热点文献带您关注AI领域的最新进展——图书馆前沿文献专题推荐服务(61)
2022-03-28
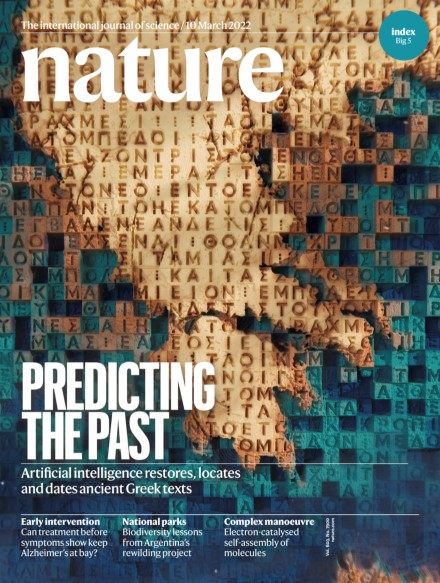
在上一期AI文献推荐中,我们为您推荐了深度学习的热点文献,包括通过深度学习预测未知原发性癌症的起源,基于贝叶斯深度学习的晶体结构识别方法,基于深度学习的步态识别,以及面向手绘素描数据的深度学习技术及其应用。
本期我们为您选取了4篇文献,介绍人工智能应用于其他学科的热点文献。包括基于深度神经网络的古籍恢复,利用人工智能机器学习指导数学直觉,气泡铸造软体机器人,人工智能与量子力学方法在化学中的应用,推送给相关领域的科研人员。
文献一 基于深度神经网络的古籍恢复
Restoring and attributing ancient texts using deep neural networks
Yannis Assael, etc.
NATURE, 2022, 603: 280–283
Ancient history relies on disciplines such as epigraphy—the study of inscribed texts known as inscriptions—for evidence of the thought, language, society and history of past civilizations1. However, over the centuries, many inscriptions have been damaged to the point of illegibility, transported far from their original location and their date of writing is steeped in uncertainty. Here we present Ithaca, a deep neural network for the textual restoration, geographical attribution and chronological attribution of ancient Greek inscriptions. Ithaca is designed to assist and expand the historian’s workflow. The architecture of Ithaca focuses on collaboration, decision support and interpretability. While Ithaca alone achieves 62% accuracy when restoring damaged texts, the use of Ithaca by historians improved their accuracy from 25% to 72%, confirming the synergistic effect of this research tool. Ithaca can attribute inscriptions to their original location with an accuracy of 71% and can date them to less than 30 years of their ground-truth ranges, redating key texts of Classical Athens and contributing to topical debates in ancient history. This research shows how models such as Ithaca can unlock the cooperative potential between artificial intelligence and historians, transformationally impacting the way that we study and write about one of the most important periods in human history.
阅读原文:https://www.nature.com/articles/s41586-022-04448-z
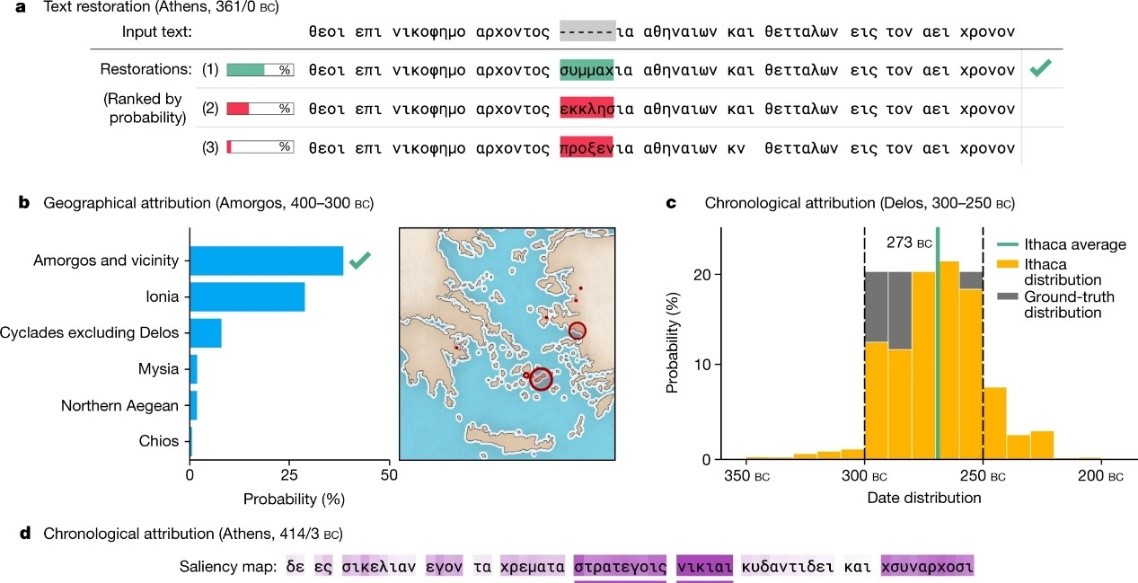
The deep neural network Ithaca’s outputs
文献二 利用人工智能机器学习指导数学直觉
Advancing mathematics by guiding human intuition with AI
Alex Davies, etc.
NATURE, 2021, 600: 70–74
The practice of mathematics involves discovering patterns and using these to formulate and prove conjectures, resulting in theorems. Since the 1960s, mathematicians have used computers to assist in the discovery of patterns and formulation of conjectures1, most famously in the Birch and Swinnerton-Dyer conjecture2, a Millennium Prize Problem3. Here we provide examples of new fundamental results in pure mathematics that have been discovered with the assistance of machine learning—demonstrating a method by which machine learning can aid mathematicians in discovering new conjectures and theorems. We propose a process of using machine learning to discover potential patterns and relations between mathematical objects, understanding them with attribution techniques and using these observations to guide intuition and propose conjectures. We outline this machine-learning-guided framework and demonstrate its successful application to current research questions in distinct areas of pure mathematics, in each case showing how it led to meaningful mathematical contributions on important open problems: a new connection between the algebraic and geometric structure of knots, and a candidate algorithm predicted by the combinatorial invariance conjecture for symmetric groups4. Our work may serve as a model for collaboration between the fields of mathematics and artificial intelligence (AI) that can achieve surprising results by leveraging the respective strengths of mathematicians and machine learning.
阅读原文:https://www.nature.com/articles/s41586-021-04086-x
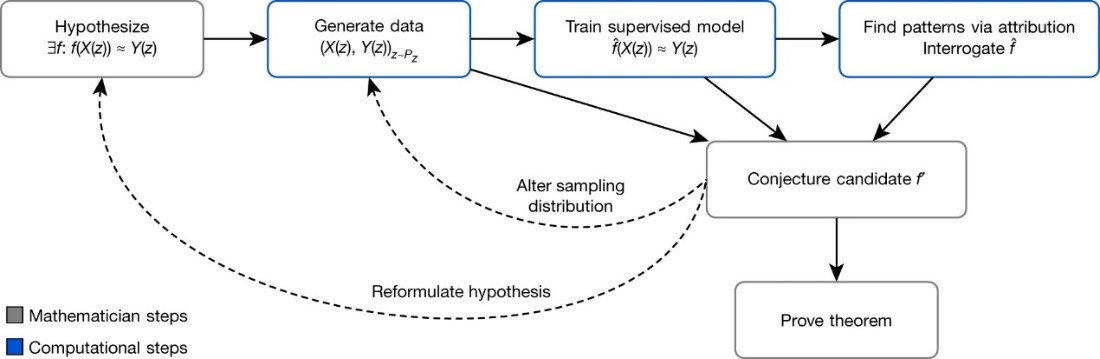
Flowchart of the framework
文献三 气泡铸造软体机器人
Bubble casting soft robotics
Trevor J. Jones, etc.
NATURE, 2021, 599: 229–233
Inspired by living organisms, soft robots are developed from intrinsically compliant materials, enabling continuous motions that mimic animal and vegetal movement1. In soft robots, the canonical hinges and bolts are replaced by elastomers assembled into actuators programmed to change shape following the application of stimuli, for example pneumatic inflation2,3,4,5. The morphing information is typically directly embedded within the shape of these actuators, whose assembly is facilitated by recent advances in rapid prototyping techniques6,7,8,9,10,11. Yet, these manufacturing processes have limitations in scalability, design flexibility and robustness. Here we demonstrate a new all-in-one methodology for the fabrication and the programming of soft machines. Instead of relying on the assembly of individual parts, our approach harnesses interfacial flows in elastomers that progressively cure to robustly produce monolithic pneumatic actuators whose shape can easily be tailored to suit applications ranging from artificial muscles to grippers. We rationalize the fluid mechanics at play in the assembly of our actuators and model their subsequent morphing. We leverage this quantitative knowledge to program these soft machines and produce complex functionalities, for example sequential motion obtained from a monotonic stimulus. We expect that the flexibility, robustness and predictive nature of our methodology will accelerate the proliferation of soft robotics by enabling the assembly of complex actuators, for example long, tortuous or vascular structures, thereby paving the way towards new functionalities stemming from geometric and material nonlinearities.
阅读原文:https://www.nature.com/articles/s41586-021-04029-6
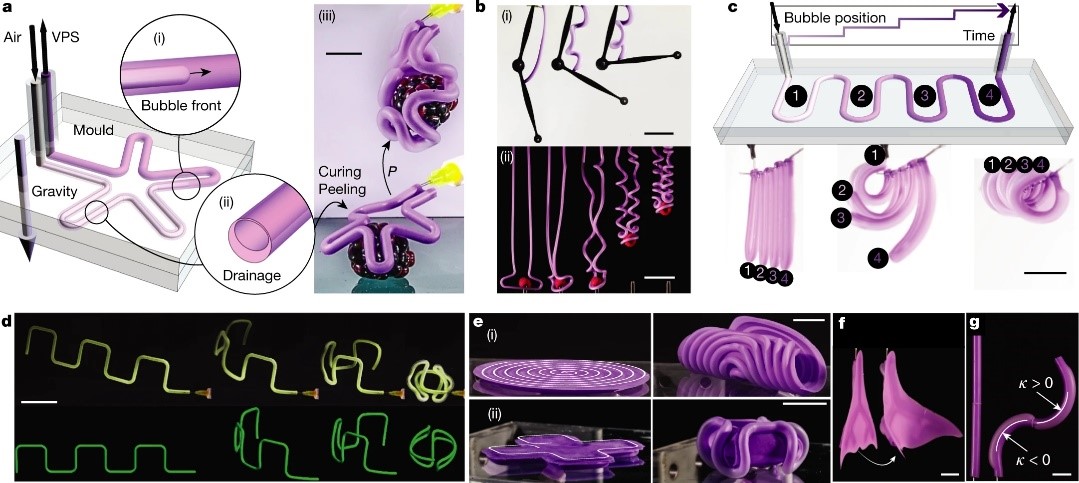
From flow to programmed actuation
文献四 人工智能与量子力学方法在化学中的应用
Artificial intelligence-enhanced quantum chemical method with broad applicability
Peikun Zheng, etc.
NATURE COMMUNICATIONS, 2021, 12: 1–13
High-level quantum mechanical (QM) calculations are indispensable for accurate explanation of natural phenomena on the atomistic level. Their staggering computational cost, however, poses great limitations, which luckily can be lifted to a great extent by exploiting advances in artificial intelligence (AI). Here we introduce the general-purpose, highly transferable artificial intelligence–quantum mechanical method 1 (AIQM1). It approaches the accuracy of the gold-standard coupled cluster QM method with high computational speed of the approximate low-level semiempirical QM methods for the neutral, closed-shell species in the ground state. AIQM1 can provide accurate ground-state energies for diverse organic compounds as well as geometries for even challenging systems such as large conjugated compounds (fullerene C60) close to experiment. This opens an opportunity to investigate chemical compounds with previously unattainable speed and accuracy as we demonstrate by determining geometries of polyyne molecules—the task difficult for both experiment and theory. Noteworthy, our method’s accuracy is also good for ions and excited-state properties, although the neural network part of AIQM1 was never fitted to these properties.
阅读原文:https://www.nature.com/articles/s41467-021-27340-2
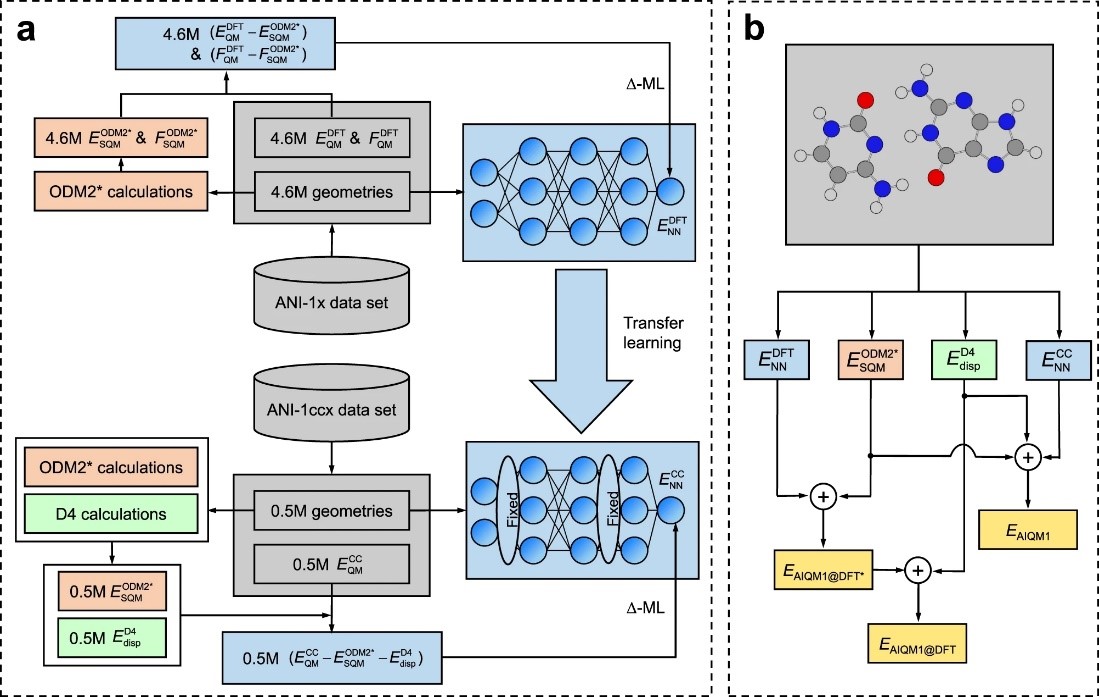
The design of the artificial intelligence–quantum mechanical method 1 (AIQM1)
往期精彩推荐
前沿论文带您解读5G应用领域 ——图书馆前沿文献专题推荐服务(2)
热点论文解读AI应用领域 ——图书馆前沿文献专题推荐服务(3)
热点论文带您探究5G和未来通信——图书馆前沿文献专题推荐服务 (4)
前沿文献带您解读自然语言处理技术 ——图书馆前沿文献专题推荐服务(5)
热点论文带您探究5G和未来通信材料技术领域 ——图书馆前沿文献专题推荐服务(6)
热点论文解读AI应用领域 ——图书馆前沿文献专题推荐服务(3)
热点论文带您探究5G和未来通信——图书馆前沿文献专题推荐服务 (4)
前沿文献带您解读自然语言处理技术 ——图书馆前沿文献专题推荐服务(5)
热点论文带您探究5G和未来通信材料技术领域 ——图书馆前沿文献专题推荐服务(6)
热点文献带您关注AI情感分类技术 ——图书馆前沿文献专题推荐服务(7)
热点论文带您探究6G的无限可能——图书馆前沿文献专题推荐服务(8)
热点文献带您关注AI文本摘要自动生成 ——图书馆前沿文献专题推荐服务(9)
热点论文:5G/6G引领社会新进步——图书馆前沿文献专题推荐服务(10)
热点文献带您关注AI机器翻译 ——图书馆前沿文献专题推荐服务(11)
热点论文与您探讨5G/6G网络技术新进展——图书馆前沿文献专题推荐服务(12)
热点文献带您关注AI计算机视觉 ——图书馆前沿文献专题推荐服务(13)
热点论文与带您领略5G/6G的硬科技与新思路 ——图书馆前沿文献专题推荐服务(14)
热点文献带您关注AI计算机视觉 ——图书馆前沿文献专题推荐服务(15)
热点论文带您领略5G/6G的最新技术动向 ——图书馆前沿文献专题推荐服务(18)
热点文献带您关注图神经网络——图书馆前沿文献专题推荐服务(19)
热点论文与带您领略5G/6G材料技术的最新发展——图书馆前沿文献专题推荐服务(20)
热点文献带您关注模式识别——图书馆前沿文献专题推荐服务(21)
热点论文与带您领略6G网络技术的最新发展趋势 ——图书馆前沿文献专题推荐服务(22)
热点文献带您关注机器学习与量子物理 ——图书馆前沿文献专题推荐服务(23)
热点论文与带您领略5G/6G通信器件材料的最新进展 ——图书馆前沿文献专题推荐服务(24)
热点文献带您关注AI自动驾驶——图书馆前沿文献专题推荐服务(25)
热点论文与带您领略5G/6G网络安全和技术的最新进展——图书馆前沿文献专题推荐服务(26)
热点文献带您关注AI神经网络与忆阻器——图书馆前沿文献专题推荐服务(27)
热点论文与带您领略5G/6G电子器件和太赫兹方面的最新进展——图书馆前沿文献专题推荐服务(28)
热点文献带您关注AI与机器人——图书馆前沿文献专题推荐服务(29)
热点论文与带您领略5G/6G热点技术的最新进展——图书馆前沿文献专题推荐服务(30)
热点文献带您关注AI与触觉传感技术——图书馆前沿文献专题推荐服务(31)
热点论文与带您领略5G/6G热点技术的最新进展——图书馆前沿文献专题推荐服务(32)
热点文献带您关注AI深度学习与计算机视觉——图书馆前沿文献专题推荐服务(33)
热点论文与带您领略未来通信的热点技术及最新进展——图书馆前沿文献专题推荐服务(34)
热点文献带您关注AI强化学习——图书馆前沿文献专题推荐服务(35)
热点论文与带您领略5G/6G基础研究的最新进展——图书馆前沿文献专题推荐服务(36)
热点文献带您关注AI与边缘计算——图书馆前沿文献专题推荐服务(37)
热点论文与带您领略5G/6G领域热点研究的最新进展——图书馆前沿文献专题推荐服务(38)
热点文献带您关注AI技术的最新进展——图书馆前沿文献专题推荐服务(39)
热点论文与带您领略5G相关领域研究的最新进展——图书馆前沿文献专题推荐服务(40)
热点文献带您关注AI视觉跟踪——图书馆前沿文献专题推荐服务(41)
热点论文带您领略未来通信在海空领域研究的最新进展——图书馆前沿文献专题推荐服务(42)
热点文献带您关注AI与医学研究——图书馆前沿文献专题推荐服务(43)
热点论文带您领略未来通信在材料领域研究的最新进展——图书馆前沿文献专题推荐服务(44)
热点文献带您关注AI与医学图像——图书馆前沿文献专题推荐服务(45)
热点论文带您领略未来通信在光电材料及信息编码领域的最新进展——图书馆前沿文献专题推荐服务(46)
热点文献带您关注AI与生物学——图书馆前沿文献专题推荐服务(47)
热点论文带您领略未来通信在新材料技术领域的最新进展——图书馆前沿文献专题推荐服务(48)
热点文献带您关注AI与人脸识别——图书馆前沿文献专题推荐服务(49)
热点论文带您领略光电半导体领域的最新进展——图书馆前沿文献专题推荐服务(50)
热点文献带您关注AI在集成电路领域的最新进展——图书馆前沿文献专题推荐服务(51)
热点论文带您领略半导体领域的最新进展——图书馆前沿文献专题推荐服务(52)
热点文献带您关注AI在光神经网络领域的最新进展——图书馆前沿文献专题推荐服务(53)
热点论文带您领略未来通信在新材料技术领域的最新进展——图书馆前沿文献专题推荐服务(54)
热点文献带您关注AI深度神经网络的最新进展——图书馆前沿文献专题推荐服务(55)
热点论文带您领略新材料半导体领域的最新进展——图书馆前沿文献专题推荐服务(56)
热点文献带您关注AI视频动作识别的最新进展——图书馆前沿文献专题推荐服务(57)
热点论文带您领略未来通信热点技术的最新进展——图书馆前沿文献专题推荐服务(58)
热点文献带您关注AI深度学习的最新进展——图书馆前沿文献专题推荐服务(59)
热点论文带您领略未来通信研究热点的最新进展——图书馆前沿文献专题推荐服务(60)