热点文献带您关注AI强化学习——图书馆前沿文献专题推荐服务(35)
2021-03-23
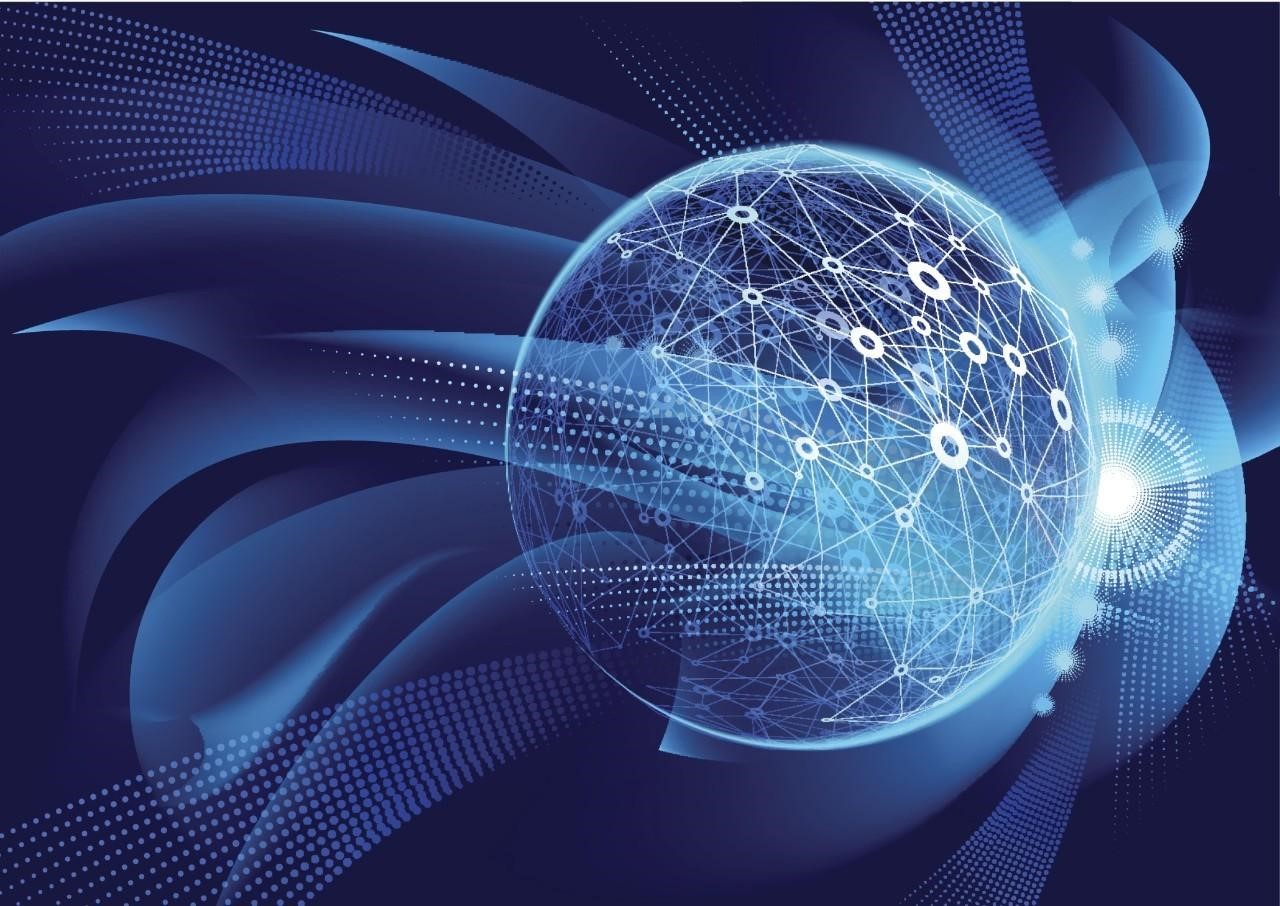
在上一期AI文献推荐中,我们为您推荐了深度学习与计算机视觉的热点论文,包括在三维矢量全息技术、图像检测与去噪、图像压缩传感深度网络体系结构、高精度单级目标检测器等方面的文献。
本期我们为您选取了4篇文献,介绍强化学习的最新动态,包括一种将学习模型纳入训练过程的基于AlphaZero搜索与策略迭代算法的强化学习,通过量子通信信道加速强化学习,基于强化学习的自主机器人纳米加工,基于自适应深度强化学习框架的冰壶机器人等文献,推送给相关领域的科研人员。
文献一 基于模型的强化学习
Mastering Atari, Go, chess and shogi by planning with a learned model
Schrittwieser, Julian, etc.
NATURE, 2020, 588(7839): 604-609
Constructing agents with planning capabilities has long been one of the main challenges in the pursuit of artificial intelligence. Tree-based planning methods have enjoyed huge success in challenging domains, such as chess and Go, where a perfect simulator is available. However, in real-world problems, the dynamics governing the environment are often complex and unknown. Here we present the MuZero algorithm, which, by combining a tree-based search with a learned model, achieves superhuman performance in a range of challenging and visually complex domains, without any knowledge of their underlying dynamics. The MuZero algorithm learns an iterable model that produces predictions relevant to planning: the action-selection policy, the value function and the reward. When evaluated on 57 different Atari games-the canonical video game environment for testing artificial intelligence techniques, in which model-based planning approaches have historically struggled-the MuZero algorithm achieved state-of-the-art performance. When evaluated on Go, chess and shogi-canonical environments for high-performance planning-the MuZero algorithm matched, without any knowledge of the game dynamics, the superhuman performance of the AlphaZero algorithm that was supplied with the rules of the game.
阅读原文 https://www.nature.com/articles/s41586-020-03051-4
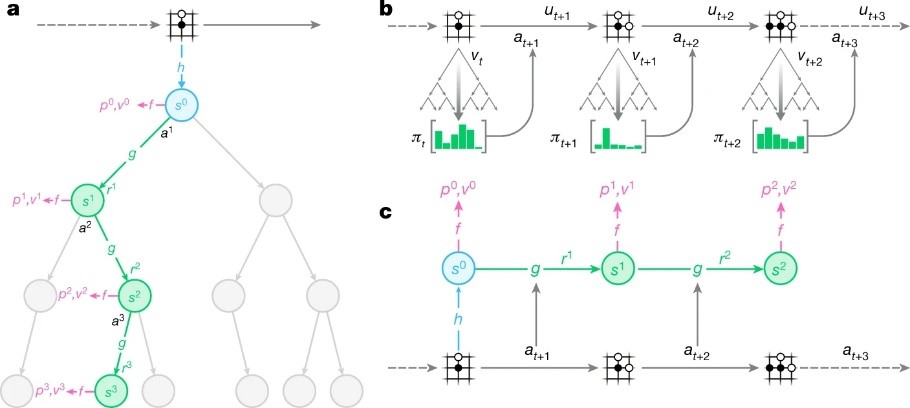
How MuZero algorithm uses its model to plan, act and train
文献二 通过量子通信信道加速强化学习
Experimental quantum speed-up in reinforcement learning agents
V. Saggio, etc.
NATURE, 2021, 591: 229–233
As the field of artificial intelligence advances, the demand for algorithms that can learn quickly and efficiently increases. An important paradigm within artificial intelligence is reinforcement learning, where decision-making entities called agents interact with environments and learn by updating their behaviour on the basis of the obtained feedback. The crucial question for practical applications is how fast agents learn. Although various studies have made use of quantum mechanics to speed up the agent’s decision-making process, a reduction in learning time has not yet been demonstrated. Here we present a reinforcement learning experiment in which the learning process of an agent is sped up by using a quantum communication channel with the environment. We further show that combining this scenario with classical communication enables the evaluation of this improvement and allows optimal control of the learning progress. We implement this learning protocol on a compact and fully tunable integrated nanophotonic processor. The device interfaces with telecommunication-wavelength photons and features a fast active-feedback mechanism, demonstrating the agent’s systematic quantum advantage in a setup that could readily be integrated within future large-scale quantum communication networks.
阅读原文 https://www.nature.com/articles/s41586-021-03242-7
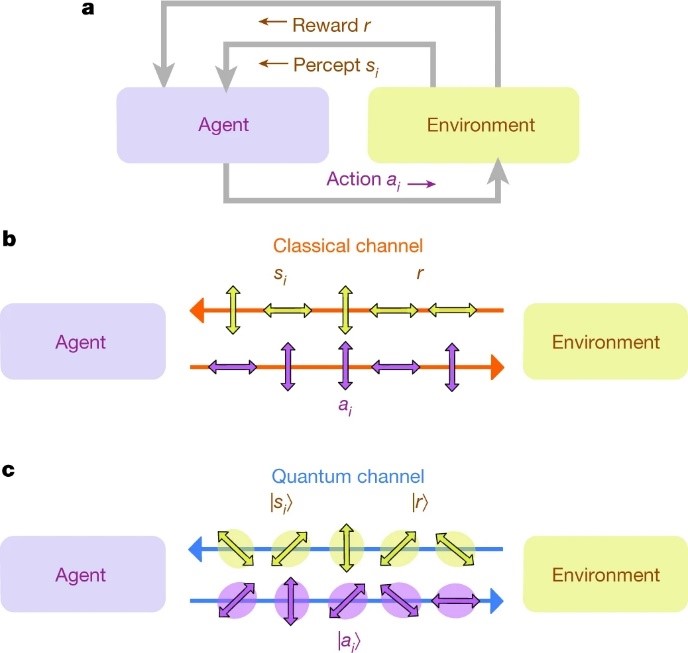
Schematic of the learning agent
文献三 基于强化学习的自主机器人纳米加工
Autonomous robotic nanofabrication with reinforcement learning
Leinen, Philipp, etc.
SCIENCE ADVANCES, 2020, 6(36)
The ability to handle single molecules as effectively as macroscopic building blocks would enable the construction of complex supramolecular structures inaccessible to self-assembly. The fundamental challenges obstructing this goal are the uncontrolled variability and poor observability of atomic- scale conformations. Here, we present a strategy to work around both obstacles and demonstrate autonomous robotic nanofabrication by manipulating single molecules. Our approach uses reinforcement learning (RL), which finds solution strategies even in the face of large uncertainty and sparse feedback. We demonstrate the potential of our RL approach by removing molecules autonomously with a scanning probe microscope from a supramolecular structure. Our RL agent reaches an excellent performance, enabling us to automate a task that previously had to be performed by a human. We anticipate that our work opens the way toward autonomous agents for the robotic construction of functional supramolecular structures with speed, precision, and perseverance beyond our current capabilities.
阅读原文 https://advances.sciencemag.org/content/6/36/eabb6987
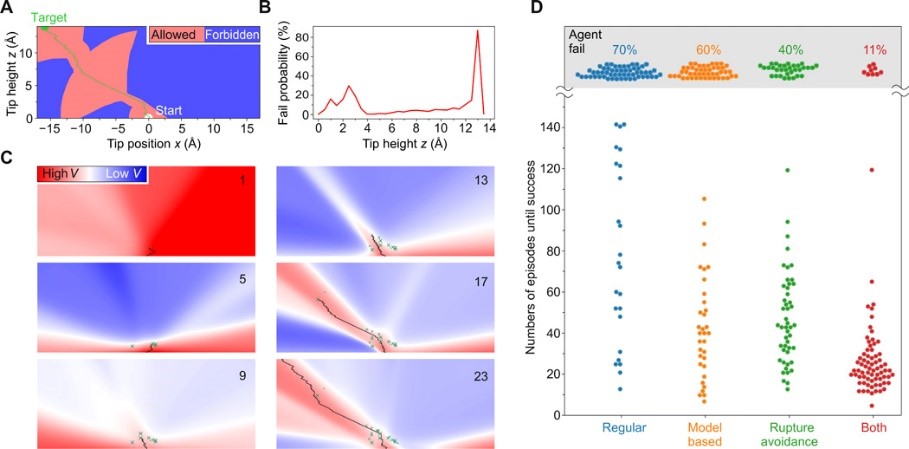
Training and performance of RL agents
文献四 基于自适应深度强化学习框架的冰壶机器人
An adaptive deep reinforcement learning framework enables curling robots with human-like performance in real-world conditions
Won, Dong-Ok, etc.
SCIENCE ROBOTICS, 2020, 5(46)
The game of curling can be considered a good test bed for studying the interaction between artificial intelligence systems and the real world. In curling, the environmental characteristics change at every moment, and every throw has an impact on the outcome of the match. Furthermore, there is no time for relearning during a curling match due to the timing rules of the game. Here, we report a curling robot that can achieve human-level performance in the game of curling using an adaptive deep reinforcement learning framework. Our proposed adaptation framework extends standard deep reinforcement learning using temporal features, which learn to compensate for the uncertainties and nonstationarities that are an unavoidable part of curling. Our curling robot, Curly, was able to win three of four official matches against expert human teams [top-ranked women's curling teams and Korea national wheelchair curling team (reserve team)]. These results indicate that the gap between physics-based simulators and the real world can be narrowed.
阅读原文 https://robotics.sciencemag.org/content/5/46/eabb9764
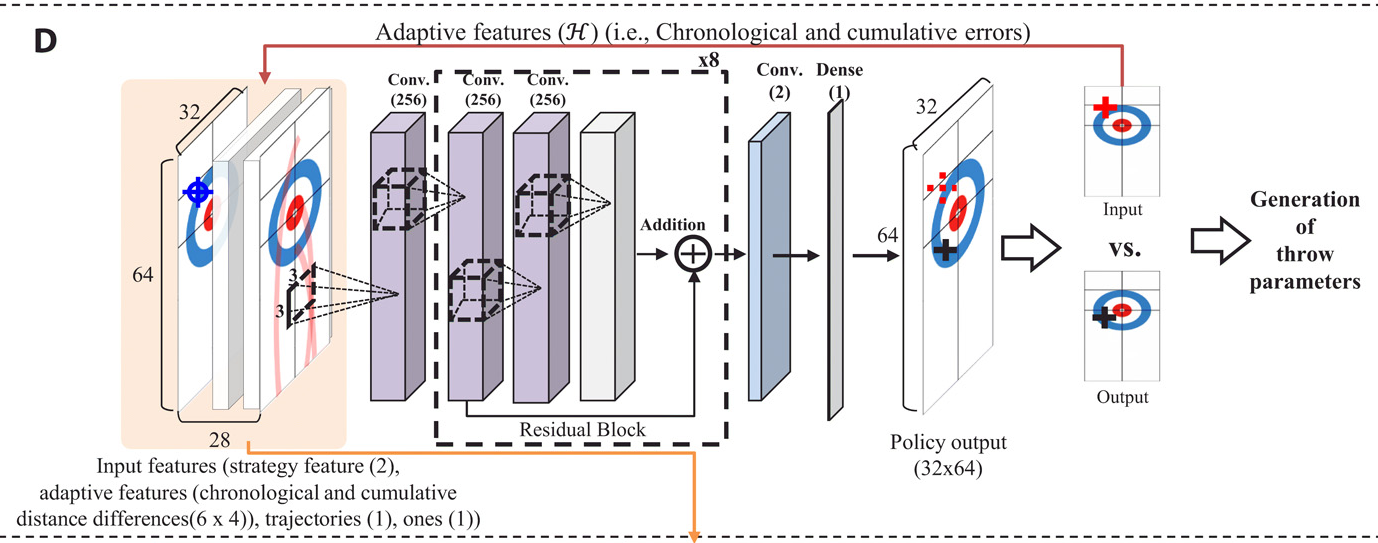
Architecture of the network for adaptative deep RL文献四 基于自适应深度强化学习框架的冰壶机器人
An adaptive deep reinforcement learning framework enables curling robots with human-like performance in real-world conditions
Won, Dong-Ok, etc.
SCIENCE ROBOTICS, 2020, 5(46)
The game of curling can be considered a good test bed for studying the interaction between artificial intelligence systems and the real world. In curling, the environmental characteristics change at every moment, and every throw has an impact on the outcome of the match. Furthermore, there is no time for relearning during a curling match due to the timing rules of the game. Here, we report a curling robot that can achieve human-level performance in the game of curling using an adaptive deep reinforcement learning framework. Our proposed adaptation framework extends standard deep reinforcement learning using temporal features, which learn to compensate for the uncertainties and nonstationarities that are an unavoidable part of curling. Our curling robot, Curly, was able to win three of four official matches against expert human teams [top-ranked women's curling teams and Korea national wheelchair curling team (reserve team)]. These results indicate that the gap between physics-based simulators and the real world can be narrowed.
阅读原文 https://robotics.sciencemag.org/content/5/46/eabb9764
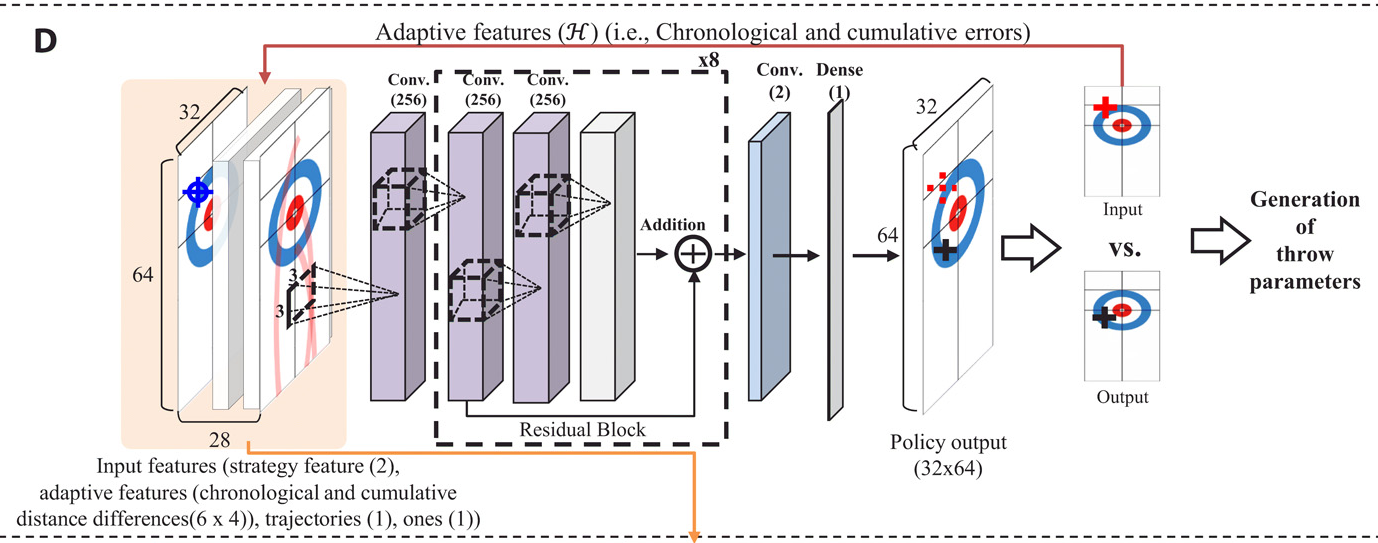
往期精彩推荐
前沿论文带您解读5G应用领域 ——图书馆前沿文献专题推荐服务(2)
热点论文解读AI应用领域 ——图书馆前沿文献专题推荐服务(3)
热点论文带您探究5G和未来通信——图书馆前沿文献专题推荐服务 (4)
前沿文献带您解读自然语言处理技术 ——图书馆前沿文献专题推荐服务(5)
热点论文带您探究5G和未来通信材料技术领域 ——图书馆前沿文献专题推荐服务(6)
热点论文解读AI应用领域 ——图书馆前沿文献专题推荐服务(3)
热点论文带您探究5G和未来通信——图书馆前沿文献专题推荐服务 (4)
前沿文献带您解读自然语言处理技术 ——图书馆前沿文献专题推荐服务(5)
热点论文带您探究5G和未来通信材料技术领域 ——图书馆前沿文献专题推荐服务(6)
热点文献带您关注AI情感分类技术 ——图书馆前沿文献专题推荐服务(7)
热点论文带您探究6G的无限可能——图书馆前沿文献专题推荐服务(8)
热点文献带您关注AI文本摘要自动生成 ——图书馆前沿文献专题推荐服务(9)
热点论文:5G/6G引领社会新进步——图书馆前沿文献专题推荐服务(10)
热点文献带您关注AI机器翻译 ——图书馆前沿文献专题推荐服务(11)
热点论文与您探讨5G/6G网络技术新进展——图书馆前沿文献专题推荐服务(12)
热点文献带您关注AI计算机视觉 ——图书馆前沿文献专题推荐服务(13)
热点论文与带您领略5G/6G的硬科技与新思路 ——图书馆前沿文献专题推荐服务(14)
热点文献带您关注AI计算机视觉 ——图书馆前沿文献专题推荐服务(15)
热点论文带您领略5G/6G的最新技术动向 ——图书馆前沿文献专题推荐服务(18)
热点文献带您关注图神经网络——图书馆前沿文献专题推荐服务(19)
热点论文与带您领略5G/6G材料技术的最新发展——图书馆前沿文献专题推荐服务(20)
热点文献带您关注模式识别——图书馆前沿文献专题推荐服务(21)
热点论文与带您领略6G网络技术的最新发展趋势 ——图书馆前沿文献专题推荐服务(22)
热点文献带您关注机器学习与量子物理 ——图书馆前沿文献专题推荐服务(23)
热点论文与带您领略5G/6G通信器件材料的最新进展 ——图书馆前沿文献专题推荐服务(24)
热点文献带您关注AI自动驾驶——图书馆前沿文献专题推荐服务(25)
热点论文与带您领略5G/6G网络安全和技术的最新进展——图书馆前沿文献专题推荐服务(26)
热点文献带您关注AI神经网络与忆阻器——图书馆前沿文献专题推荐服务(27)
热点论文与带您领略5G/6G电子器件和太赫兹方面的最新进展——图书馆前沿文献专题推荐服务(28)
热点文献带您关注AI与机器人——图书馆前沿文献专题推荐服务(29)
热点论文与带您领略5G/6G热点技术的最新进展——图书馆前沿文献专题推荐服务(30)
热点文献带您关注AI与触觉传感技术——图书馆前沿文献专题推荐服务(31)
热点论文与带您领略5G/6G热点技术的最新进展——图书馆前沿文献专题推荐服务(32)
热点文献带您关注AI深度学习与计算机视觉——图书馆前沿文献专题推荐服务(33)
热点论文与带您领略未来通信的热点技术及最新进展——图书馆前沿文献专题推荐服务(34)