热点文献带您关注AI与生物学——图书馆前沿文献专题推荐服务(47)
2021-10-15
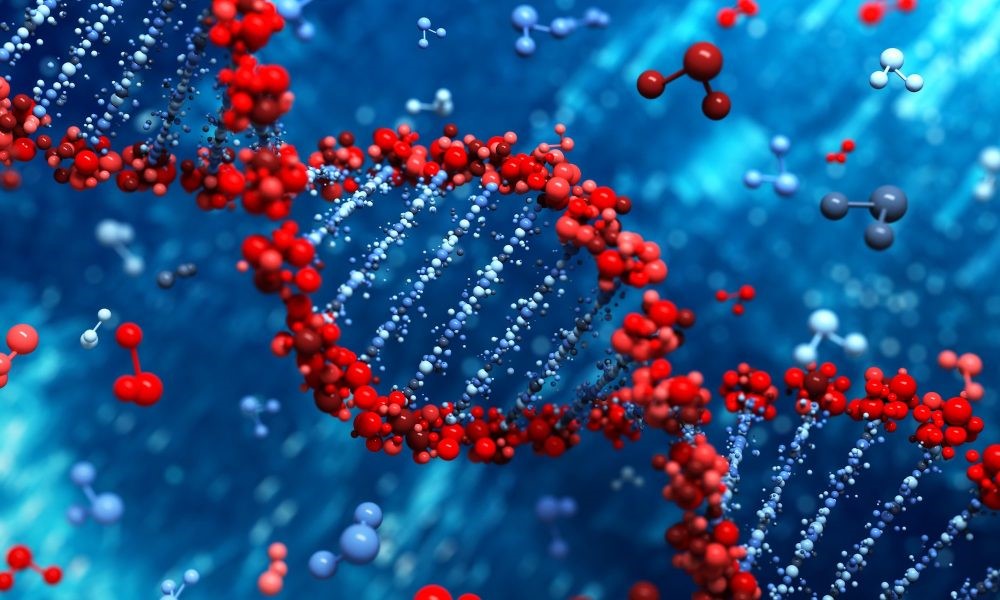
在上一期AI文献推荐中,我们为您推荐了人工智能在医学图像领域的热点论文,包括结合边缘计算与区块链的去中心化分散机器学习Swarm Learning技术、基于深度学习的图像分割方法、人工智能在心血管成像中的应用、利用深度学习通过眼底照片预测光学相干断层扫描成像得出糖尿病黄斑水肿分级等方面的文献。
本期我们为您选取了4篇文献,介绍AI在生物学领域的热点文献,包括提高RNA结构预测能力的深度学习方法、应用深度学习设计高度多样化的AAV衣壳蛋白变体、利用基于深度学习的精确估计指导改进蛋白质结构、利用递归神经网络寻找基因网络拓扑等文献,推送给相关领域的科研人员。
文献一 提高RNA结构预测能力的深度学习方法
Geometric deep learning of RNA structure
Townshend, Raphael J. L., etc.
SCIENCE, 2021, 373(6558): 1047-1051
RNA molecules adopt three-dimensional structures that are critical to their function and of interest in drug discovery. Few RNA structures are known, however, and predicting them computationally has proven challenging. We introduce a machine learning approach that enables identification of accurate structural models without assumptions about their defining characteristics, despite being trained with only 18 known RNA structures. The resulting scoring function, the Atomic Rotationally Equivariant Scorer (ARES), substantially outperforms previous methods and consistently produces the best results in community-wide blind RNA structure prediction challenges. By learning effectively even from a small amount of data, our approach overcomes a major limitation of standard deep neural networks. Because it uses only atomic coordinates as inputs and incorporates no RNA-specific information, this approach is applicable to diverse problems in structural biology, chemistry, materials science, and beyond.
阅读原文 https://www.science.org/doi/10.1126/science.abe5650
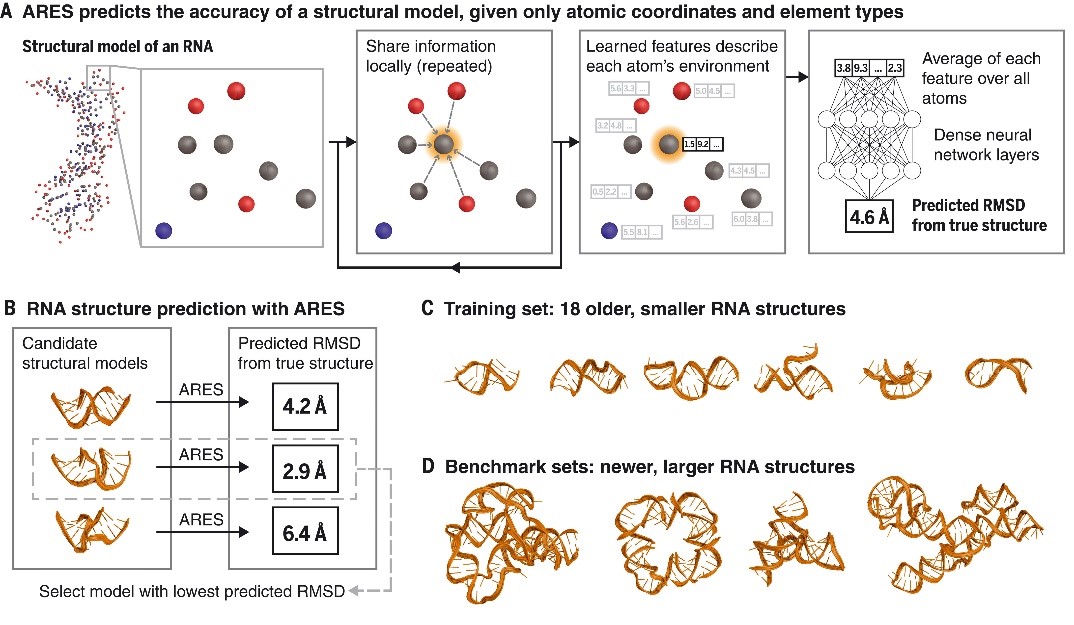
文献二 应用深度学习设计多样的AAV衣壳蛋白变体
Deep diversification of an AAV capsid protein by machine learning
Bryant, Drew H., etc.
Nature Biotechnology, 2021, 39(6): 691–696
Modern experimental technologies can assay large numbers of biological sequences, but engineered protein libraries rarely exceed the sequence diversity of natural protein families. Machine learning (ML) models trained directly on experimental data without biophysical modeling provide one route to accessing the full potential diversity of engineered proteins. Here we apply deep learning to design highly diverse adeno-associated virus 2 (AAV2) capsid protein variants that remain viable for packaging of a DNA payload. Focusing on a 28-amino acid segment, we generated 201,426 variants of the AAV2 wild-type (WT) sequence yielding 110,689 viable engineered capsids, 57,348 of which surpass the average diversity of natural AAV serotype sequences, with 12-29 mutations across this region. Even when trained on limited data, deep neural network models accurately predict capsid viability across diverse variants. This approach unlocks vast areas of functional but previously unreachable sequence space, with many potential applications for the generation of improved viral vectors and protein therapeutics.
阅读原文 https://www.nature.com/articles/s41587-020-00793-4
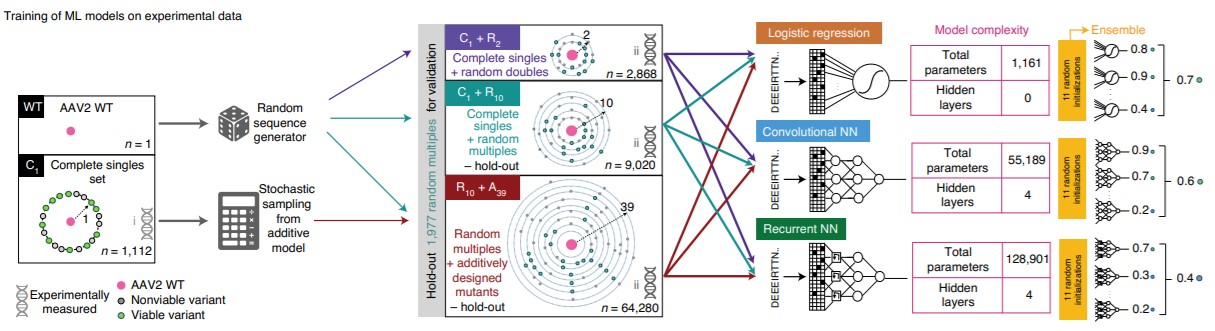
文献三 利用基于深度学习的精确估计指导改进蛋白质结构
Improved protein structure refinement guided by deep learning based accuracy estimation
Hiranuma, Naozumi, etc.
NATURE COMMUNICATIONS, 2021, 12(1)
We develop a deep learning framework (DeepAccNet) that estimates per-residue accuracy and residue-residue distance signed error in protein models and uses these predictions to guide Rosetta protein structure refinement. The network uses 3D convolutions to evaluate local atomic environments followed by 2D convolutions to provide their global contexts and outperforms other methods that similarly predict the accuracy of protein structure models. Overall accuracy predictions for X-ray and cryoEM structures in the PDB correlate with their resolution, and the network should be broadly useful for assessing the accuracy of both predicted structure models and experimentally determined structures and identifying specific regions likely to be in error. Incorporation of the accuracy predictions at multiple stages in the Rosetta refinement protocol considerably increased the accuracy of the resulting protein structure models, illustrating how deep learning can improve search for global energy minima of biomolecules. Here the authors present DeepAccNet, a deep learning framework that estimates per-residue accuracy and residue-residue distance signed error in protein models, which are used to guide Rosetta protein structure refinement. Benchmarking suggests an improvement of accuracy prediction and refinement compared to other related state of the art methods.
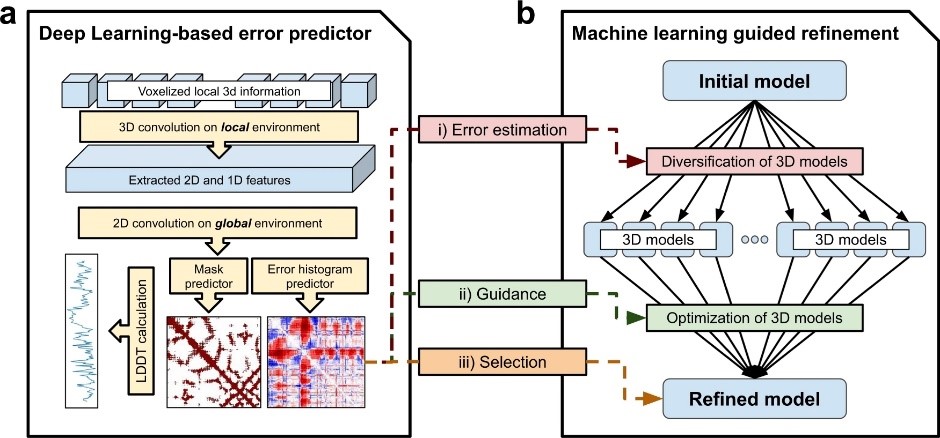
阅读原文 https://www.nature.com/articles/s41467-021-21511-x
文献四 利用递归神经网络寻找基因网络拓扑
Finding gene network topologies for given biological function with recurrent neural network
Shen, Jingxiang, etc.
NATURE COMMUNICATIONS, 2021, 12(1)
Searching for possible biochemical networks that perform a certain function is a challenge in systems biology. For simple functions and small networks, this can be achieved through an exhaustive search of the network topology space. However, it is difficult to scale this approach up to larger networks and more complex functions. Here we tackle this problem by training a recurrent neural network (RNN) to perform the desired function. By developing a systematic perturbative method to interrogate the successfully trained RNNs, we are able to distill the underlying regulatory network among the biological elements (genes, proteins, etc.). Furthermore, we show several cases where the regulation networks found by RNN can achieve the desired biological function when its edges are expressed by more realistic response functions, such as the Hill-function. This method can be used to link topology and function by helping uncover the regulation logic and network topology for complex tasks. Networks are useful ways to describe interactions between molecules in a cell, but predicting the real topology of large networks can be challenging. Here, the authors use deep learning to predict the topology of networks that perform biologically-plausible functions.
阅读原文 https://www.nature.com/articles/s41467-021-23420-5
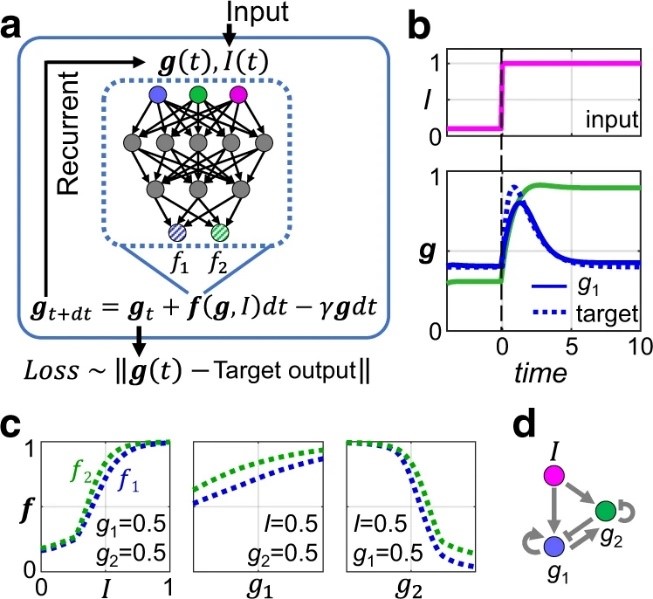
往期精彩推荐
前沿论文带您解读5G应用领域 ——图书馆前沿文献专题推荐服务(2)
热点论文解读AI应用领域 ——图书馆前沿文献专题推荐服务(3)
热点论文带您探究5G和未来通信——图书馆前沿文献专题推荐服务 (4)
前沿文献带您解读自然语言处理技术 ——图书馆前沿文献专题推荐服务(5)
热点论文带您探究5G和未来通信材料技术领域 ——图书馆前沿文献专题推荐服务(6)
热点论文解读AI应用领域 ——图书馆前沿文献专题推荐服务(3)
热点论文带您探究5G和未来通信——图书馆前沿文献专题推荐服务 (4)
前沿文献带您解读自然语言处理技术 ——图书馆前沿文献专题推荐服务(5)
热点论文带您探究5G和未来通信材料技术领域 ——图书馆前沿文献专题推荐服务(6)
热点文献带您关注AI情感分类技术 ——图书馆前沿文献专题推荐服务(7)
热点论文带您探究6G的无限可能——图书馆前沿文献专题推荐服务(8)
热点文献带您关注AI文本摘要自动生成 ——图书馆前沿文献专题推荐服务(9)
热点论文:5G/6G引领社会新进步——图书馆前沿文献专题推荐服务(10)
热点文献带您关注AI机器翻译 ——图书馆前沿文献专题推荐服务(11)
热点论文与您探讨5G/6G网络技术新进展——图书馆前沿文献专题推荐服务(12)
热点文献带您关注AI计算机视觉 ——图书馆前沿文献专题推荐服务(13)
热点论文与带您领略5G/6G的硬科技与新思路 ——图书馆前沿文献专题推荐服务(14)
热点文献带您关注AI计算机视觉 ——图书馆前沿文献专题推荐服务(15)
热点论文带您领略5G/6G的最新技术动向 ——图书馆前沿文献专题推荐服务(18)
热点文献带您关注图神经网络——图书馆前沿文献专题推荐服务(19)
热点论文与带您领略5G/6G材料技术的最新发展——图书馆前沿文献专题推荐服务(20)
热点文献带您关注模式识别——图书馆前沿文献专题推荐服务(21)
热点论文与带您领略6G网络技术的最新发展趋势 ——图书馆前沿文献专题推荐服务(22)
热点文献带您关注机器学习与量子物理 ——图书馆前沿文献专题推荐服务(23)
热点论文与带您领略5G/6G通信器件材料的最新进展 ——图书馆前沿文献专题推荐服务(24)
热点文献带您关注AI自动驾驶——图书馆前沿文献专题推荐服务(25)
热点论文与带您领略5G/6G网络安全和技术的最新进展——图书馆前沿文献专题推荐服务(26)
热点文献带您关注AI神经网络与忆阻器——图书馆前沿文献专题推荐服务(27)
热点论文与带您领略5G/6G电子器件和太赫兹方面的最新进展——图书馆前沿文献专题推荐服务(28)
热点文献带您关注AI与机器人——图书馆前沿文献专题推荐服务(29)
热点论文与带您领略5G/6G热点技术的最新进展——图书馆前沿文献专题推荐服务(30)
热点文献带您关注AI与触觉传感技术——图书馆前沿文献专题推荐服务(31)
热点论文与带您领略5G/6G热点技术的最新进展——图书馆前沿文献专题推荐服务(32)
热点文献带您关注AI深度学习与计算机视觉——图书馆前沿文献专题推荐服务(33)
热点论文与带您领略未来通信的热点技术及最新进展——图书馆前沿文献专题推荐服务(34)
热点文献带您关注AI强化学习——图书馆前沿文献专题推荐服务(35)
热点论文与带您领略5G/6G基础研究的最新进展——图书馆前沿文献专题推荐服务(36)
热点文献带您关注AI与边缘计算——图书馆前沿文献专题推荐服务(37)
热点论文与带您领略5G/6G领域热点研究的最新进展——图书馆前沿文献专题推荐服务(38)
热点文献带您关注AI技术的最新进展——图书馆前沿文献专题推荐服务(39)
热点论文与带您领略5G相关领域研究的最新进展——图书馆前沿文献专题推荐服务(40)
热点文献带您关注AI视觉跟踪——图书馆前沿文献专题推荐服务(41)
热点论文带您领略未来通信在海空领域研究的最新进展——图书馆前沿文献专题推荐服务(42)
热点文献带您关注AI与医学研究——图书馆前沿文献专题推荐服务(43)
热点论文带您领略未来通信在材料领域研究的最新进展——图书馆前沿文献专题推荐服务(44)
热点文献带您关注AI与医学图像——图书馆前沿文献专题推荐服务(45)
热点论文带您领略未来通信在光电材料及信息编码领域的最新进展——图书馆前沿文献专题推荐服务(46)