks (CNNs) have triumphed over semantic segmentation, which is one of the core tasks in many applications such as autonomous driving and augmented reality. However, to train CNNs requires a considerable amount of data, which is difficult to collect and laborious to annotate. Recent advances in computer graphics make it possible to train CNNs on photo-realistic synthetic imagery with computer-generated annotations. Despite this, the domain mismatch between real images and the synthetic data hinders the models' performance. Hence, we propose a curriculum-style learning approach to minimizing the domain gap in urban scene semantic segmentation. The curriculum domain adaptation solves easy tasks first to infer necessary properties about the target domain; in particular, the first task is to learn global label distributions over images and local distributions over landmark superpixels. These are easy to estimate because images of urban scenes have strong idiosyncrasies (e.g., the size and spatial relations of buildings, streets, cars, etc.). We then train a segmentation network, while regularizing its predictions in the target domain to follow those inferred properties. In experiments, our method outperforms the baselines on two datasets and three backbone networks. We also report extensive ablation studies about our approach.阅读原文 https://ieeexplore.ieee.org/document/8661514
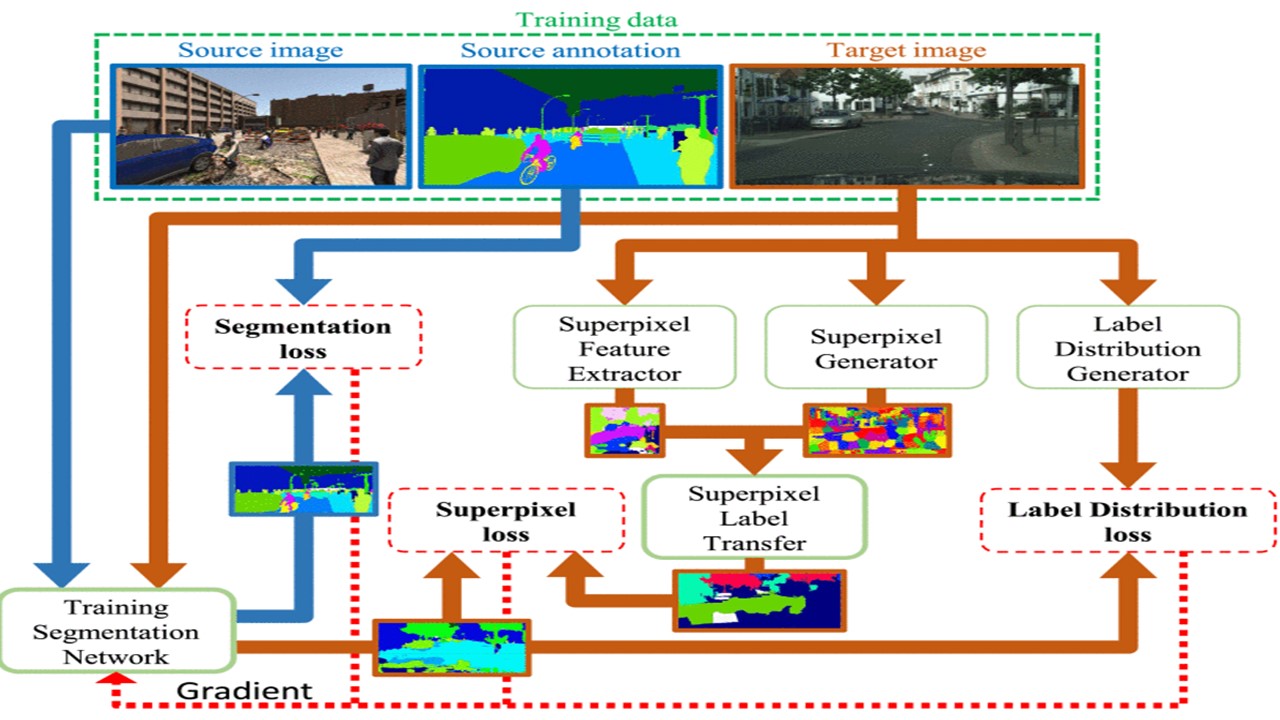
领域四 密度估计的生成对抗方法及其应用
GADE: A Generative Adversarial Approach to Density Estimation and its Applications
Abbasnejad, M. Ehsan, etc.
INTERNATIONAL JOURNAL OF COMPUTER VISION, 2020, 128(10-11) SI: 2731-2743
Density estimation is a challenging unsupervised learning problem. Current maximum likelihood approaches for density estimation are either restrictive or incapable of producing high-quality samples. On the other hand, likelihood-free models such as generative adversarial networks, produce sharp samples without a density model. The lack of a density estimate limits the applications to which the sampled data can be put, however. We propose a generative adversarial density estimator (GADE), a density estimation approach that bridges the gap between the two. Allowing for a prior on the parameters of the model, we extend our density estimator to a Bayesian model where we can leverage the predictive variance to measure our confidence in the likelihood. Our experiments on challenging applications such as visual dialog or autonomous driving where the density and the confidence in predictions are crucial shows the effectiveness of our approach.阅读原文 https://link.springer.com/article/10.1007/s11263-020-01360-9
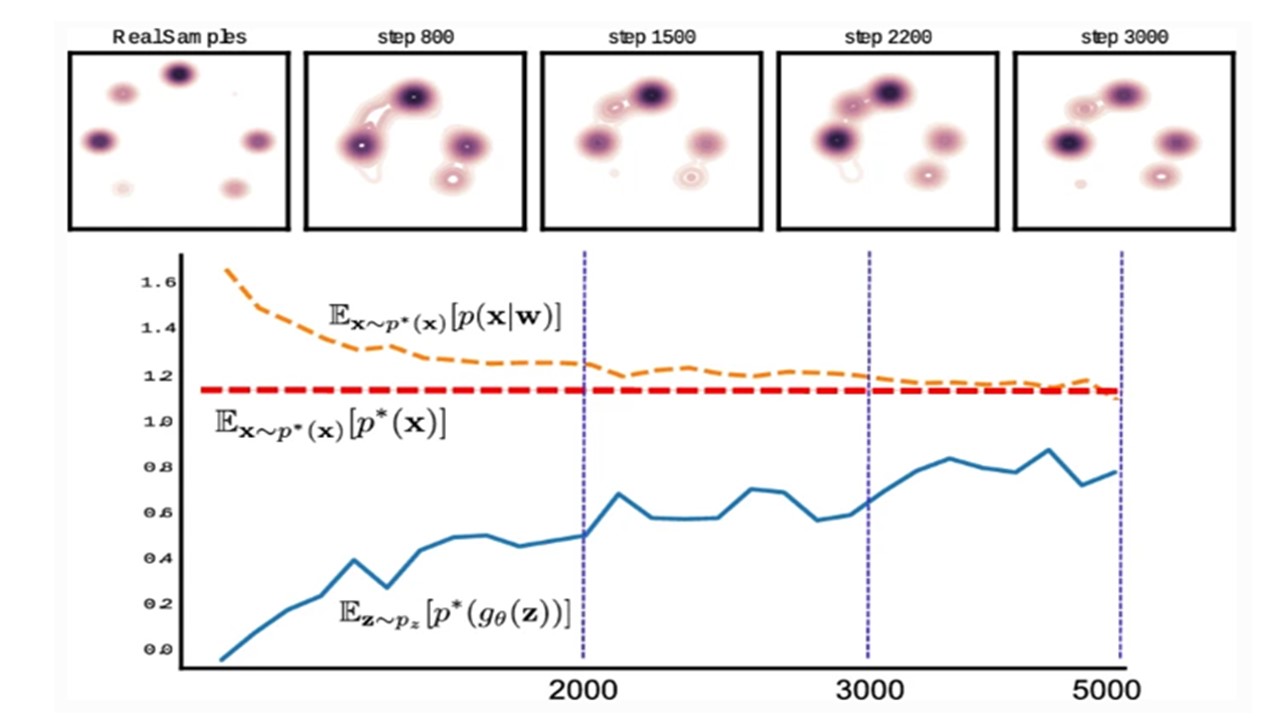
前沿论文带您解读5G应用领域 ——图书馆前沿文献专题推荐服务(2)
热点论文解读AI应用领域 ——图书馆前沿文献专题推荐服务(3)
热点论文带您探究5G和未来通信——图书馆前沿文献专题推荐服务 (4)
前沿文献带您解读自然语言处理技术 ——图书馆前沿文献专题推荐服务(5)
热点论文带您探究5G和未来通信材料技术领域 ——图书馆前沿文献专题推荐服务(6)
热点论文解读AI应用领域 ——图书馆前沿文献专题推荐服务(3)
热点论文带您探究5G和未来通信——图书馆前沿文献专题推荐服务 (4)
前沿文献带您解读自然语言处理技术 ——图书馆前沿文献专题推荐服务(5)
热点论文带您探究5G和未来通信材料技术领域 ——图书馆前沿文献专题推荐服务(6)
热点文献带您关注AI情感分类技术 ——图书馆前沿文献专题推荐服务(7)
热点论文带您探究6G的无限可能——图书馆前沿文献专题推荐服务(8)
热点文献带您关注AI文本摘要自动生成 ——图书馆前沿文献专题推荐服务(9)
热点论文:5G/6G引领社会新进步——图书馆前沿文献专题推荐服务(10)
热点文献带您关注AI机器翻译 ——图书馆前沿文献专题推荐服务(11)
热点论文与您探讨5G/6G网络技术新进展——图书馆前沿文献专题推荐服务(12)
热点文献带您关注AI计算机视觉 ——图书馆前沿文献专题推荐服务(13)
热点论文与带您领略5G/6G的硬科技与新思路 ——图书馆前沿文献专题推荐服务(14)
热点文献带您关注AI计算机视觉 ——图书馆前沿文献专题推荐服务(15)