热点文献带您关注AI视觉跟踪——图书馆前沿文献专题推荐服务(41)
2021-05-31
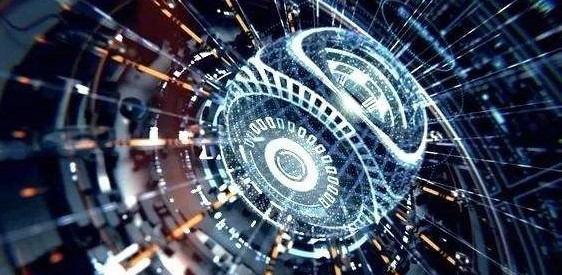
在上一期AI文献推荐中,我们为您推荐了人工智能与三维全息成像技术与重建、运动识别等方面的热点论文,包括利用三维卷积神经网络评估逐搏心脏功能与在血管造影及分割与重建中的应用、基于卷积神经网络的实时三维全息图合成、利用深度学习方法进行3D行为识别等方面的文献。
本期我们为您选取了4篇文献,介绍基于连续深度Q学习的动作预测网络对给定序列的超参数进行自适应优化、基于动态记忆网络适应跟踪过程中目标外观变化的视觉跟踪、基于深度学习的视频显著性预测、基于多层前馈神经网络的石墨烯透明焦堆成像系统三维跟踪等文献,推送给相关领域的科研人员。
文献一 视觉跟踪中基于深度强化学习的动态超参数优化
Dynamical Hyperparameter Optimization via Deep Reinforcement Learning in Tracking
Dong, Xingping, etc.
IEEE TRANSACTIONS ON PATTERN ANALYSIS AND MACHINE INTELLIGENCE, 2021, 43(5): 1515-1529
Hyperparameters are numerical pre-sets whose values are assigned prior to the commencement of a learning process. Selecting appropriate hyperparameters is often critical for achieving satisfactory performance in many vision problems, such as deep learning-based visual object tracking. However, it is often difficult to determine their optimal values, especially if they are specific to each video input. Most hyperparameter optimization algorithms tend to search a generic range and are imposed blindly on all sequences. In this paper, we propose a novel dynamical hyperparameter optimization method that adaptively optimizes hyperparameters for a given sequence using an action-prediction network leveraged on continuous deep Q-learning. Since the observation space for object tracking is significantly more complex than those in traditional control problems, existing continuous deep Q-learning algorithms cannot be directly applied. To overcome this challenge, we introduce an efficient heuristic strategy to handle high dimensional state space, while also accelerating the convergence behavior. The proposed algorithm is applied to improve two representative trackers, a Siamese-based one and a correlation-filter-based one, to evaluate its generalizability. Their superior performances on several popular benchmarks are clearly demonstrated.
阅读原文 https://ieeexplore.ieee.org/document/8918068
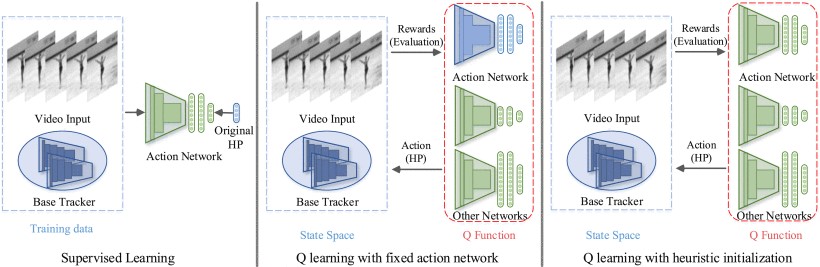
The training process for handling the task of dynamical hyperparameter optimization
文献二 基于动态记忆网络的视觉跟踪Visual Tracking via Dynamic Memory Networks
Yang, Tianyu, etc.
IEEE TRANSACTIONS ON PATTERN ANALYSIS AND MACHINE INTELLIGENCE, 2021, 43(1): 360-374
Template-matching methods for visual tracking have gained popularity recently due to their good performance and fast speed. However, they lack effective ways to adapt to changes in the target object's appearance, making their tracking accuracy still far from state-of-the-art. In this paper, we propose a dynamic memory network to adapt the template to the target's appearance variations during tracking. The reading and writing process of the external memory is controlled by an LSTM network with the search feature map as input. A spatial attention mechanism is applied to concentrate the LSTM input on the potential target as the location of the target is at first unknown. To prevent aggressive model adaptivity, we apply gated residual template learning to control the amount of retrieved memory that is used to combine with the initial template. In order to alleviate the drift problem, we also design a "negative" memory unit that stores templates for distractors, which are used to cancel out wrong responses from the object template. To further boost the tracking performance, an auxiliary classification loss is added after the feature extractor part. Unlike tracking-by-detection methods where the object's information is maintained by the weight parameters of neural networks, which requires expensive online fine-tuning to be adaptable, our tracker runs completely feed-forward and adapts to the target's appearance changes by updating the external memory. Moreover, the capacity of our model is not determined by the network size as with other trackers - the capacity can be easily enlarged as the memory requirements of a task increase, which is favorable for memorizing long-term object information. Extensive experiments on the OTB and VOT datasets demonstrate that our trackers perform favorably against state-of-the-art tracking methods while retaining real-time speed.
阅读原文 https://ieeexplore.ieee.org/document/8770289
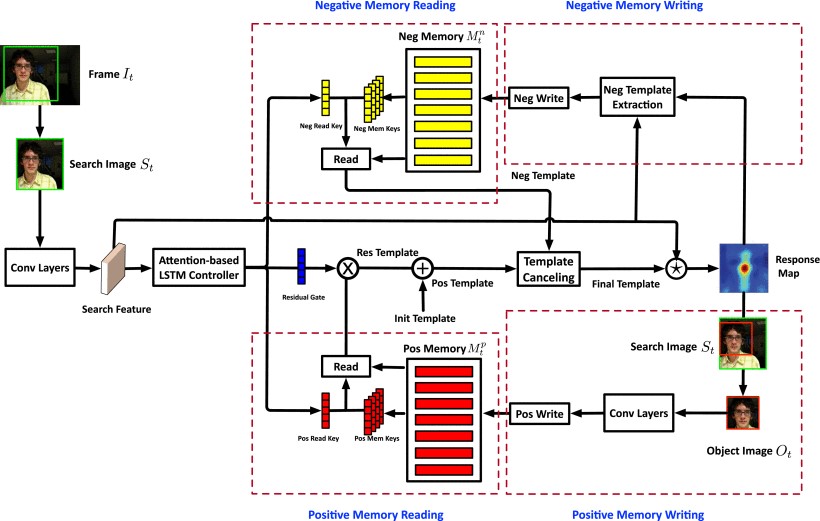
The pipeline of the tracking algorithm
文献三 基于深度学习的视频显著性预测
Revisiting Video Saliency Prediction in the Deep Learning Era
Wang, Wenguan, etc.
IEEE TRANSACTIONS ON PATTERN ANALYSIS AND MACHINE INTELLIGENCE, 2021, 43(1): 220-237
Predicting where people look in static scenes, a.k.a visual saliency, has received significant research interest recently. However, relatively less effort has been spent in understanding and modeling visual attention over dynamic scenes. This work makes three contributions to video saliency research. First, we introduce a new benchmark, called DHF1K (Dynamic Human Fixation 1K), for predicting fixations during dynamic scene free-viewing, which is a long-time need in this field. DHF1K consists of 1K high-quality elaborately-selected video sequences annotated by 17 observers using an eye tracker device. The videos span a wide range of scenes, motions, object types and backgrounds. Second, we propose a novel video saliency model, called ACLNet (Attentive CNN-LSTM Network), that augments the CNN-LSTM architecture with a supervised attention mechanism to enable fast end-to-end saliency learning. The attention mechanism explicitly encodes static saliency information, thus allowing LSTM to focus on learning a more flexible temporal saliency representation across successive frames. Such a design fully leverages existing large-scale static fixation datasets, avoids overfitting, and significantly improves training efficiency and testing performance. Third, we perform an extensive evaluation of the state-of-the-art saliency models on three datasets : DHF1K, Hollywood-2, and UCF sports. An attribute-based analysis of previous saliency models and cross-dataset generalization are also presented. Experimental results over more than 1.2K testing videos containing 400K frames demonstrate that ACLNet outperforms other contenders and has a fast processing speed (40 fps using a single GPU). Our code and all the results are available at https://github.com/wenguanwang/DHF1K.
阅读原文 https://ieeexplore.ieee.org/document/8744328
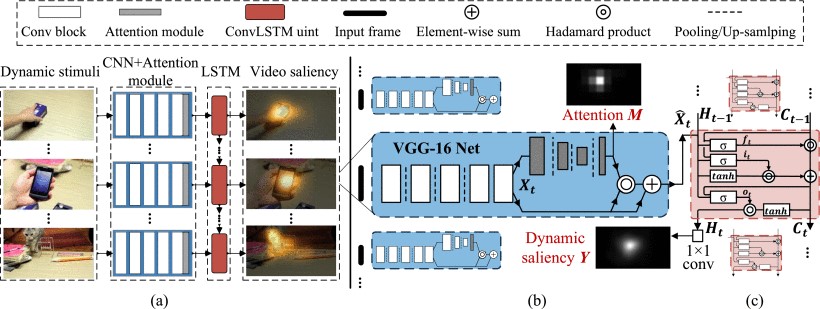
Network architecture of the proposed video saliency model ACLNet
文献四 基于神经网络的石墨烯透明焦堆成像系统三维跟踪
Neural network based 3D tracking with a graphene transparent focal stack imaging system
Dehui Zhang, etc.
NATURE COMMUNICATIONS, 2021, 12
Recent years have seen the rapid growth of new approaches to optical imaging, with an emphasis on extracting three-dimensional (3D) information from what is normally a two-dimensional (2D) image capture. Perhaps most importantly, the rise of computational imaging enables both new physical layouts of optical components and new algorithms to be implemented. This paper concerns the convergence of two advances: the development of a transparent focal stack imaging system using graphene photodetector arrays, and the rapid expansion of the capabilities of machine learning including the development of powerful neural networks. This paper demonstrates 3D tracking of point-like objects with multilayer feedforward neural networks and the extension to tracking positions of multi-point objects. Computer simulations further demonstrate how this optical system can track extended objects in 3D, highlighting the promise of combining nanophotonic devices, new optical system designs, and machine learning for new frontiers in 3D imaging.
阅读原文 https://www.nature.com/articles/s41467-021-22696-x
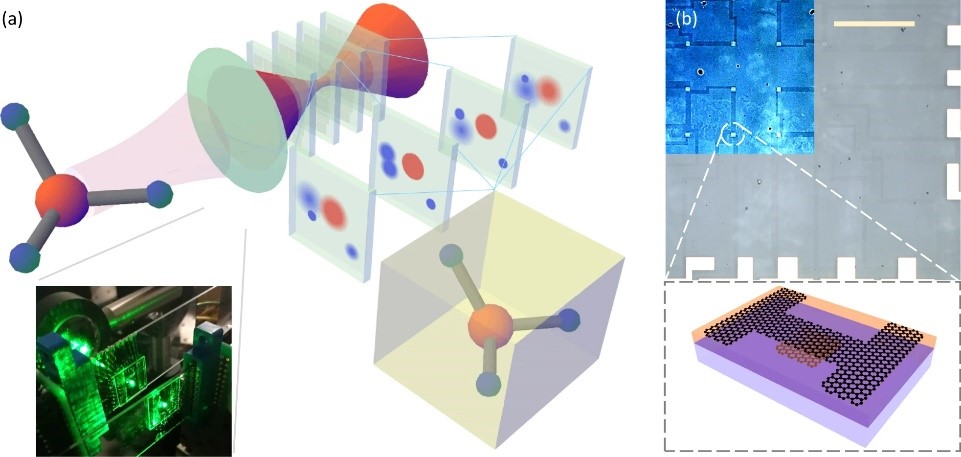
Concept of focal stack imaging system enabled by focal stacks of transparent all-graphene photodetector arrays
往期精彩推荐
前沿论文带您解读5G应用领域 ——图书馆前沿文献专题推荐服务(2)
热点论文解读AI应用领域 ——图书馆前沿文献专题推荐服务(3)
热点论文带您探究5G和未来通信——图书馆前沿文献专题推荐服务 (4)
前沿文献带您解读自然语言处理技术 ——图书馆前沿文献专题推荐服务(5)
热点论文带您探究5G和未来通信材料技术领域 ——图书馆前沿文献专题推荐服务(6)
热点论文解读AI应用领域 ——图书馆前沿文献专题推荐服务(3)
热点论文带您探究5G和未来通信——图书馆前沿文献专题推荐服务 (4)
前沿文献带您解读自然语言处理技术 ——图书馆前沿文献专题推荐服务(5)
热点论文带您探究5G和未来通信材料技术领域 ——图书馆前沿文献专题推荐服务(6)
热点文献带您关注AI情感分类技术 ——图书馆前沿文献专题推荐服务(7)
热点论文带您探究6G的无限可能——图书馆前沿文献专题推荐服务(8)
热点文献带您关注AI文本摘要自动生成 ——图书馆前沿文献专题推荐服务(9)
热点论文:5G/6G引领社会新进步——图书馆前沿文献专题推荐服务(10)
热点文献带您关注AI机器翻译 ——图书馆前沿文献专题推荐服务(11)
热点论文与您探讨5G/6G网络技术新进展——图书馆前沿文献专题推荐服务(12)
热点文献带您关注AI计算机视觉 ——图书馆前沿文献专题推荐服务(13)
热点论文与带您领略5G/6G的硬科技与新思路 ——图书馆前沿文献专题推荐服务(14)
热点文献带您关注AI计算机视觉 ——图书馆前沿文献专题推荐服务(15)
热点论文带您领略5G/6G的最新技术动向 ——图书馆前沿文献专题推荐服务(18)
热点文献带您关注图神经网络——图书馆前沿文献专题推荐服务(19)
热点论文与带您领略5G/6G材料技术的最新发展——图书馆前沿文献专题推荐服务(20)
热点文献带您关注模式识别——图书馆前沿文献专题推荐服务(21)
热点论文与带您领略6G网络技术的最新发展趋势 ——图书馆前沿文献专题推荐服务(22)
热点文献带您关注机器学习与量子物理 ——图书馆前沿文献专题推荐服务(23)
热点论文与带您领略5G/6G通信器件材料的最新进展 ——图书馆前沿文献专题推荐服务(24)
热点文献带您关注AI自动驾驶——图书馆前沿文献专题推荐服务(25)
热点论文与带您领略5G/6G网络安全和技术的最新进展——图书馆前沿文献专题推荐服务(26)
热点文献带您关注AI神经网络与忆阻器——图书馆前沿文献专题推荐服务(27)
热点论文与带您领略5G/6G电子器件和太赫兹方面的最新进展——图书馆前沿文献专题推荐服务(28)
热点文献带您关注AI与机器人——图书馆前沿文献专题推荐服务(29)
热点论文与带您领略5G/6G热点技术的最新进展——图书馆前沿文献专题推荐服务(30)
热点文献带您关注AI与触觉传感技术——图书馆前沿文献专题推荐服务(31)
热点论文与带您领略5G/6G热点技术的最新进展——图书馆前沿文献专题推荐服务(32)
热点文献带您关注AI深度学习与计算机视觉——图书馆前沿文献专题推荐服务(33)
热点论文与带您领略未来通信的热点技术及最新进展——图书馆前沿文献专题推荐服务(34)
热点文献带您关注AI强化学习——图书馆前沿文献专题推荐服务(35)
热点论文与带您领略5G/6G基础研究的最新进展——图书馆前沿文献专题推荐服务(36)
热点文献带您关注AI与边缘计算——图书馆前沿文献专题推荐服务(37)
热点论文与带您领略5G/6G领域热点研究的最新进展——图书馆前沿文献专题推荐服务(38)
热点文献带您关注AI技术的最新进展——图书馆前沿文献专题推荐服务(39)
热点论文与带您领略5G相关领域研究的最新进展——图书馆前沿文献专题推荐服务(40)