热点文献带您关注AI深度强化学习的最新进展——图书馆前沿文献专题推荐服务(67)
2022-06-10
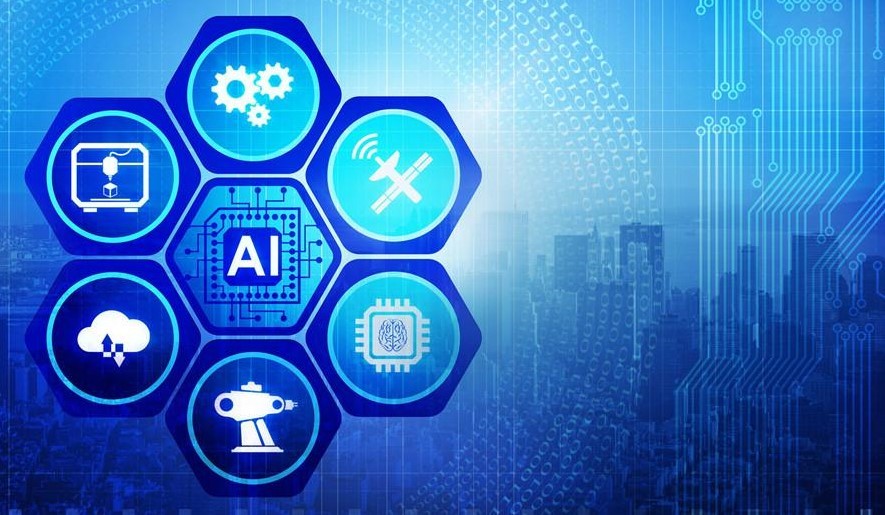
在上一期AI文献推荐中,我们为您推荐了人工智能在传感器设计方面的热点文献,包括利用表面温度的变化调节手指摩擦的方法,利用taxel等值线理论指导超分辨率触觉皮肤的设计,一种拇指大小基于视觉的三维触觉传感器,以及基于主动学习和数据增强的软机器自动应变传感器设计。
本期我们为您选取了4篇文献,介绍来自DeepMind、Sony AI等研究机构与高校在深度强化学习的最新发展前沿,包括通过深度强化学习在赛车游戏中超越人类车手,基于深度强化学习实现托卡马克磁控制器设计,增强、增量和跨语言的社交事件检测体系结构,以及基于深度强化学习的对抗性图像隐写术的热点文献,推送给相关领域的科研人员。
文献一 通过深度强化学习在赛车游戏中超越人类车手
Outracing champion Gran Turismo drivers with deep reinforcement learning
Peter R. Wurman, etc.
NATURE, 2022, 602(7896): 223-228
Many potential applications of artificial intelligence involve making real-time decisions in physical systems while interacting with humans. Automobile racing represents an extreme example of these conditions; drivers must execute complex tactical manoeuvres to pass or block opponents while operating their vehicles at their traction limits1. Racing simulations, such as the PlayStation game Gran Turismo, faithfully reproduce the non-linear control challenges of real race cars while also encapsulating the complex multi-agent interactions. Here we describe how we trained agents for Gran Turismo that can compete with the world’s best e-sports drivers. We combine state-of-the-art, model-free, deep reinforcement learning algorithms with mixed-scenario training to learn an integrated control policy that combines exceptional speed with impressive tactics. In addition, we construct a reward function that enables the agent to be competitive while adhering to racing’s important, but under-specified, sportsmanship rules. We demonstrate the capabilities of our agent, Gran Turismo Sophy, by winning a head-to-head competition against four of the world’s best Gran Turismo drivers. By describing how we trained championship-level racers, we demonstrate the possibilities and challenges of using these techniques to control complex dynamical systems in domains where agents must respect imprecisely defined human norms.
阅读原文:https://www.nature.com/articles/s41586-021-04357-7
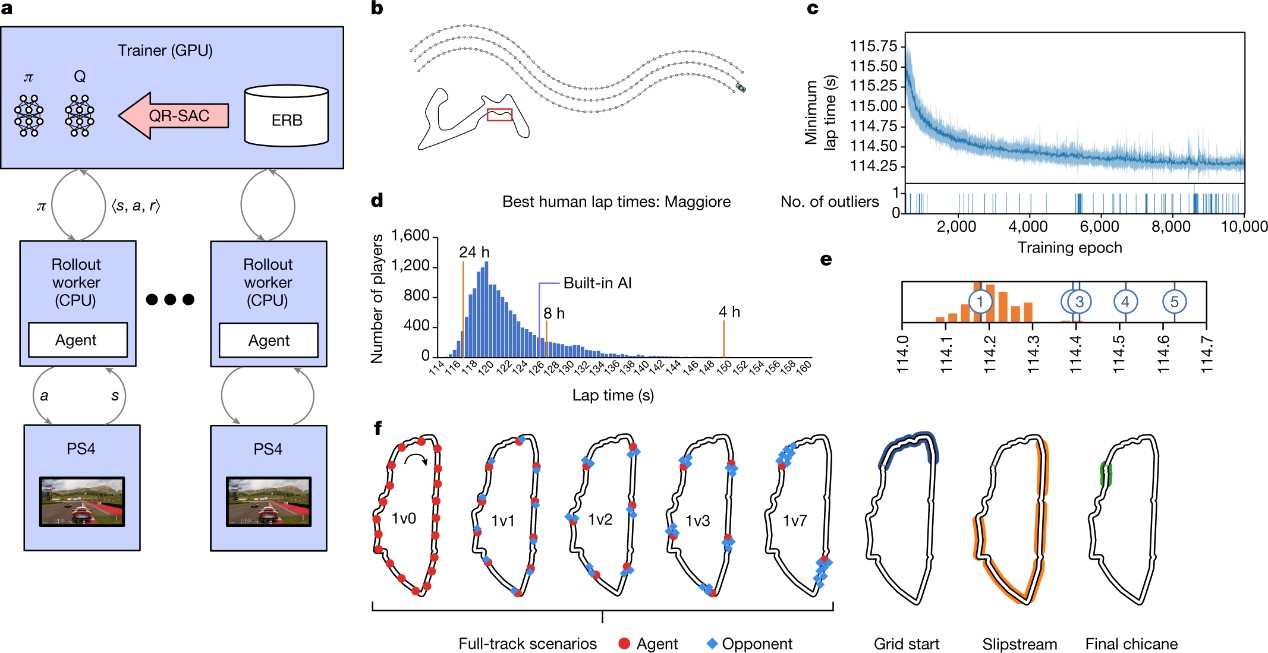
The training configuration and scenarios
文献二 基于深度强化学习实现托卡马克磁控制器设计Magnetic control of tokamak plasmas through deep reinforcement learning
Jonas Degrave, etc.
NATURE, 2022, 602(7897): 414-419
Nuclear fusion using magnetic confinement, in particular in the tokamak configuration, is a promising path towards sustainable energy. A core challenge is to shape and maintain a high-temperature plasma within the tokamak vessel. This requires high-dimensional, high-frequency, closed-loop control using magnetic actuator coils, further complicated by the diverse requirements across a wide range of plasma configurations. In this work, we introduce a previously undescribed architecture for tokamak magnetic controller design that autonomously learns to command the full set of control coils. This architecture meets control objectives specified at a high level, at the same time satisfying physical and operational constraints. This approach has unprecedented flexibility and generality in problem specification and yields a notable reduction in design effort to produce new plasma configurations. We successfully produce and control a diverse set of plasma configurations on the Tokamak à Configuration Variable1,2, including elongated, conventional shapes, as well as advanced configurations, such as negative triangularity and ‘snowflake’ configurations. Our approach achieves accurate tracking of the location, current and shape for these configurations. We also demonstrate sustained ‘droplets’ on TCV, in which two separate plasmas are maintained simultaneously within the vessel. This represents a notable advance for tokamak feedback control, showing the potential of reinforcement learning to accelerate research in the fusion domain, and is one of the most challenging real-world systems to which reinforcement learning has been applied.
阅读原文:https://www.nature.com/articles/s41586-021-04301-9
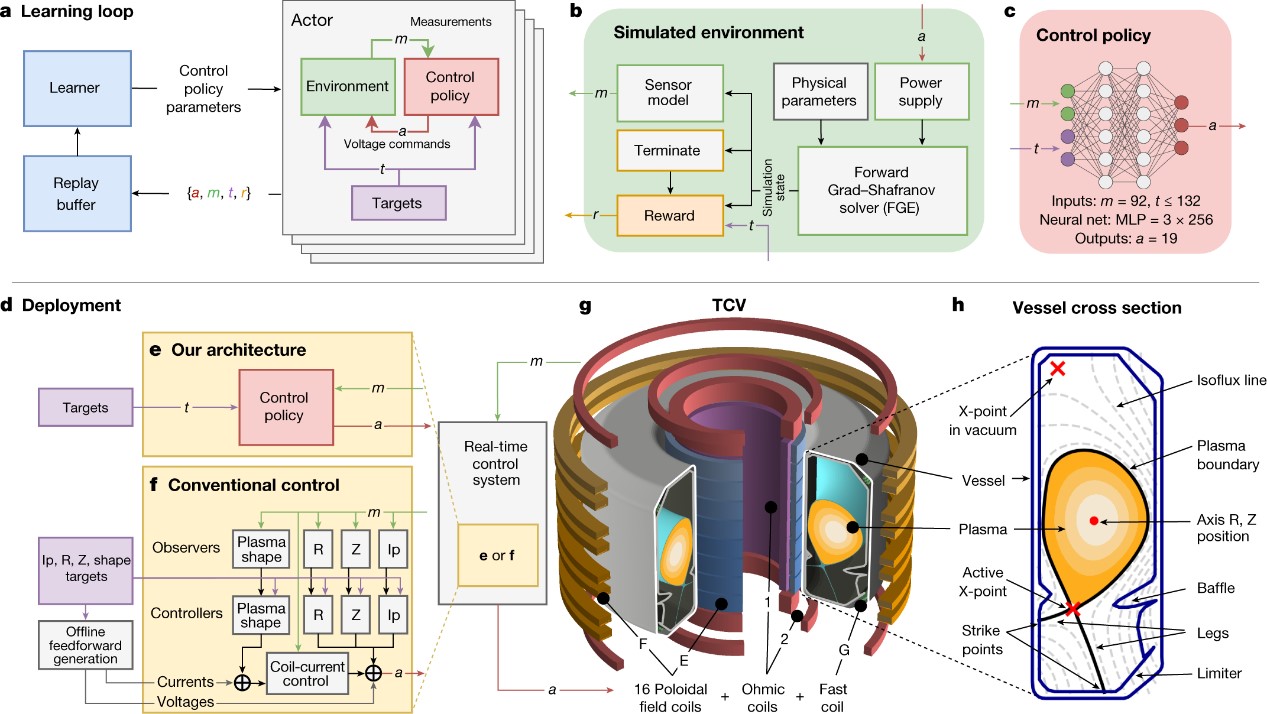
Representation of the components of our controller design architecture
文献三 增强、增量和跨语言的社交事件检测体系结构
Reinforced, Incremental and Cross-lingual Event Detection From Social Messages
Hao Peng, etc.
IEEE TRANSACTIONS ON PATTERN ANALYSIS AND MACHINE INTELLIGENCE, 2022
Detecting hot social events from social messages is crucial as it highlights significant happenings. However, the challenge is that the existing event detection methods are generally confronted with ambiguous events features, dispersive text contents, and multiple languages. In this paper, we present a novel reinForced, incremental and cross-lingual social Event detection architecture, namely FinEvent, from streaming social messages. Concretely, we first model social messages into heterogeneous graphs. Secondly, we propose a new reinforced weighted multi-relational graph neural network framework to select optimal aggregation thresholds to learn social message embeddings. To solve the long-tail problem, a balanced sampling strategy guided Contrastive Learning mechanism is designed for incremental social message representation learning. Thirdly, a new Deep Reinforcement Learning guided density-based spatial clustering model is designed to select the optimal minimum number of samples and optimal minimum distance between two clusters. Finally, we implement incremental social message representation learning based on knowledge preservation on the graph neural network and achieve the transferring cross-lingual social event detection. We conduct extensive experiments to evaluate the FinEvent on Twitter streams, demonstrating a significant and consistent improvement in model quality with 14%-118%, 8%-170%, and 2%-21% increases in performance on offline, online, and cross-lingual social event detection tasks.
阅读原文:https://ieeexplore.ieee.org/document/9693189
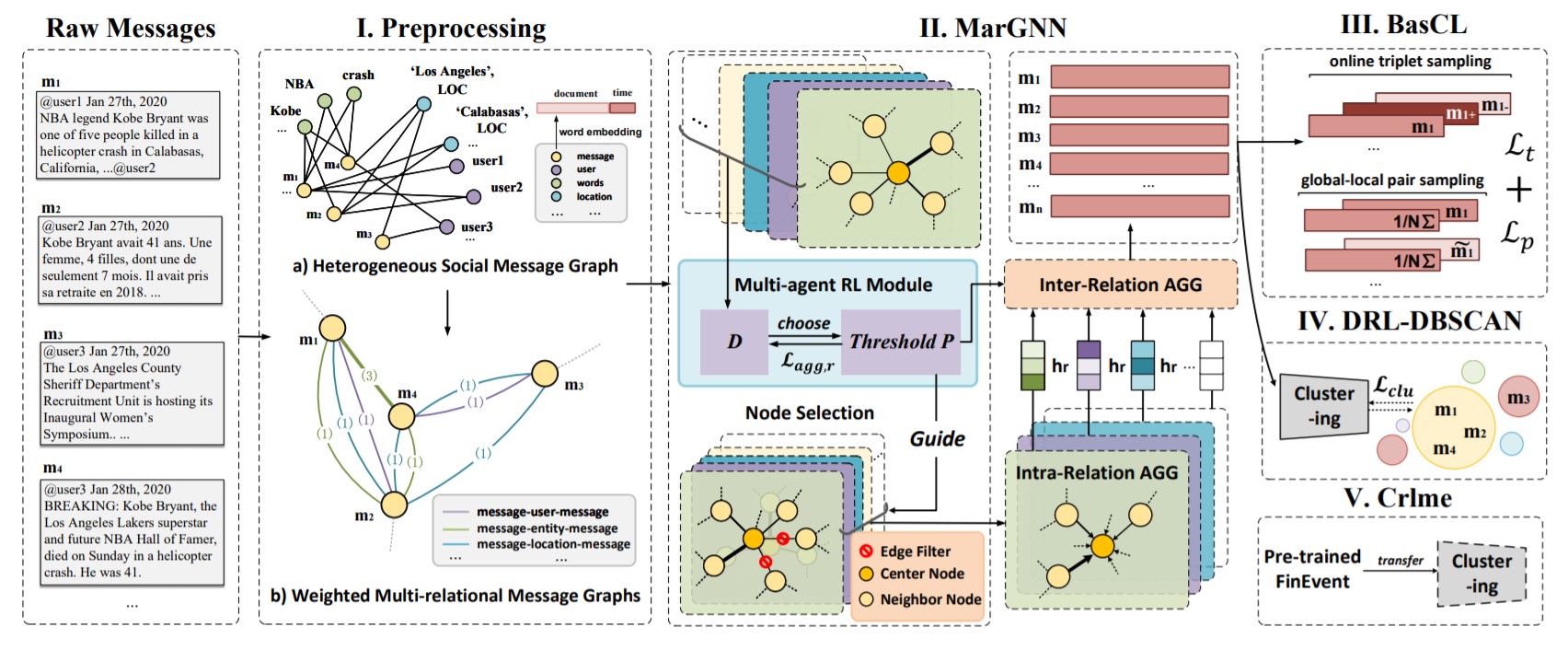
The architecture of the proposed FinEvent
文献四 基于深度强化学习的对抗性图像隐写术
Seek-and-Hide: Adversarial Steganography via Deep Reinforcement Learning
Wenwen Pan, etc.
IEEE TRANSACTIONS ON PATTERN ANALYSIS AND MACHINE INTELLIGENCE, 2021
The goal of image steganography is to hide a full-sized image, termed secret, into another, termed cover. Prior image steganography algorithms can conceal only one secret within one cover. We propose an adaptive local image steganography (AdaSteg) system that allows for scale- and location-adaptive image steganography. By adaptively hiding the secret on a local scale, the proposed system makes the steganography more secured, and further enables multi-secret steganography within one single cover. Specifically, this is achieved via adaptive patch selection stage and secret encryption stage. Given a pair of secret and cover, the optimal local patch for concealment is determined adaptively by exploiting deep reinforcement learning with the proposed steganography quality function and policy network. The secret image is then converted into a patch of encrypted noises, resembling the process of generating adversarial examples, which are further encoded to a local region of the cover to realize a more secured steganography. Furthermore, we propose a novel criterion for the assessment of local steganography and collect a challenging dataset, thus contributing to a standardized benchmark for the area. Experimental results demonstrate that the proposed approach yields results superior to the state of the art in both security and capacity.
阅读原文:https://ieeexplore.ieee.org/document/9546656
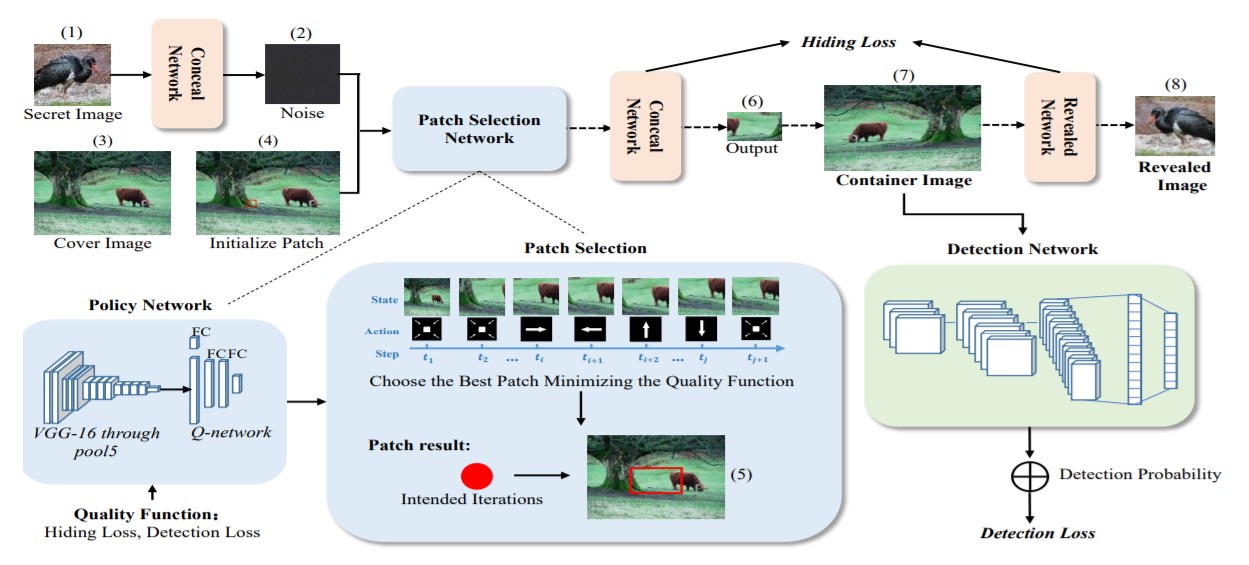
The framework of the proposed AdaSteg system
往期精彩推荐
前沿论文带您解读5G应用领域 ——图书馆前沿文献专题推荐服务(2)
热点论文解读AI应用领域 ——图书馆前沿文献专题推荐服务(3)
热点论文带您探究5G和未来通信——图书馆前沿文献专题推荐服务 (4)
前沿文献带您解读自然语言处理技术 ——图书馆前沿文献专题推荐服务(5)
热点论文带您探究5G和未来通信材料技术领域 ——图书馆前沿文献专题推荐服务(6)
热点论文解读AI应用领域 ——图书馆前沿文献专题推荐服务(3)
热点论文带您探究5G和未来通信——图书馆前沿文献专题推荐服务 (4)
前沿文献带您解读自然语言处理技术 ——图书馆前沿文献专题推荐服务(5)
热点论文带您探究5G和未来通信材料技术领域 ——图书馆前沿文献专题推荐服务(6)
热点文献带您关注AI情感分类技术 ——图书馆前沿文献专题推荐服务(7)
热点论文带您探究6G的无限可能——图书馆前沿文献专题推荐服务(8)
热点文献带您关注AI文本摘要自动生成 ——图书馆前沿文献专题推荐服务(9)
热点论文:5G/6G引领社会新进步——图书馆前沿文献专题推荐服务(10)
热点文献带您关注AI机器翻译 ——图书馆前沿文献专题推荐服务(11)
热点论文与您探讨5G/6G网络技术新进展——图书馆前沿文献专题推荐服务(12)
热点文献带您关注AI计算机视觉 ——图书馆前沿文献专题推荐服务(13)
热点论文与带您领略5G/6G的硬科技与新思路 ——图书馆前沿文献专题推荐服务(14)
热点文献带您关注AI计算机视觉 ——图书馆前沿文献专题推荐服务(15)
热点论文带您领略5G/6G的最新技术动向 ——图书馆前沿文献专题推荐服务(18)
热点文献带您关注图神经网络——图书馆前沿文献专题推荐服务(19)
热点论文与带您领略5G/6G材料技术的最新发展——图书馆前沿文献专题推荐服务(20)
热点文献带您关注模式识别——图书馆前沿文献专题推荐服务(21)
热点论文与带您领略6G网络技术的最新发展趋势 ——图书馆前沿文献专题推荐服务(22)
热点文献带您关注机器学习与量子物理 ——图书馆前沿文献专题推荐服务(23)
热点论文与带您领略5G/6G通信器件材料的最新进展 ——图书馆前沿文献专题推荐服务(24)
热点文献带您关注AI自动驾驶——图书馆前沿文献专题推荐服务(25)
热点论文与带您领略5G/6G网络安全和技术的最新进展——图书馆前沿文献专题推荐服务(26)
热点文献带您关注AI神经网络与忆阻器——图书馆前沿文献专题推荐服务(27)
热点论文与带您领略5G/6G电子器件和太赫兹方面的最新进展——图书馆前沿文献专题推荐服务(28)
热点文献带您关注AI与机器人——图书馆前沿文献专题推荐服务(29)
热点论文与带您领略5G/6G热点技术的最新进展——图书馆前沿文献专题推荐服务(30)
热点文献带您关注AI与触觉传感技术——图书馆前沿文献专题推荐服务(31)
热点论文与带您领略5G/6G热点技术的最新进展——图书馆前沿文献专题推荐服务(32)
热点文献带您关注AI深度学习与计算机视觉——图书馆前沿文献专题推荐服务(33)
热点论文与带您领略未来通信的热点技术及最新进展——图书馆前沿文献专题推荐服务(34)
热点文献带您关注AI强化学习——图书馆前沿文献专题推荐服务(35)
热点论文与带您领略5G/6G基础研究的最新进展——图书馆前沿文献专题推荐服务(36)
热点文献带您关注AI与边缘计算——图书馆前沿文献专题推荐服务(37)
热点论文与带您领略5G/6G领域热点研究的最新进展——图书馆前沿文献专题推荐服务(38)
热点文献带您关注AI技术的最新进展——图书馆前沿文献专题推荐服务(39)
热点论文与带您领略5G相关领域研究的最新进展——图书馆前沿文献专题推荐服务(40)
热点文献带您关注AI视觉跟踪——图书馆前沿文献专题推荐服务(41)
热点论文带您领略未来通信在海空领域研究的最新进展——图书馆前沿文献专题推荐服务(42)
热点文献带您关注AI与医学研究——图书馆前沿文献专题推荐服务(43)
热点论文带您领略未来通信在材料领域研究的最新进展——图书馆前沿文献专题推荐服务(44)
热点文献带您关注AI与医学图像——图书馆前沿文献专题推荐服务(45)
热点论文带您领略未来通信在光电材料及信息编码领域的最新进展——图书馆前沿文献专题推荐服务(46)
热点文献带您关注AI与生物学——图书馆前沿文献专题推荐服务(47)
热点论文带您领略未来通信在新材料技术领域的最新进展——图书馆前沿文献专题推荐服务(48)
热点文献带您关注AI与人脸识别——图书馆前沿文献专题推荐服务(49)
热点论文带您领略光电半导体领域的最新进展——图书馆前沿文献专题推荐服务(50)
热点文献带您关注AI在集成电路领域的最新进展——图书馆前沿文献专题推荐服务(51)
热点论文带您领略半导体领域的最新进展——图书馆前沿文献专题推荐服务(52)
热点文献带您关注AI在光神经网络领域的最新进展——图书馆前沿文献专题推荐服务(53)
热点论文带您领略未来通信在新材料技术领域的最新进展——图书馆前沿文献专题推荐服务(54)
热点文献带您关注AI深度神经网络的最新进展——图书馆前沿文献专题推荐服务(55)
热点论文带您领略新材料半导体领域的最新进展——图书馆前沿文献专题推荐服务(56)
热点文献带您关注AI视频动作识别的最新进展——图书馆前沿文献专题推荐服务(57)
热点论文带您领略未来通信热点技术的最新进展——图书馆前沿文献专题推荐服务(58)
热点文献带您关注AI深度学习的最新进展——图书馆前沿文献专题推荐服务(59)
热点论文带您领略未来通信研究热点的最新进展——图书馆前沿文献专题推荐服务(60)
热点文献带您关注AI领域的最新进展——图书馆前沿文献专题推荐服务(61)
热点论文带您探索智能化以及超表面在未来通信中的应用——图书馆前沿文献专题推荐服务(62)
热点文献带您关注AI领域的最新进展——图书馆前沿文献专题推荐服务(63)
热点论文带您领略半导体领域的最新进展——图书馆前沿文献专题推荐服务(64)
热点文献带您关注AI领域的最新进展——图书馆前沿文献专题推荐服务(65)
热点论文带您探索新型网络架构以及超表面在未来通信中的应用——图书馆前沿文献专题推荐服务(66)